1. Context & Impact – The Precision Imperative
Data accuracy is not just an ideal—it is a necessity. Imprecise data creates a domino effect known as “data pipeline regret,” where errors in data reporting can disrupt operations, cost time, and undermine trust. Every misstep in your data stream can lead to costly operational fallout. I’ve helped teams translate business questions into prompt scaffolding that returns bulletproof, auditable output, ensuring that each data point drives the right decision.
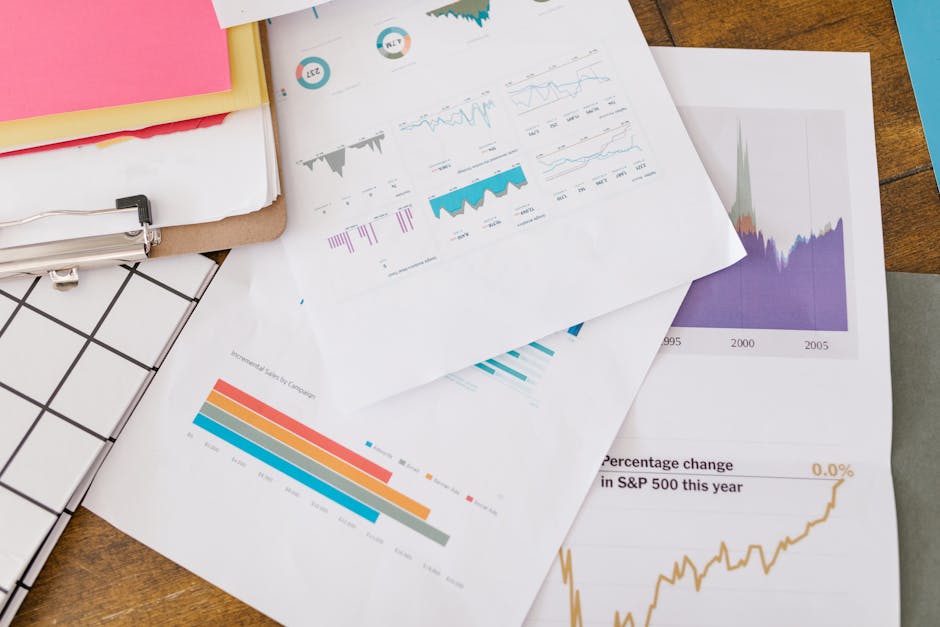
2. Diagnosing Data Flaws – Step 1: Data Source Scrutiny
Before you can fix a problem, you need to find it. A methodical examination of data sources is essential. Begin mapping out every connection in your data pipeline. Similar to the segmentation workflows and experiment tracking employed by industry leaders, this step requires tracing each element meticulously. Ask yourself: have you methodically traced every data element to locate and correct errors?
This approach not only identifies discrepancies but also sets the stage for ongoing improvements, ensuring that manual form cleaning and lead quality scoring remain effective.
3. Actionable Corrections – Step 2: Implementing Robust Solutions
The next step is taking decisive action to rectify data flaws. By combining manual data cleaning with automated lead scoring solutions, organizations can significantly enhance data reliability. Referencing tools such as Demandbase alongside insights from discussions on ZoomInfo alternatives, this strategy reinforces the creation of strong standard operating procedures (SOPs) and thorough contract reviews.
Real corrections come from robust systems that embody the real truths of business operations, ensuring that every data point is both accurate and actionable.
4. Real-World Illustrations – Step 3: Learning from Industry Giants
Insightful case studies teach more than theoretical concepts. From Google's innovative data validation processes to lively discussions on Reddit forums that reveal practical challenges, industry giants underscore the importance of stringent data management. These examples serve as a benchmark for building validation processes as rigorous as those used by top-tier companies.
By learning from these real-world implementations, leaders can better align their processes with proven strategies, minimizing risks and boosting lead conversion.
5. Prevention & Implementation – Step 4: Proactive Integration
The final piece is about building a forward-thinking framework that embeds heuristic logic and adaptive segmentation workflows into daily operations. Proactivity is the key to avoiding "data pipeline regret." This approach not only promotes precision by catching issues before they escalate but also empowers teams by streamlining lead generation strategies.
Adopting these transformative practices means fewer disruptions, enhanced team dynamics, and a sustainable competitive advantage.
Precision Tactics
Noisy Data | Precision Signals |
---|---|
High Error Rates | Less than 2% error rate |
Low Lead Conversion | 20% upswing in lead conversion |
Redundant Debugging | 30% reduction in debugging time |
Inconsistent Data Links | Streamlined, consistent data flows |
The table contrasts typical symptoms of imprecise data with targeted, precise signals leading to streamlined operations. Keywords: manual_form_cleaning, lead_quality_scoring, sop_generation, contract_review. |
- 1. Automated Anomaly Detection
- Implementation can drop manual debugging times by up to 30%, streamlining SaaS operations by pinpointing and rectifying data issues automatically.
- 2. Adaptive Segmentation Workflows
- This method facilitates parallel criteria testing, revealing hidden inefficiencies and benefiting asynchronous teams by ensuring that each segment of data is accurately categorized.