Embracing an AI-Driven Future in Retail
Digital transformation in retail is redefining everyday operations. With a focus on automation and real-time data, retailers are experiencing improved efficiency and decision-making. By breaking down outdated processes, industry leaders are leveraging artificial intelligence to reduce operational delays and boost responsive strategies.
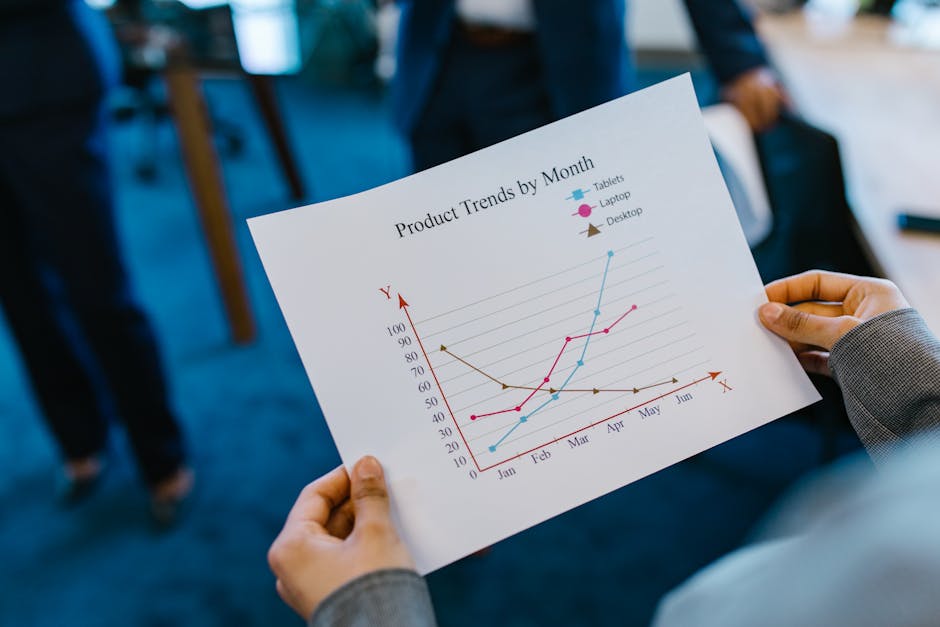
Revolutionizing Cost Forecasts and Operational Efficiency
Innovative AI integration has emerged as a catalyst for enhanced cost management. Retailers are using advanced forecasting models to minimize overheads and fine-tune supply chain decisions. Major industry players like IBM and SAP have demonstrated expansive success with data fabric approaches and AI-driven supply chain solutions. These tools provide an accurate pulse of the market, ensuring decisions are made quickly and efficiently.
Industry Case Studies: Proactive Alert Systems
Real-world transformations stand as a testament to AI’s effectiveness. One prominent North American retailer revamped its KPI dashboards to incorporate automated alerts that pinpoint supply chain misalignments. This strategic overhaul has reduced delays and improved customer satisfaction, proving the value of a proactive approach in handling complex retail dynamics.
Enhancing KPI Dashboards for Immediate Insights
KPI dashboards are evolving. From static displays to dynamic, AI-enhanced platforms, decision-makers now have access to actionable insights. Upgrading dashboards with semantic search and logic pathways eliminates the traditional data-to-decision lag. This streamlined process empowers teams to act on precise data quickly.
Navigating the Buy vs. Build Debate
The choice between off-the-shelf AI solutions and custom model development continues to spark discussion. Legacy constraints and underused AI tools can complicate custom builds. However, pre-built solutions offered by industry giants deliver fast implementation and scalability, effectively addressing the challenges of legacy systems while maintaining strong dashboard performance.
Advanced Modeling, Logic, and Data-Driven Strategies
Techniques like survival analysis are becoming standard in retail forecasting. These methods extend beyond simple failure prediction and refine inventory management by accurately identifying product lifecycles. With enhanced modeling strategies, retailers transition from basic metric tracking to strategic automation, fortifying resilience and sharpening decision-making processes.
Data Comparison: Pre-AI vs. Post-AI KPI Workflows
Task | Time | Accuracy | Human Input |
---|---|---|---|
Data Collection | Hours | Moderate | High |
Data Analysis | Hours | Low | High |
Alert Generation | Variable | Inconsistent | Significant |
Real-Time Adjustments | Days | Low | High |
After AI Integration | Minutes | High | Minimal |
The table highlights the transformation in operational dynamics. Look for keywords such as automated KPI alerts, real-time metrics, semantic search, and AI-driven decision-making when exploring similar case studies in retail automation. |
The Road Ahead: Unleashing the Full Power of Retail Automation
Retail is on the brink of a significant transformation where agile processes and precise data converge. As the industry continues to improve KPI dashboards with automated logic and lightweight data cleaning, the future points toward a seamless data-to-decision journey.
- Retail Metrics Fatigue
- Overwhelming traditional dashboards that fail to provide clear, actionable insights.
- Decision Lag
- A delay between data collection and decision-making, often caused by manual data analysis processes.
- Automation Debt
- The long-term cost and inefficiencies incurred by sticking to outdated manual workflows.
How can retailers quickly adapt to these challenges with AI? The answer lies in intelligently designed dashboards and streamlined AI workflows that directly target these pain points by reducing manual intervention while maximizing actionable insight.
AI vs. Legacy: A Flowchart of Decision Paths
The following flowchart summarizes the decision paths between AI-driven solutions and legacy systems:
- Data Input: Raw data collection (legacy: manual, AI: automated sensors)
- Data Processing: Batch processing versus real-time analytics
- Insight Generation: Periodic reports versus dynamic dashboards
- Decision Implementation: Delayed actions versus instant, AI-enabled responses
This progressive shift enables seamless, efficient decision-making and underlines the strategic advantage of adopting AI in retail operations.