- Black Box Models
- Systems whose inner workings are hidden from end users, making understanding and improvement challenging.
- Explainability
- The ability to detail how AI systems reach their decisions, ensuring trust and accountability.
- Model Drift
- The gradual deviation of an AI model’s performance over time, often due to evolving data and contexts, necessitating continual evaluation.
Introduction
In today’s fast-paced technology landscape, the drive for innovation intersects with the need for ethical standards and clarity in decision-making. Organizations—from financial institutions to nonprofits—are increasingly incorporating AI into everyday operations. This includes generating standard operating procedures, detecting SQL gaps, and managing data flows with integrity. Transparent and traceable algorithms are not mere trends; they are a responsibility for those whose mission is to serve with integrity.
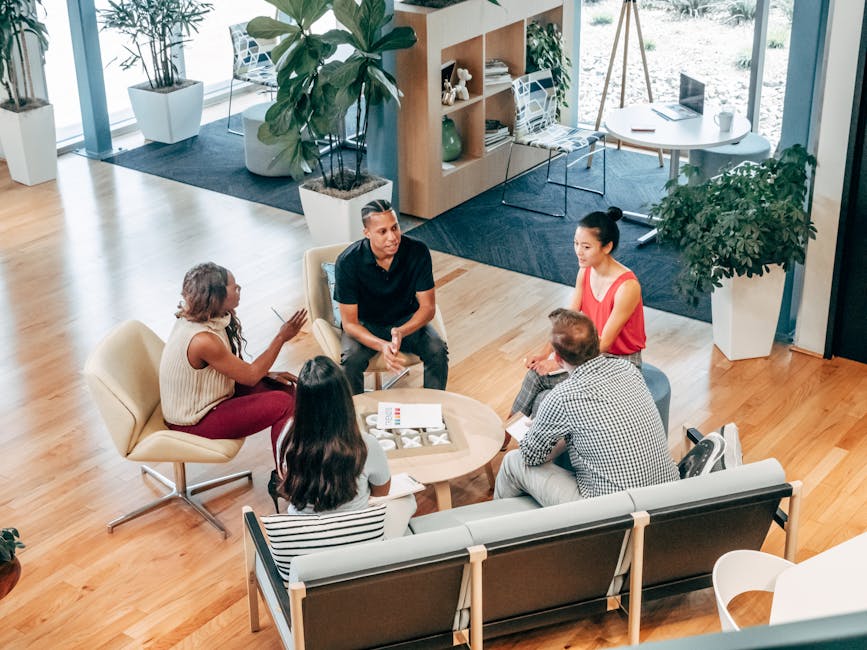
The Imperative of Transparent AI
Decisions made through opaque, unexplained algorithms can have lasting impacts. In high-stakes environments, every choice must be clear not only to technical teams but also to board members and donors. For example, automated dashboards monitor audit trails during financial reviews, helping mitigate errors caused by overlapping roles or manual data adjustments. This transparency directly influences the confidence stakeholders have in resource allocation and service delivery.
How do we ensure that every digital decision can be traced back to its source? The answer increasingly lies in policies that demand clarity and accountability from every AI application.
Real-World Impacts and Institutional Trust
In practice, institutions committed to ethical AI demonstrate noticeable improvements. Consider Suffolk University's National Center for Public Performance—they have shown that regulated, traceable AI systems lead to more predictable and reliable outcomes. In one notable instance, clear audit logs from automated systems helped reduce reconciliation errors during board reviews, re-establishing donor trust and operational reliability.
Below is a comparative analysis of AI tools used by organizations such as Give Directly and local food banks:
Tool | Use Case | Transparency Level |
---|---|---|
Give Directly AI Suite | Resource allocation analysis | High |
Food Bank Insight | Inventory & donor tracking | Moderate |
Community Care Analyzer | Beneficiary impact prediction | High |
Local Impact Tracker | Operational compliance | Moderate |
Considerations: The table highlights the importance of transparency in operational AI. Key search terms include resource allocation, audit trails, and compliance in nonprofit tech. |
Ethical Considerations and Implementation Best Practices
The path toward ethical AI implementation involves rigorous documentation and built-in transparency protocols. Experts in Responsible AI advocate for algorithm version control and comprehensive audit logs that provide step-by-step traceability. This approach is similar to IT compliance standards, where every modification is tracked and verified.
Embracing detailed testing cycles with iterative feedback minimizes risks associated with manual adjustments and conflicting roles. Such processes ensure that any emerging model drift is quickly identified and corrected, fostering an environment where ethical AI practices are not optional but standard.
More on Implementation Strategies
Best practices include:
- Regular algorithm audits
- Comprehensive version control
- Real-time data validation
- Stakeholder feedback integration
These measures help organizations maintain trust and deliver operational excellence.
Conclusion and Future Directions
Ethical AI is more than a buzzword—it is the cornerstone of trust and accountability in modern operations. Transparent decision-making not only inspires confidence among stakeholders but also positions organizations at the forefront of innovation. By committing to clear, traceable models, organizations ensure that technology serves its true purpose: reliable service and improved impact.
As we move forward, the lesson is clear: if you can’t explain it, don’t deploy it.
- If you can’t explain it, don’t deploy it.