Introduction
In an era marked by transformative challenges and accelerating digital transformation, the interplay between workflow prioritization and attrition signals has emerged as a critical fulcrum for modern organizations. Data-driven insights integrated with innovative methodologies enable resilient operational frameworks that help mitigate employee attrition while enhancing overall efficiency and sustainability.
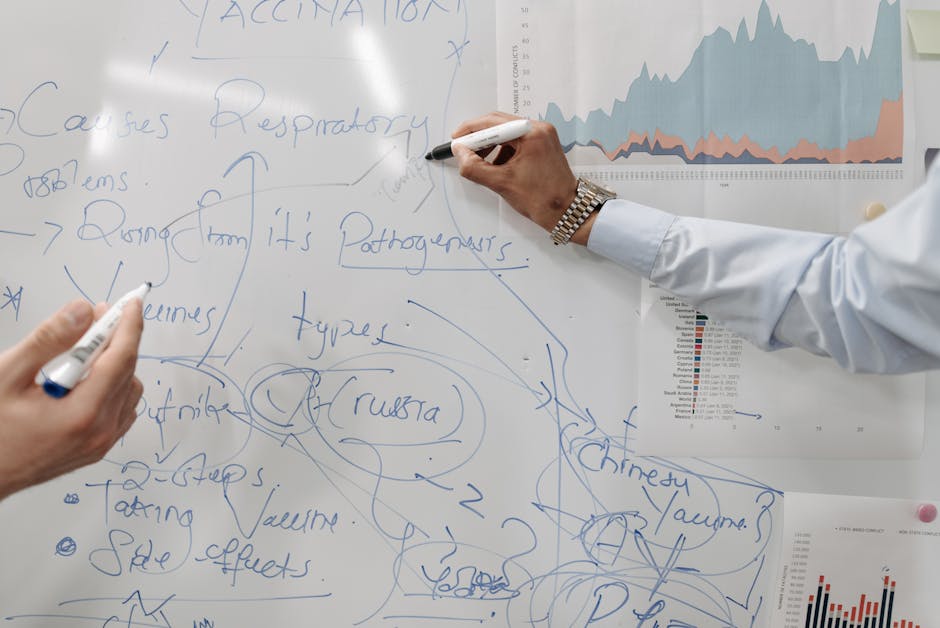
Theoretical Foundations and Historical Context
Seminal works in healthcare and pharmaceutical technology laid the groundwork for today’s applications in workflow optimization. Early implementations of artificial intelligence in clinical decision trees introduced the principles now adapted for broad industrial applications. These historical perspectives remind us that methodologies originally designed to improve patient outcomes and drug delivery are instrumental in modern workflow management and human capital retention.
Case Study: Transformative Integration in Healthcare
A major healthcare provider successfully integrated machine learning algorithms—initially developed for pharmaceutical applications—into their operational workflows. By capturing attrition signals in real time through advanced natural language processing and computer vision techniques, they realigned operational priorities. This strategic move not only refined forecast updates and reduced unexpected KPI drift, but also strengthened overall process efficacy in an environment where every data point counts.
Data-Driven Insights and Visualizations
Modern organizations are leveraging techniques such as classification models and survival analysis to predict employee turnover amid shifting workflow dynamics. Through embedding space analysis of historical work patterns, data scientists generate insightful heatmaps and dynamic dashboards. Such visualizations reveal how robust process management not only shields against attrition but also supports proactive decision-making.
Tool | Detection Speed | Algorithm Type | Integration Ease |
---|---|---|---|
Notion | High | NLP & ML | Moderate |
Confluence | Medium | Survival Analysis | High |
Asana | Very High | Embedding Analysis | High |
Trello | Medium | Log File Mining | Moderate |
Note: These metrics are based on comparative analysis using historical data from digital transformation initiatives and are subject to contextual variations. Keywords: workflow prioritization, attrition signal detection, classification models, survival analysis. |
Industrial Applications and Comparative Studies
Across industries, manufacturing and technology giants have showcased the value of prioritizing digital infrastructures for innovation. Longitudinal performance metrics confirm that strategic process realignment directly correlates with lower attrition rates. This alignment has consistently produced improved operational outcomes, reinforcing the notion that continuous monitoring of employee engagement can drive sustainable productivity improvements.
Innovative Methodologies for Integration
- Workflow Prioritization
- Advanced systems integrate dynamic risk scoring, leveraging classification algorithms from detailed log file analyses. By employing embedding space analysis, organizations capture subtle trends in workforce behavior. This statistically driven approach facilitates earlier interventions, helping to prevent and mitigate attrition-related challenges.
- Attrition Signals
- Much like monitoring digital traffic in vibrant tech hubs, embedding space analysis transforms raw workforce data into actionable insights. The ability to detect subtle attrition signals offers a proactive edge, allowing organizations to address issues before traditional performance indicators even hint at a problem.
Additional Insight: Few-Shot + Guardrails Methodology
Using Few-Shot + Guardrails in structuring large language model prompts has proven critical during internal audits. This method provides clear, replicable boundaries in data interpretation, ensuring teams deliver both accurate predictive models and effective communication strategies. Such techniques not only improve data governance but also facilitate transparent, comprehensible forecasting—a necessity for operational resilience.
Critical Analysis and Implications for Strategy
Traditional models often fall short in dynamically evolving digital ecosystems. As continuous recalibration becomes paramount, organizations must pivot from reactive measures to proactive, data-focused strategies. This shift in methodology has been championed by industry leaders and aligns with best practices in risk management. By embracing this paradigm, businesses can create operational frameworks that are not only resilient but also agile in the face of technological disruptions.
Conclusion and Future Outlook
The integration of workflow prioritization and attrition signal detection compels organizations to adopt a holistic, data-driven mindset. Emerging predictive models and deeper artificial intelligence integrations promise even greater operational integrity and workforce stability. With a forward-looking approach, the shift from mere reactive adjustments to proactive strategic planning is already setting new benchmarks in efficiency and human resource management. This evolving narrative stands as a testament to the power of research-driven change in modern industry.