AI-Driven Sales Forecasting: Customized Strategies for Marketplaces
Setting the Stage
This piece explores how modern artificial intelligence is reshaping sales forecasting in dynamic marketplaces. It walks through the transition from traditional, static metrics to agile, predictive models that empower precise reporting and strategic decision-making.
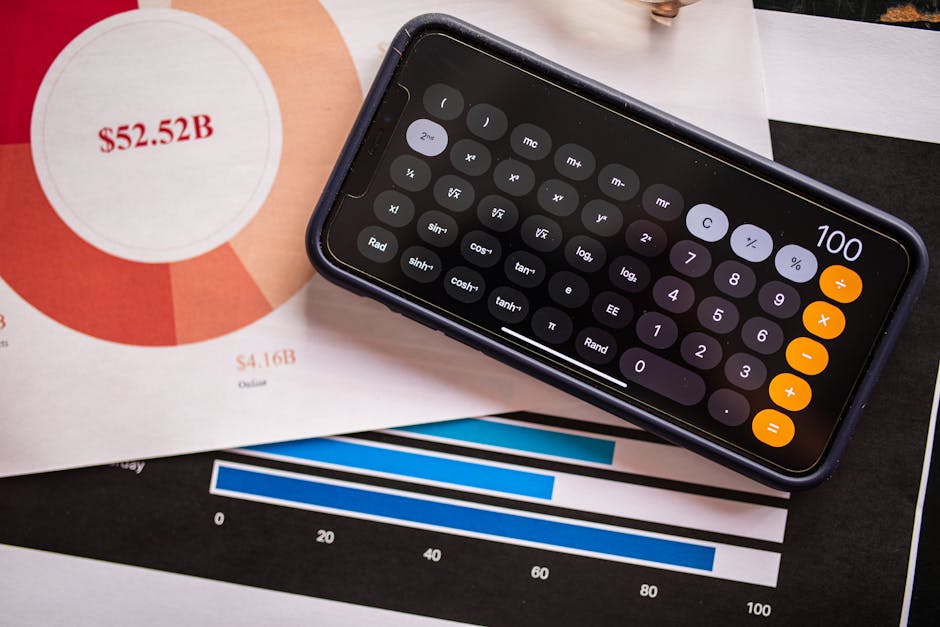
Industry Insights and Lessons
Drawing inspiration from industry pioneers and data-driven marketplaces, the narrative unpacks how detailed analyses and forecast adjustments are critical to operational excellence. Notable examples—such as methods inspired by renowned ride-sharing platforms—demonstrate that embracing anticipatory models can drive better alignment of metrics and reporting across teams.
AI Innovations and Technological Breakthroughs
Major advancements in AI, including breakthroughs from well-known companies like Google Gemini, have paved the way for more refined sales forecasting techniques. By integrating advanced classification models that capture non-linear sales trends and adjusting for seasonal variations, businesses can now manage challenges related to misaligned metrics more proactively and effectively.
Tailored Strategies for Success
Implementing these forward-thinking approaches involves a series of step-by-step strategies:
- Assessing and realigning built-in KPIs to better measure sales performance.
- Leveraging ensemble modeling techniques that blend classical statistics with machine learning algorithms.
- Capturing and analyzing the volatile effects within diverse sales channels for actionable insights.
Deep Dive: Ensemble Modeling Components
Ensemble modeling combines various strategies to improve forecast accuracy. For example, while traditional statistical models provide a stable baseline, modern machine learning algorithms help adapt to sudden market shifts, ensuring reports stay relevant even as market dynamics change.
Comparing Forecasting Approaches
Old Guard | Neural Edge |
---|---|
Static models | Adaptive, dynamic algorithms |
Delayed responsiveness | Real-time data integration |
Reliance on historical data | Predictive analytics incorporating future trends |
Siloed reporting | Integrated, cross-functional insights |
Keywords: forecast adjustments, classification models, seasonal skew, demand elasticity, velocity decay |
Marketplace Forecasting Terms Explained
- demand elasticity
- This term defines the responsiveness of the quantity demanded relative to changes in price, a critical metric in adjusting sales forecasts.
- seasonal skew
- Represents unusual variations in buying patterns due to seasonal events, influencing forecast precision.
- velocity decay
- Describes the diminishing impact of a sales surge over time, essential for smoothing out forecast anomalies.
Conclusion
Adopting targeted AI strategies transforms traditional forecasting challenges into competitive advantages. Precise, emotionally resonant forecasting not only improves internal reporting but also builds the confidence needed to drive marketplace success. The integration of advanced algorithms and detailed KPI analyses ensures teams are well-equipped to navigate an ever-changing sales landscape.