- Automated Forecasting
- A system that uses advanced algorithms and machine learning to predict future outcomes by processing historical data, reducing manual input and error.
- Model Drift
- The gradual change in model performance over time, often due to evolving data patterns which necessitates regular updates and scrutiny.
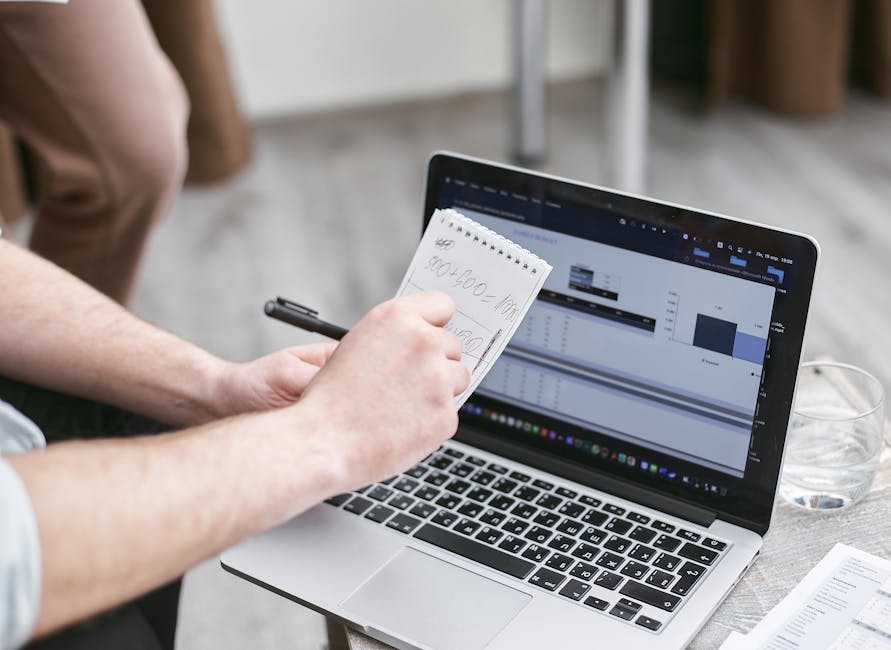
Pinpoint Precision in Forecasting
In today's competitive environment, mastering prediction is vital for business success. Historical examples—championed by innovators like Edouard Beaucourt—demonstrate how automation in report creation and analytical forecasts significantly reduces time and labor costs. Industries ranging from retail to e-commerce have evolved by integrating these historical shifts in performance management.
The Path to Automated Forecasting
Transformation starts with clear guidance: a series of steps that synthesize data using advanced tools reminiscent of methods practiced at AI21 Labs. Educational institutions, for instance, can enhance their KPI dashboards (such as enrollment metrics) through real-time updates.
Step | Tool | Time Saved |
---|---|---|
1 | Data Identification & Cleaning | High |
2 | Data Augmentation (e.g., ClicData) | Moderate |
3 | Multi-Model Orchestration | High |
4 | Integration & Monitoring | High |
Consider each step as an essential component to maintain accuracy and agility in forecasting. Optimizing these processes brings insights applicable to report automation, contract review, customer complaint summary, and forecast adjustments. |
Navigating Forecasting Challenges
Stop Guessing
While automation offers significant advantages, vigilance is crucial. Avoid pitfalls such as over-reliance on historical data without periodic adjustments and disregarding unstructured information accessible by natural language processing. Past efforts that failed to align forecasting with real-time dynamics led to costly setbacks. Always implement robust oversight and scenario analysis to ensure data streams—from learning management systems to other institutional sources—remain current and accurate.
Interactive Learning and Engagement
Engaging, interactive content is key for reinforcing learning. Incorporate quizzes that help spot data mishaps and checklists covering every forecasting facet—from data gathering to modeling and reviewing. This hands-on approach enables a smooth transition from theory to effective action.
Dig Deeper into Interactive Methods
Testing scenarios with real metrics from industries like consumer electronics and logistics bridges strategy with practice. For educational institutions, integrating forecasting into ubiquitous tools such as pivot tables lowers the manual workload while promoting swift decision-making.
Advanced Tactics and Permanent Gains
Your Spreadsheet Is Lying
Exploring how generative AI enhances predictive analytics unveils iterative process improvements not only in demand forecasting but also in contractual reviews. By transitioning to automated processes, organizations witness a breakthrough in forecasting accuracy and operational flexibility. This transformation chronicles the future of strategic planning, especially in education platforms where fluid student behavior necessitates agile predictive models.
-
①
Analyze current data infrastructure and identify integration gaps.
-
②
Implement automated tools like multi-model orchestration to streamline forecasting.
-
③
Conduct regular quality checks and update models to prevent drift.
-
④
Leverage generative AI insights to continuously refine the forecasting process.