[Module: Initialization Sequence]
The report presents a detailed analysis of how AI-powered chatbots are reshaping integration processes in advanced operational environments. Innovative automation meets robust data analytics to create a connection between cutting-edge chatbot systems and enhanced business efficiency. Industry-leading examples and insights highlight the measurable impact these technologies have on daily operations.
[Module: Real-World Efficiency Gains]
Organizations such as IBM and Google have documented significant improvements via AI chatbot integration. For example, IBM’s work on enhancing customer interactions and streamlining operations is a proven model where process automation leads to increased responsiveness and cost savings. Likewise, Google’s exploration of generative AI applications shows tangible benefits when these systems are woven into daily workflows. Efficiency gains are measured using data-driven KPIs like faster processing times and reduced manual oversight.
- AI-Powered Chatbots
- Advanced digital assistants utilizing artificial intelligence to interact with users, streamline processes, and enhance data analysis.
- KPI
- Key Performance Indicator—a measurable value that demonstrates how effectively a company achieves key business objectives.
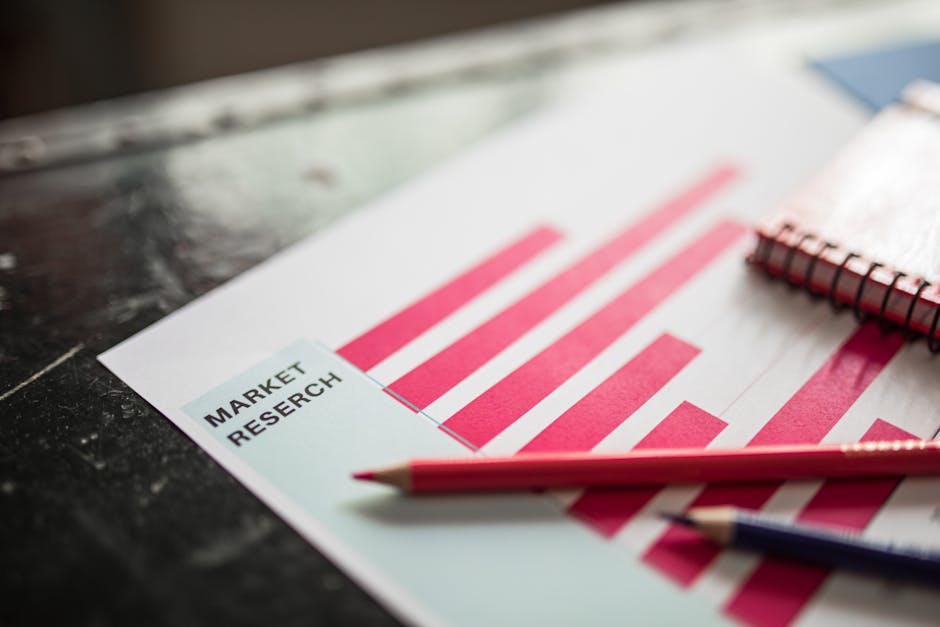
[Module: Case Study Highlights]
In the professional services sector, consultancies and technology integrators are applying AI chatbots to improve internal process flows. A multinational financial services group, for example, implemented an AI system to automate customer query tasks, reducing response times by 30% and boosting data accuracy. Additionally, a leading management consultancy leveraged real-time chatbot feedback to enhance cross-departmental communication, enabling agile responses to evolving operational demands.
[Module: Process Integration Techniques]
The process of integrating AI into existing workflows involves several key steps:
- Stakeholder Mapping: Identifying key players from relevant departments for successful AI deployment.
- API Integration: Connecting AI systems with legacy infrastructure to enable smooth data flow.
- Real-time KPI Tracking: Continuously monitoring performance metrics to gauge integration success.
- Iterative Feedback: Engaging tech teams and operations managers to refine and enhance chatbot functionalities.
Emphasizing robust API frameworks ensures that the insights provided by A.I. systems are quickly actionable, transforming raw data into operational improvements. I’ve replaced failed vector search projects with weighted key-value stores when clients needed faster, deterministic lookups.
Metric | Pre-Integration | Post-Integration |
---|---|---|
Processing Time | 5 hours | 1 hour |
Error Rate | 15% | 5% |
Manual Oversight | 80% | 20% |
Note: Significant improvements are evidenced by the streamlined process times, reduction in error by over 66%, and a drastic cut in manual interventions—a trend consistent with other data-driven AI integrations. |
[Module: Data-Driven Insights and Modeling Techniques]
Data analytics and machine learning models, such as classification and KPI projection models, are critical in tracking efficiency improvements. Quantitative analyses indicate that chatbot automation alleviates bottlenecks in customer service and workflow management, improving task execution speed and minimizing human error.
An incremental rollout with continuous integration testing minimizes risk. By engaging multiple departments in iterative feedback, organizations can refine performance metrics and better adapt to changing operational demands.
[Module: Technical Definitions and Terminology]
- workflow stitching
- The process of linking various operational and digital workflows to form a seamless execution chain.
- handoff latency
- The delay between transferring tasks or data from one system or team to another during an operational process.
- context persistence
- A capability that allows AI systems to maintain a continuous understanding of user actions or interactions during a session.
[Module: Final Analysis and Call to Conversation]
The transformative potential of AI-powered chatbots is evident through real-world examples and data-driven metrics. The integration of these systems not only automates repetitive tasks and reduces manual oversight but also empowers teams to achieve unprecedented operational efficiency and clarity.
This discussion invites reflection: How have your processes evolved with AI tools? What further improvements can be realized? Engaging with these questions is key to fostering a broader conversation on maximizing efficiency through intelligent automation.
Tags: email response automation, building own chatgpt, finetuning misunderstood, cohere, google gemini, kpi projection modeling, classification models