The New Era of Precision Cost Forecasting
Imagine preparing for a big local football match only to discover that the weather forecast was based solely on yesterday’s conditions. That frustration mirrors the pitfalls of relying on outdated forecasting methods in supply chain cost analysis. Today, advanced regression models are emerging as the game-changer, offering robust statistical insights that refine cost predictions by capturing hidden variabilities and operational drifts.
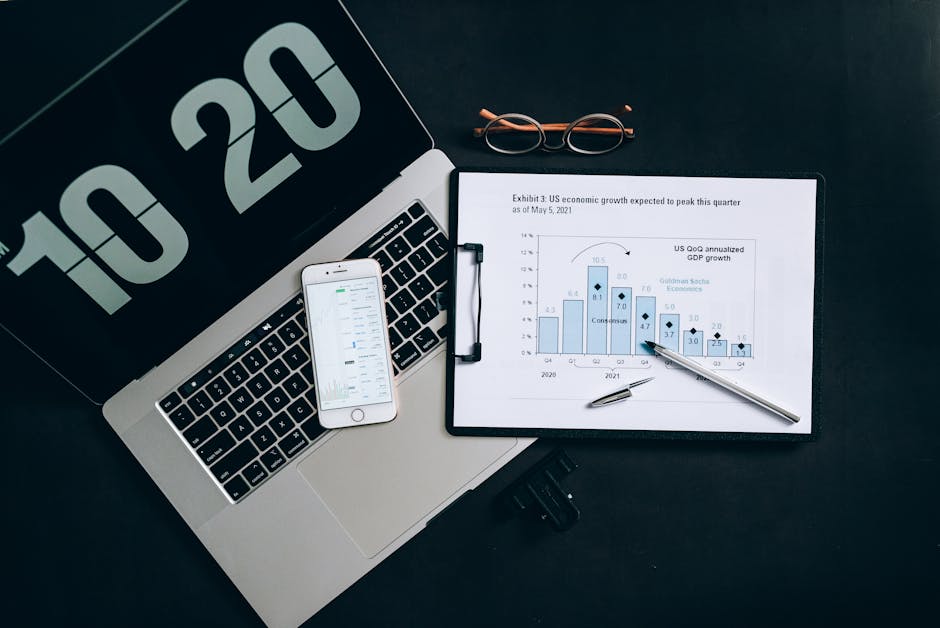
RMSE ↓ 18.4%
– a statistic that speaks to the technical depth behind these innovative models.
Technical Insights into Advanced Regression Models
Advanced regression models have evolved far beyond basic linear calculations. Techniques like multivariable and Poisson regression have emerged to navigate the complex, non-linear relationships that exist between operational costs and key performance indicators (KPIs). This layered understanding allows companies to refine their cost forecasting with greater precision.
- Multivariable Regression
- A statistical technique that involves more than one predictor variable to forecast an outcome.
- Poisson Regression
- A technique used for modeling count data and its relationship with a set of predictor variables.
However, challenges do exist. Just as incorrect weather predictions can ruin game day plans, issues such as model overfitting that distorts predictive accuracy can compromise forecasting efforts. To combat such pitfalls, experts employ methods like cross-validation, regularization techniques (e.g., LASSO and Ridge), and automated drift detection – techniques that help to ensure forecasts remain agile and reflective of real-time data.
Academic research has repeatedly shown that when these methods are rigorously applied, they can produce insights comparable to those seen in specialized fields like healthcare cost modeling.
Real-World Applications and Success Stories
Across numerous industries, advanced regression methodologies have transformed the way companies manage costs. Consider an enterprise that methodically reconciles diverse data streams – much like merging city-wide transport logs – to maintain accuracy in forecasting. Such efforts are reminiscent of the innovation seen in platforms like Microsoft’s cost management resources.
An illustrative case involves logistics leaders who integrated continuous real-time data adjustments, successfully reducing forecast errors. They combined insights from traditional linear regression with the flexibility of advanced analytics to optimize delivery routes and inventory allocations. These refined practices not only improve financial transparency but also promote operational efficiency by addressing scattered urban utilities data and aligning it into a cohesive forecast system.
Expert Perspectives and Common Pitfalls
Many industry experts emphasize that a deep understanding of model outputs is essential for effective forecasting. Echoing insights from reputable institutions like Harvard Business School, the synthesis of historical trends with real-time analytics is critical. Such an approach aids in identifying subtle KPI drifts, ensuring that models remain dynamic amid rapid operational changes.
"Combining historical data with real-time adjustments forms the crux of resilient cost forecasting. It's not just about the numbers; it's about understanding what those numbers mean in a dynamic marketplace."
Nevertheless, oversimplification can prove dangerous. When advanced models are applied without acknowledging the broader historical context, significant trends may be overlooked. This oversight is particularly problematic when trying to detect gradual shifts in key variables – a risk inherent in any forecasting effort that fails to fully leverage the depth of advanced analytics.
A Comparative Look at Model Performance Across Regions
Region | Model Type | Forecast Error Reduction | Key Considerations |
---|---|---|---|
Rotterdam | Multivariable | 15% | High variability in energy consumption |
Shenzhen | Poisson | 18% | Abrupt demand shifts |
London | Multivariable | 13% | Complex regulatory environment |
New York | Poisson | 16% | High consumer demand volatility |
Note: These comparisons illustrate the impact of using tailored regression models in diverse operational contexts. Relevant keywords include "multivariable regression," "Poisson regression," "cost forecasting," and "operational efficiency." |
Conclusion and Future Directions
In summary, embracing advanced regression techniques can transform cost forecasting into a precise and adaptive process. Much like a well-planned strategy before a derby match, integrating academic research with real-time data creates a forecasting system that not only anticipates market storms but also adjusts dynamically to them.
This discussion prompts us to ask: Are your models agile enough to weather sudden market storms? Could a blend of real-time analytics and rigorous academic research pave the way for breakthrough accuracy?
By confronting common pitfalls and capitalizing on advanced analytics, enterprises have the opportunity to reshape fiscal strategies, ensure sustainable operational efficiency, and ultimately, rebuild prompt chains that waste analyst time. Such a shift marks a pivotal evolution in controlling costs, fueling both innovation and resilient growth.
Tags: cost forecast tweaks, email response automation, finetuning misunderstood, openai, regression models