Introduction
This guide highlights the critical role of mastering internal knowledge within education institutions. It emphasizes that effective document retrieval is not just about accessing data, but about ensuring that internal systems command trust through measurable metrics. With actionable AI-driven techniques, institutions can transform raw data into powerful insights.
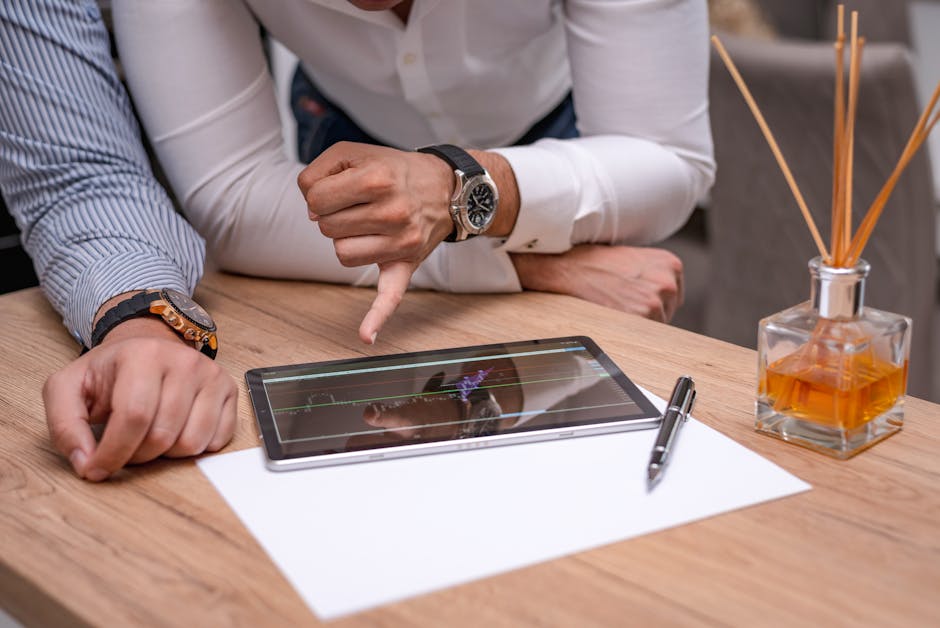
Establishing Trust in Metrics
Metrics form the backbone of any successful retrieval process. Trust in these metrics is paramount; a single lapse can lead to misguided strategies and wasted resources. Much like the rigorous validation procedures in the pharmaceutical industry, robust metric systems in education can revolutionize document management and ensure the accuracy of retrieved information.
Streamlining Document Retrieval
Precision in document retrieval should mirror the analytical rigor seen in FDA label comprehension studies. By integrating user-centric design principles and prioritizing rigorous quality control, internal systems can efficiently manage extensive volumes of knowledge. This approach ensures that documents remain accessible and verifiable for internal audits and reporting.
Fine-Tuning Models: Strategy and Process
The guide details a fine-tuning process based on consistent, step-by-step adjustments aligned with embedding space analysis. Personalizing AI models through real-world data input is essential. Iterative refinements guarantee that models stay relevant and effective, echoing the continuous improvement seen in pioneering technology initiatives. Below is an explanatory definition of some insider terms:
- retrieval-augmented generation
- A process where external documents support AI-generated content, enhancing context and accuracy.
- latent knowledge drift
- The gradual misalignment of stored information over time, affecting retrieval precision if not periodically calibrated.
Leveraging Embedding Space Analysis
Embedding space analysis reveals hidden relationships within vast document collections. By mapping semantic similarities, institutions can dramatically enhance retrieval speeds and reduce errors. This sophisticated technique is akin to mapping neural networks in leading research centers—transforming how information is accessed and prioritized.
Dispelling Common AI Misconceptions
Many misunderstandings about AI fine-tuning persist. Often, the complexity of model training is significantly overstated. Drawing on evidence-based processes—similar to those used in healthcare safety studies—the guide clarifies that systematic, iterative testing can demystify AI challenges and ensure reliability and consistency.
Evidence from Real-World Applications
Insights from reputable studies, including those by the National Center for Biotechnology Information, reinforce the guide’s recommendations. Research on meticulous data verification and medicinal safety user testing offers a compelling model that can be applied to educational document systems, ensuring that every retrieval process is both reliable and effective.
Step-by-Step Fine-Tuning Process
The following process outlines how institutions can refine their document retrieval systems:
Process Flow
- Baseline Metrics: Begin with a careful analysis of current retrieval systems to establish initial performance metrics.
- Data Collection: Gather historical usage data and preprocess it for analysis.
- Iterative Fine-Tuning: Implement targeted adjustments in embedding space to refine AI accuracy.
- Revalidation: Test against real-world document scenarios to ensure sustained metric reliability.
Real-World Case Examples
Remote-first education institutions, such as prominent innovative schools, have implemented similar strategies with notable success. These institutions report improved document accessibility, a reduction in retrieval errors, and increased confidence in data metrics. Their approach serves as a practical example for others looking to bridge theory and practice.
Implementation Roadmap
A well-planned roadmap is essential for rapid deployment of these strategies. Key components include robust testing protocols, continuous stakeholder feedback, and ongoing monitoring. For example, integrating iterative spreadsheet-based metric monitoring with conditional formatting and version-controlled tracking has proven to enhance data validation, ensuring that every phase of the upgrade is measured and trusted.

Comparative Analysis of Retrieval Methods
Method | Latency | Accuracy | Cognitive Load |
---|---|---|---|
Baseline Retrieval | High | Moderate | High |
Iterative Fine-Tuning | Moderate | High | Moderate |
Embedding Space Analysis | Low | Very High | Low |
Hybrid Approach | Moderate | High | Low |
Considerations: Evaluate your institution's needs against latency, accuracy, and cognitive load. Keywords for further research: document retrieval, AI fine-tuning, embedding space analysis, streamlined metrics. |
Conclusion and Impact
The journey to a robust document retrieval system in education is paved with meticulous fine-tuning, strategic embedding space analysis, and a steadfast commitment to reliable metrics. This guide urges education institutions to embrace these advanced strategies to maintain a competitive edge and ensure that every piece of internal knowledge is trustworthy, accessible, and actionable.
How does embedding space analysis dramatically improve document retrieval accuracy? By mapping semantic relationships with precision, this method enables institutions to retrieve documents rapidly and accurately—providing the bedrock for a trusted, agile reporting framework.
Integrating these methodologies—not only streamlines document management but also optimizes internal reporting and metric monitoring, ensuring that strategy and execution work hand in hand.