Urgent Insight: Retail's Evolving Forecasting and Attrition Detection
Introduction
In today’s dynamic retail environment, forecasting techniques and attrition detection are undergoing rapid transformation. Cross-functional analysts and enablement leads are increasingly relying on data-driven insights during recurring forecast updates and quarterly operational reviews. Traditional spreadsheets are giving way to sophisticated real-time predictive models that track market trends and local events.
- demand sensing
- A method of capturing real-time demand signals from various sources, allowing for more responsive inventory and pricing adjustments.
- attrition flags
- Indicators that help identify early warning signs of employee turnover, enabling preemptive strategies to stabilize workforce performance.
- inventory lag
- A situation where inventory levels fall behind consumer demand due to delayed forecasting or supply chain disruptions.
Exploring Advanced Forecasting Techniques
Retail powerhouses have embraced advanced forecasting models that integrate external economic variables. These techniques go far beyond simple historical data. For example, teams on Chicago’s Magnificent Mile have successfully incorporated local event data into real-time sales forecasts, reducing inventory costs and achieving strategic advantages. Such case studies underscore the potential for improved planning and reduced waste.
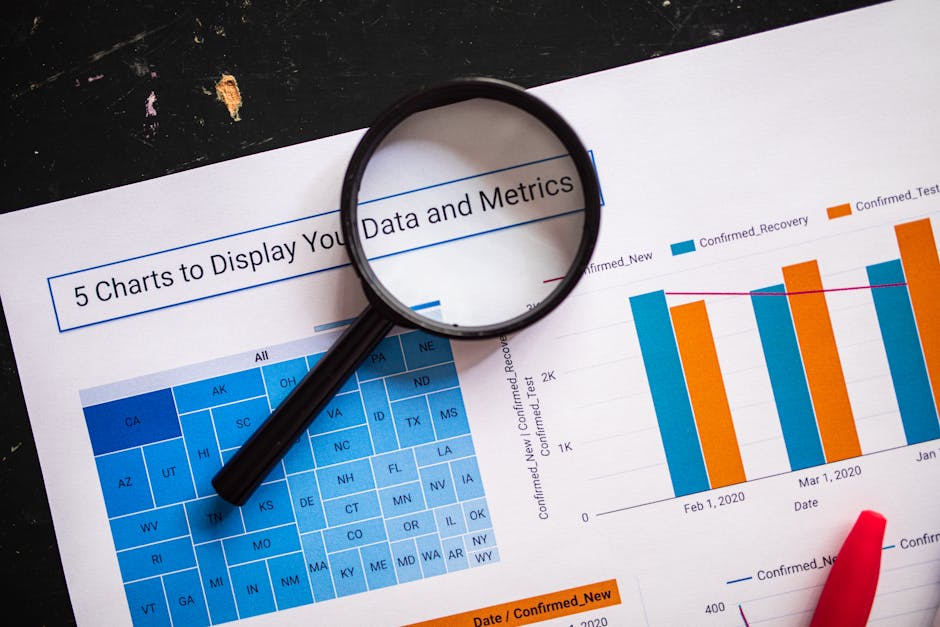
Data Integrity and Early Attrition Detection
A significant, yet often overlooked, cost in the retail sector is staff turnover. Studies like Oxford Economics’ “The Cost of Brain Drain” illustrate the financial impact of high attrition rates. A boutique chain in Atlanta, for example, used automated form cleaning to improve data verification, which led to a 15% improvement in forecasting accuracy and a tangible decrease in turnover costs.
By identifying early attrition flags, organizations can intervene before issues escalate, ensuring stability in workforce performance, even amidst fluctuating market conditions.
Comparative Analysis of Forecast Models
Region Comparison | What’s Broken | What’s Working |
---|---|---|
SoMa | Inventory Lag due to delayed data updates |
Fast response to local events |
Flatiron | Underestimation of demand peaks | Effective use of real-time analytics |
Magnificent Mile | Overreliance on historical patterns | Integrated event-driven forecasting |
Downtown Core | Inefficient data cleaning methods causing noise | Streamlined, automated verification processes |
Note: This table emphasizes the contrast between outdated traditional methods and innovative solutions driving better forecast accuracy. Keywords: retail analytics, predictive models, workforce stability. |
Innovative Modeling Approaches and Impact on Decision-Making
The integration of techniques such as clustering analysis and SQL-to-prompt pipelines is revolutionizing retail forecasting. Major AI innovators have demonstrated how these methods uncover hidden patterns, which in turn drive strategic advantages. An iterative validation process—similar to agile sprint reviews in tech startups—enhances model adaptation to market shifts and localized factors. This agile approach ensures that decision-making is both precise and responsive.
Retailers are now positioned to harness the full potential of advanced analytics, paving the way for a future where operational insights are woven directly into strategic planning.
Future Strategies and Implementation
The transformation in forecasting indicates a clear path forward. Integrating external data sources, automating manual data cleaning, and deploying custom GPT models are key strategies recommended for addressing operational complexities. By embracing these technologies and focusing on accurately capturing local economic variables, retail leaders can make informed decisions that propel sustained growth.
In summary, the evolution of forecasting and attrition detection in the retail sector is a call to action for decision-makers: modernize data practices, invest in innovative analytics, and never underestimate the impact of localized insights. This paradigm shift will be essential for competitive advantage in an increasingly complex market.
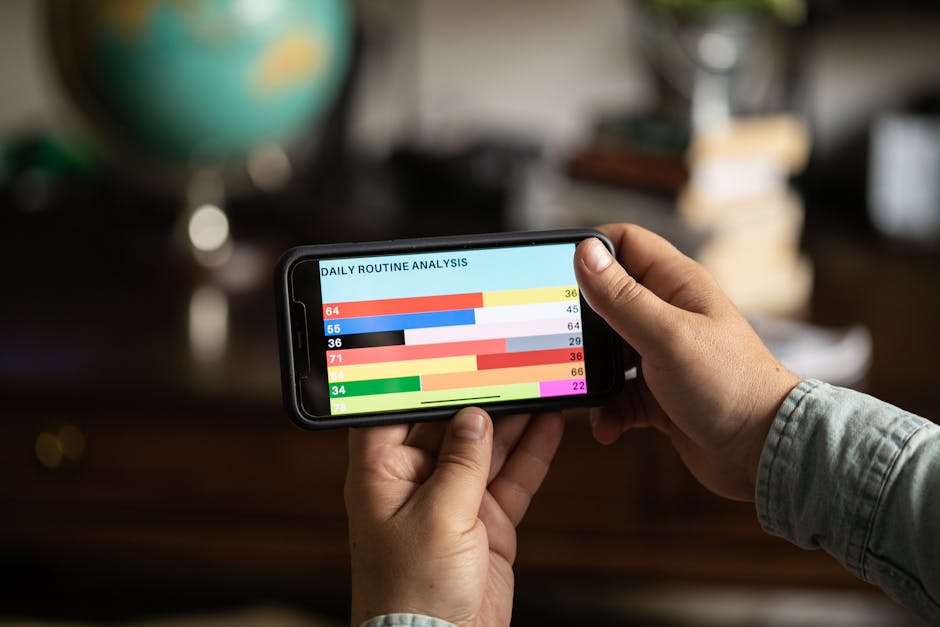