Article Overview
This article delves into how experimental tracking and real-time data analytics are revolutionizing cost forecasting within ecommerce. By integrating AI-driven experimentation, businesses swiftly detect anomalies and refine their financial strategy, ultimately increasing operational efficiency and market responsiveness.
Conceptual Background
- Introduction
- Precision tracking systems merge iterative experimentation with real-time data flows. This integration equips teams with accurate insights to flag cost anomalies quickly.
- The Evolution of Forecasting in Ecommerce
- Traditional forecasting has evolved from historical data reliance to dynamic, live analytics. Leading platforms have embraced this shift, harnessing customer behavior, inventory metrics, and market trends.
- Real-Time Data Analytics
- Live streams of data not only pinpoint inefficiencies but also allow for continuous, agile adjustments to forecasts, ensuring that financial strategies stay ahead of market shifts.
- Iterative Experimentation
- Structured experiments and feedback loops refine algorithmic models, reducing forecasting errors and enabling proactive decision-making.
- AI-Driven Anomaly Detection
- Machine learning models, updated via iterative feedback, enhance the detection of cost anomalies, mitigating risks before they escalate.
- Case Studies and Industry Benchmarks
- Initiatives from industry leaders, including those from Prescience Decision Solutions, demonstrate how integrating diverse data sources can boost demand forecasting accuracy to over 95%.
- Future Directions
- The fusion of experimentation with live analytics is setting new standards for cost forecasting. As these methods mature, their influence on strategic decision-making will continue to expand.
Enhanced Metrics and Methodologies
The adoption of intricate metrics, such as cost-per-acquisition adjustments and demand forecasting variance, bridges conventional methodologies with modern analytics. Experimental tracking methodologies using tools like MLflow and Weights & Biases quantify algorithm adjustments with precision.
Controlled A/B testing further refines anomaly detection techniques, transitioning financial planning from reactive to a proactive forecasting model—an essential evolution for contemporary ecommerce strategies.
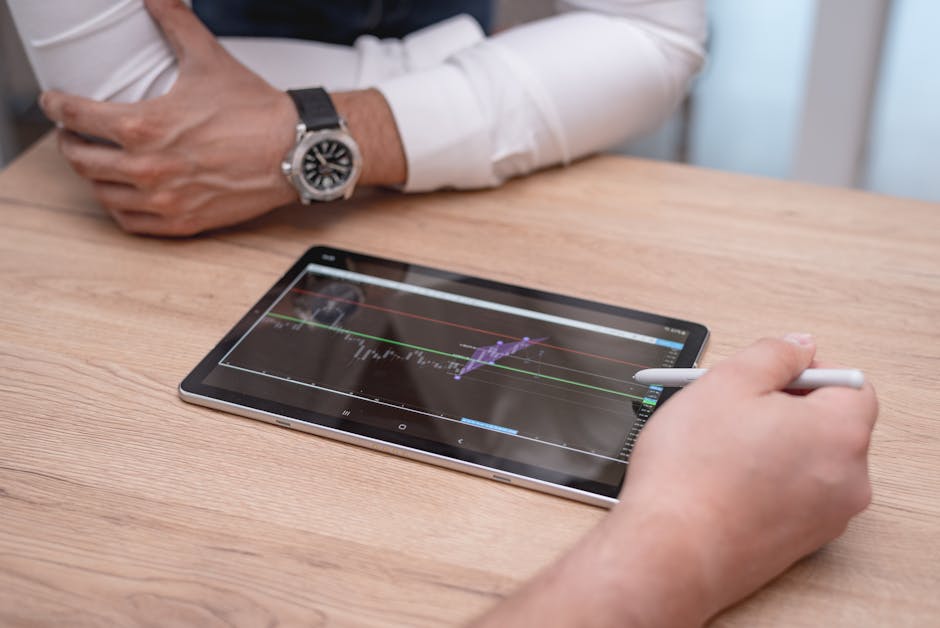
Comparative Analytics of Tracking Tools
Tool | Latency | Cost Efficiency | Integration Ease |
---|---|---|---|
MLflow | Low | Moderate | High |
Weights & Biases | Very Low | High | Very High |
Other Emerging Tools | Medium | Variable | Medium |
Traditional Systems | High | Low | Low |
Note: This table highlights key performance areas that are critical in determining efficient experiment tracking. Keywords include latency, cost efficiency, and integration ease. |
Key Industry Terms
- Attribution Drift
- The gradual shift in causal relationships between marketing campaigns and resulting sales outcomes over time.
- SKU-Level Forecasting
- Forecasting techniques that analyze cost and sales data down to the individual product unit, allowing granular insights.
- Model Decay
- The deterioration in the performance of predictive models over time as market dynamics change, requiring periodic recalibration.
Closing Thoughts
The integration of precision experimental tracking with real-time analytic systems represents a transformative approach to cost forecasting. By embracing iterative testing, continuous feedback, and advanced AI tools, ecommerce platforms empower their teams to stay ahead of unpredictable market shifts. This evolution not only enhances forecasting accuracy but also drives strategic decisions that benefit overall operational performance.
As technology advances, the capacity to harness precise, actionable data will further sharpen financial forecasts, ensuring that organizations operate with agility and foresight in today's dynamic marketplace.
Stay informed and adapt swiftly—the future of ecommerce dynamics is built on data-driven decision making.