INTRODUCTION
A renowned Silicon Valley tech leader once turned a turbulent churn crisis into a data-driven success story. By leveraging advanced document retrieval techniques, platforms like BigQuery, and insights drawn from Google's AI triumphs, executives were able to integrate multi-source data effectively and preempt customer churn. This approach serves as a real-life playbook for converting potential losses into strategic opportunities.
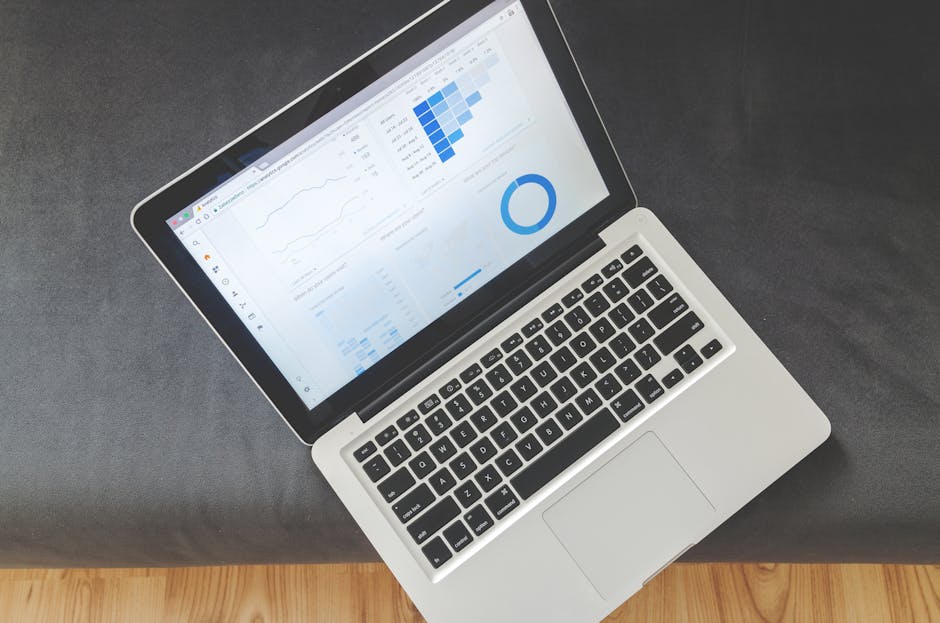
STEP-BY-STEP DOCUMENT RETRIEVAL
This section dissects the key stages of a robust document retrieval process, reminiscent of industry giants. The procedure involves:
- Aggregating Multi-Source Data: Combining data from various platforms into a unified repository.
- Semantic Search Algorithms: Utilizing algorithms that understand context and nuances.
- Refining Queries: Employing domain-specific language models to spot churn indicators early.
Learn More About Semantic Search Techniques
Semantic search relies on understanding the context around words rather than matching exact keywords. This method improves the discovery of critical patterns hidden in extensive data sets. By refining search queries based on industry language, companies can pinpoint early warning signs of churn that traditional methods might miss.
NAVIGATING PITFALLS IN DATA INTEGRATION
Even the best strategies can face setbacks. Industry research and real experiences highlight several pitfalls:
- Misaligned Reporting Flows: Data from various sources may not always communicate effectively.
- Misconfigured BI Systems: Even minor setup errors can lead to significant misinterpretations.
- Overlooked Data Nuances: Small details in the data can be the key to spotting early-stage churn.
For those managing diverse data sources, attention to detail and timely adjustments are crucial. The key is to implement processes that double-check alignments and configurations at every step.
BUSINESS IMPACT OF EFFECTIVE RETRIEVAL
Effective document retrieval isn’t just about managing data—it’s a strategic tool that fuels decision-making. Early detection of churn signals translates into:
- Custom integration tools that tailor customer retention efforts.
- Synchronized feedback loops, ensuring all functions are aligned.
- Boosted profitability through timely, informed actions.
By drawing parallel to leading enterprise marketing software innovations, it becomes evident that a well-oiled retrieval process can revolutionize customer relationship strategies.
VISUAL INSIGHTS & REFLECTION
This final section challenges you to consider:
- What hidden patterns in your data could serve as cost-saving treasures?
- How might refining your retrieval methods transform overall business outcomes?
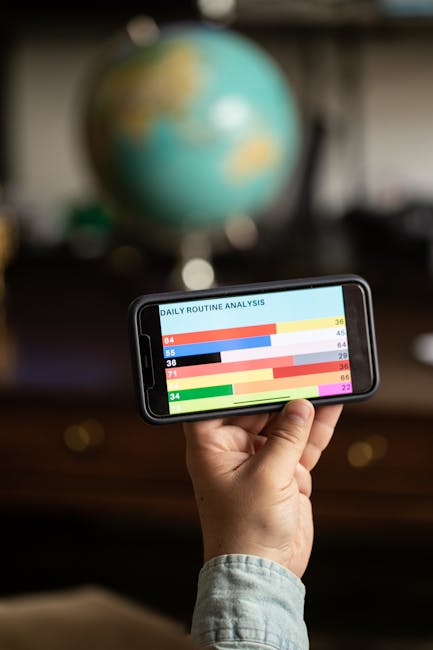
These reflective questions aim to empower professionals to take actionable insights away from the discussion, transforming theoretical approaches into practical, strategic results.
Key Churn Signals
- inactivity decay
- An indicator signifying declining engagement with the product over time.
- support fatigue
- A measure of customer frustration due to repetitive or ineffective support interactions.
- silent dissatisfaction
- The lack of positive feedback or engagement that often precedes churn in long-term customers.
Anecdotes & Retrieval Techniques
Remote Team Anecdotes | Retrieval Techniques |
---|---|
Instances of Slack ghosting in asynchronous communications. | Usage of BM25 for high precision in data searches. |
Delayed responses in globally distributed teams. | Integration of dense vector embeddings for contextual search. |
Miscommunication due to differing time zones and workflows. | Refinements in domain-specific query language models. |
Reliance on anecdotal evidence leading to overlooked patterns. | Systematic multi-source data aggregation to identify churn signals. |
Considerations: Align processes, verify alignment between data sources, and continuously adapt retrieval methods to evolving business needs. Keywords: churn detection, no code ai limitations, semantic search. |