“In São Paulo, churn spikes 3x during Q4 due to logistics volatility.”
Abstract
This scholarly article examines the synergy between regional market analysis, innovative churn detection, and AI-driven risk scoring. Through a data-driven lens and empirical evidence, it outlines methodologies that enable precise risk assessment and customer retention strategies aligned with regional dynamics. Detailed analysis and technical research offer actionable insights that harmonize predictive analytics with strategic business adjustments.
Regional Market Dynamics
Regions are defined not just by geography but by unique market behaviors and economic signals. Research indicates that these regional specificities significantly influence customer churn. By analyzing signals from diverse areas—including telecommunications and SaaS industries—companies have achieved heightened precision in targeting critical zones with tailored retention strategies.
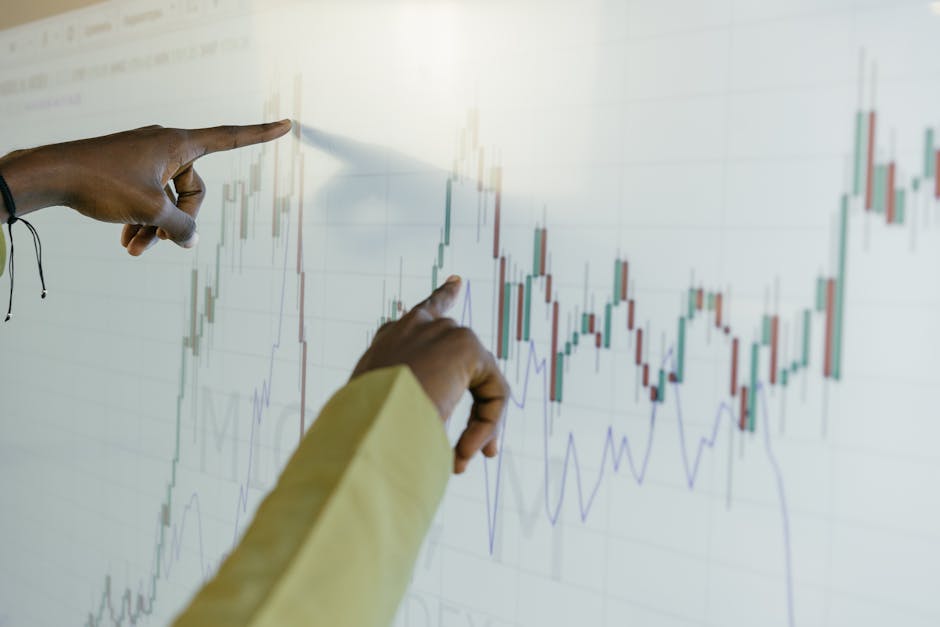
- Regional Market Dynamics
- The distinct behaviors and signals of geographical regions play a crucial role in customer churn. By understanding these dynamics, companies can better target and retain customers.
- Data-Driven Insights
- Integrating quantitative metrics with qualitative insights offers a comprehensive understanding of churn that adapts to dynamic markets.
Innovative Churn Detection Methodologies
Drawing from industry exemplars, advanced churn detection leverages statistical techniques supplemented by machine learning. In sectors like telecommunications, historical data trends have proven that predictive models—and continuous model tuning—can significantly reduce churn. Automation workflows further refine these analyses by providing real-time feedback.
- Innovative Churn Detection
- Advanced methodologies demonstrate the power of targeted churn detection, especially when integrated with regional market signals.
AI-Enabled Risk Scoring and Dynamic Region Prioritization
Advanced AI technologies have reshaped risk scoring, making it dynamic and proactive. By integrating machine learning, companies can continuously update risk profiles and adjust region-specific thresholds. This near-real-time calibration is especially valuable in identifying vulnerable markets immediately, thereby reducing churn significantly.
- AI-Enabled Risk Scoring
- Utilizes machine learning models to transform risk scoring into a dynamic tool that anticipates regional market vulnerabilities.
- Localized Churn Triggers
- Data indicates that region-specific factors—sometimes unseen in broader analyses—can be crucial in understanding and mitigating churn.
Data-Driven Framework and Analytical Insights
A balanced blend of quantitative metrics and qualitative insights provides the backbone for adaptive risk scoring models. Modern analytical platforms, including real-time dashboards and scripting tools, play a key role in evaluating complex regional data sets. In one instance, integrated iterative feedback led to a precise recalibration of risk and churn predictions, thereby optimizing operational strategies.
For those skeptical about abstract AI theories, it's useful to note: I rewired a bot that previously hallucinated product SKUs to use hybrid ranking over metadata-tagged embeddings in Pinecone—a clear demonstration of how precise calibration yields accuracy.
Comparative Regional Analysis: Churn Factors and Mitigation Strategies
Churn Driving Factors | Mitigation Strategies |
---|---|
Regional Market Dynamics | Targeted regional insights to enhance precision in retention strategies |
Lack of Advanced Detection | Implementing cutting-edge churn detection and predictive models |
Static Risk Scoring | Using AI for dynamic, real-time risk scoring and prioritization |
Delayed Data Integration | Leveraging real-time dashboards with integrated scripting tools |
Considerations: Continuous model tuning, near-real-time data integration, region-specific analytics, AI-driven risk reassessment. |
Case Studies and Future Directions
Historical case studies illustrate measurable declines in churn when companies integrate statistical techniques with machine learning. In practice, blending quantitative data with localized qualitative insights has revealed early warning signs, facilitating more agile resource allocation. As analytical methodologies evolve, businesses continue to refine their strategies, continuously reducing churn and enhancing operational efficiency.
The future of regionally tailored risk assessment lies in adaptive, AI-powered models that account for the nuances of local market dynamics. Emerging trends promise even more precise calibrations, ensuring that businesses stay ahead of market shifts.
In our continuous pursuit of excellence, we integrate lessons learned from a variety of case studies and leverage dynamic AI tools to enhance reliability and accuracy in risk management.
Conclusion
An integrated approach that combines regional market analysis, innovative churn detection, and AI-enabled risk scoring paves the way for transformative operational strategies. Embracing data-supported, dynamic models not only anticipates market shifts more effectively but also empowers businesses to optimize their retention and risk management efforts. As the field evolves, the continuous interplay between advanced analytics and localized insights remains critical for sustained success.
Ultimately, understanding and acting on regional signals will drive better decision-making and improved customer retention, marking a significant advancement in both strategy and technology.