System Alert: Enhancing Patient Retention With AI-Driven Insights
Comparative Metrics Across Provider Types
Provider Type | Churn Rate (%) | Average Stay (months) | Intervention Success (%) |
---|---|---|---|
Urgent Care | 8.5 | 12 | 72 |
Telehealth | 15.2 | 9 | 65 |
Primary Care | 5.1 | 18 | 80 |
Specialty Care | 7.8 | 14 | 75 |
Data derived from heuristic modeling and industry reports. Keywords: churn detection, data dashboards, healthcare retention. |
Real-World Churn Spike and Data-Driven Responses
A dramatic post-COVID telhealth dip revealed a surge in patient discharges at several institutions. Leading centers like Mayo Clinic and Cleveland Clinic spearheaded efforts by integrating AI-driven churn analytics within their operational dashboards. The advanced algorithms pinpointed areas of concern—identifying patients at risk of becoming silent disenrollees through both segmentation workflows and regression models.
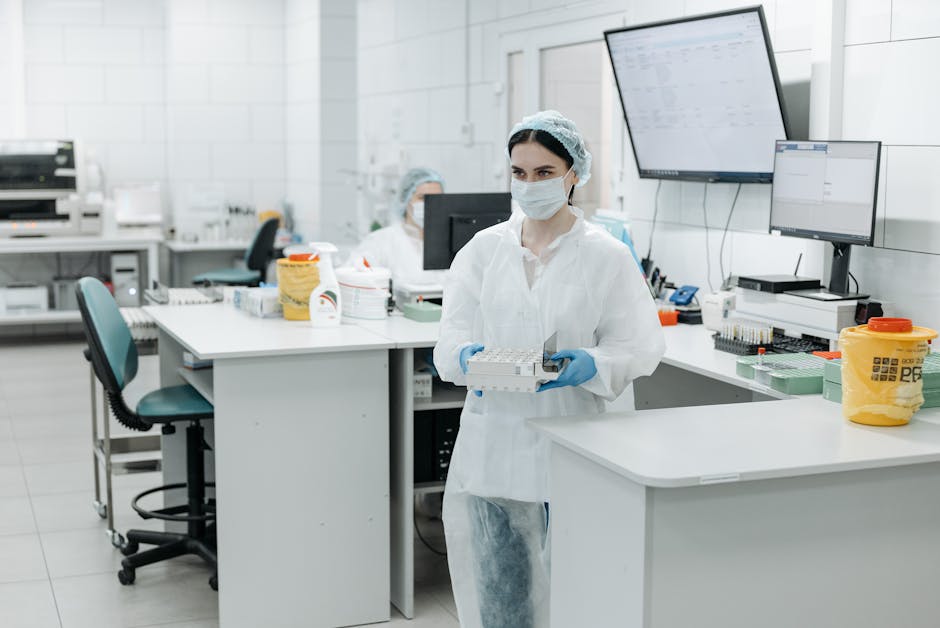
Segmentation Workflows: Customizing Interventions
Sophisticated segmentation workflows partition patient data based on demographics and clinical nuances, enabling personalized interventions. This method has been essential in identifying high-churn periods that align with specific operational constraints. It diverges sharply from generic retention tactics by tailoring efforts to each patient segment.
Learn more about segmentation benefits
In one prominent case, a regional provider noted that correlating specific seasonal trends with patient segments resulted in a 10% improvement in retention rates. Data dashboards integrated with these workflows highlighted subtle differences across age groups and treatment categories, triggering timely interventions.
Regression Models: Predicting and Preventing Churn
Regression models provide powerful predictions by analyzing historical data to forecast patient behavior anomalies. This predictive approach enables healthcare teams to allocate resources efficiently and implement effective retention programs before issues escalate. The data-driven insights empower decision-makers to anticipate rare but critical events, refining the overall patient care strategy.
Overcoming Implementation Pitfalls
Despite the promising capabilities of AI, internal build failures and data inconsistencies have posed significant challenges. Issues such as flawed data feeds and suboptimal system performance are common pitfalls. To tackle these challenges, continuous data validation and adaptive A/B testing cycles are implemented, ensuring smooth integration with existing Electronic Health Record systems.
“Rigorous quality assurance is the cornerstone for successful AI deployment in healthcare; without it, even the most advanced systems will falter.”
Evolution of Data Dashboards for Operational Excellence
Data dashboards have come a long way from static reports to dynamic, interactive platforms. Modern dashboards, inspired by innovations from platforms like June and Coupler, provide real-time patient metrics and actionable insights. Their design transforms complex data sets into clear visualizations, facilitating better decision-making on the ground.
Actionable Insights: Integration & Automation
With the integration of segmentation workflows and regression analysis, healthcare dashboards now offer automated alert systems. These systems identify real-time anomalies and deploy predictive triggers that significantly reduce unexpected patient churn. As a result, teams can quickly adjust strategies and focus on proactive patient care.
Additional insights on integrating data-driven tools
Incorporating advanced analytics not only refines patient retention strategies but also assists in operational cost optimization. By strategically routing complex prompts to AI services like Claude and simpler ones to GPT through multi-agent logic, institutions have efficiently managed inference costs while preserving high accuracy.
Looking Ahead: The Future of Data-Driven Healthcare
As digital transformation in healthcare accelerates, the blend of churn analytics and interactive dashboards is set to redefine operational strategies. Drawing on lessons from both successful and challenging AI projects, providers are better equipped to engage tools that deliver granular, actionable insights. The forecast points to an era dominated by integrated, data-centric approaches that enhance patient care while streamlining operational workflows.
Glossary of Key Terms
- Churn Detection
- A method for identifying patients at risk of disengagement.
- Segmentation Workflows
- Processes used to divide patient data into actionable groups for tailored interventions.
- Regression Models
- Statistical models used to predict patient behavior by analyzing historical data.