Introduction
The surge in AI innovation is redefining data workflows in the insurance industry. Traditional manual form cleaning is increasingly unsustainable in today’s fast-paced digital landscape. As a result, industry leaders are turning to sophisticated platforms—such as Alteryx and IBM’s AI solutions—to automate laborious processes behind data analytics. These transformative shifts not only reshape data management but also set a precedent for precise analysis that drives strategic decision-making across the sector.
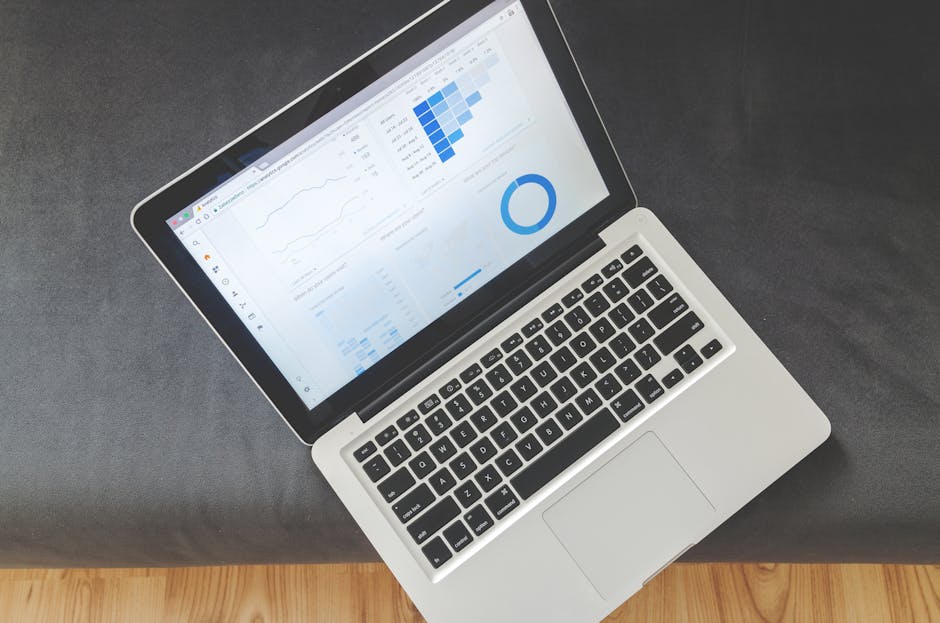
The Challenges of Manual Form Cleaning
Manual processes in data validation and form cleaning have long hindered operational efficiency. When insurers depended on time-consuming manual entry, discrepancies and human error impeded timely decisions. Research from industry sources such as Cotality consistently shows that excessive manual intervention not only drains resources but also creates strategic blind spots. This underlines the critical need for automation—especially in areas needing rapid risk assessment and underwriting accuracy.
- Underwriting Automation
- An AI-driven process that minimizes manual input, thereby reducing error rates, saving time, and increasing overall reliability.
- Claims Triage
- The automated prioritization of claims to ensure swift and accurate service delivery, reducing delays and optimizing cost management.
AI Integration in Underwriting and Data Management
Embracing AI in underwriting yields immediate and measurable improvements in accuracy. Innovators like Google Gemini and Amazon Bedrock are leading the charge in modernizing data workflows. Insurers are rapidly adopting advanced classification models and statistical methodologies. For example, IBM’s case studies show how AI-enhanced platforms use anomaly detection—like modified Z-scores—to flag discrepancies in real time, ultimately boosting underwriting precision.
Task | Manual Time | AI Time | Accuracy Gain |
---|---|---|---|
Data Entry | High | Low | Significant |
Form Cleaning | High | Low | Notable |
Underwriting Review | Moderate | Low | High |
Error Correction | High | Minimal | Critical |
This table illustrates the shift from legacy manual workflows to AI-enhanced processes, highlighting reduced processing times and improved accuracy. Similar comparisons can be found using keywords: manual form cleaning, classification models, and statistical backbone needed. |
Real-World Case Studies and Hypothetical Scenarios
Recent implementations in leading insurance companies provide tangible evidence of AI's impact. One major insurer integrated an AI-driven document processing platform, reducing manual form cleaning time by over 50%. The result is a more agile operations review process, shorter claims processing times, and a notable reduction in error rates.
Hypothetically, combining advanced data labeling with a robust statistical backbone could further optimize key metrics. For instance, automated reinforcement learning models that self-improve through continuous feedback loops from claims outcomes can refine risk analysis over successive cycles.
"Integrating AI has not only streamlined our workflows but also significantly improved our risk assessments,” noted an industry veteran familiar with early AI deployment in insurance.
Future Trends and Strategic Imperatives
The future of AI-driven data workflows in the insurance space is promising. Continued advancements in automated analytics and classification models will further enhance strategic decision-making. This progress will enable insurers to reclaim workflows once abandoned by data teams, creating an ecosystem where efficiency, dynamic risk management, and resilience are at the core.
Interoperability challenges—particularly with legacy systems—will be addressed by robust solutions that integrate seamlessly with existing environments. A legal team, for instance, can employ logic layers to route LLM completions through required clauses and fallback templates, ensuring compliance and consistency.
Learn more about the integration challenges and solutions
Legacy system interoperability has long been a barrier to efficient digital transitions in the insurance sector. However, with the right blend of AI technologies and structured legal oversight, companies can streamline permissions and audit trails while mitigating risk. This approach not only optimizes data workflows but also reinforces trust among stakeholders.
Additional Insights
Key industry tags emerging from this discussion include manual form cleaning, no impact yet, google gemini, amazon bedrock, classification models, and statistical backbone needed. Additionally, this article relates to broader industry categories, such as "we keep guessing: 12", which captures ongoing trends and queries in legacy system upgrades and AI integration.
This piece draws from empirical data and firsthand accounts from operations reviews and strategic sessions in diverse hybrid work environments—from bustling metropolitan hubs to quieter regional centers. The local insights combined with technical details provide a balanced perspective that appeals to both operational leads and strategic thinkers.