Optimizing Input Precision
The discussion begins by asserting that accurate data input is essential in high-stakes industries. Precision in forms and invoice data dramatically alters strategic outcomes. Drawing inspiration from success narratives of companies like SAP and MetaSource, the narrative outlines how automation and finely tuned AI integrations have transformed accounts payable work—reducing error margins and streamlining processes, even in remote and hybrid work environments.
Diagnosing Early Challenges
Organizations traditionally face pitfalls such as uncontrolled tool outputs, misaligned metrics across teams, and unchecked dashboards. Historical industry trends, especially in insurance, have shown that ignoring these challenges leads to delayed insights and misdirected strategies. Insights from cross-functional review meetings have exposed discrepancies in AI outputs, urging teams to address these blind spots head-on.
Navigating Input Complexities
The conversion of form inputs into actionable data is complex. As detailed by Stampli's research, even advanced systems suffer when manual intervention or deficient validation is present. Implementing a dual-layer validation model—combining automated reporting with periodic human audits—has proved essential in resolving misalignments and safeguarding timely data insights.
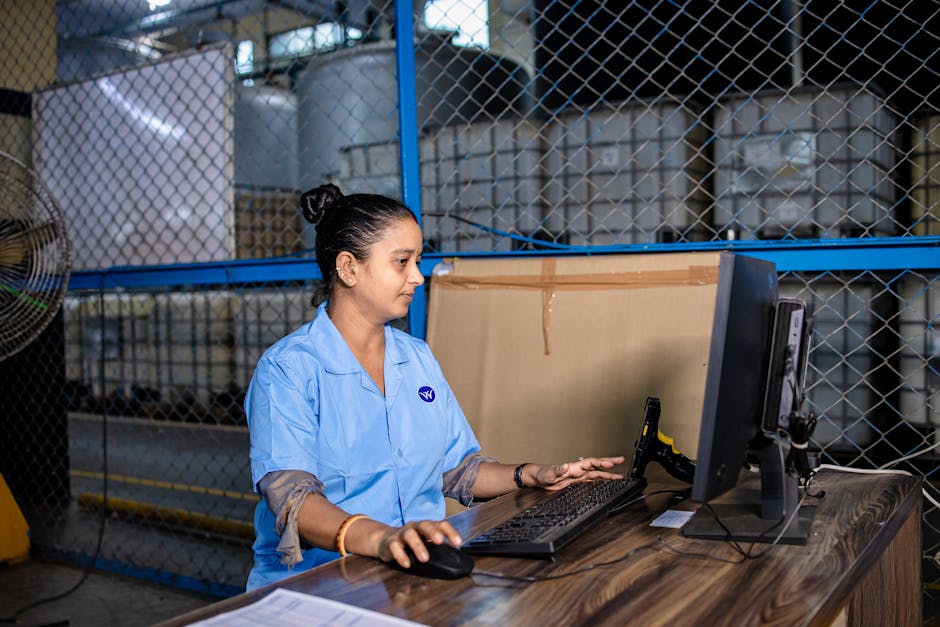
Manual vs. AI-Enhanced Input Workflows
Criteria | Manual Workflow | AI-Enhanced Workflow |
---|---|---|
Accuracy | Variable, prone to human error | High, due to constant calibration |
Speed | Slow; limited by human processing | Fast; near real-time processing |
Oversight | Manual audits required | Dual-layer with automated alerts and human reviews |
Scalability | Limited scalability | Easily scalable with iterative learning loops |
Keywords: form input augmentation, automated reconciliation, reinforcement learning loops |
Industry-Specific Definitions
- automated reconciliation
- An AI-driven process that continuously matches data inputs with corresponding financial records to ensure accuracy.
- submission triage
- A mechanism that evaluates data submissions for priority and correctness, ensuring critical invoices are processed first.
Enhancing Invoice Precision
Embracing AI-Driven Solutions
AI plays a pivotal role in refining invoice processes through systems like reinforcement learning loops. Platforms such as Microsoft Copilot show considerable improvements by continuously fine-tuning output accuracy based on feedback—though these systems require iterative adjustments post-deployment. This approach has become a strategic asset for companies combating invoice inaccuracies.
Real-World Case Studies
Case studies from major insurers reveal that after recalibrating invoice processing via rigorous automation guidelines, issues like metrics drift could be effectively managed. Pre-set thresholds and alerts allow teams to adjust algorithms in response to evolving risk profiles, a practice solidified during monthly cross-team calibration sessions.
Measuring Success Through Technology
Success depends on a robust framework that blends automated reporting with hands-on human oversight. This approach transforms theoretical benefits into measurable performance gains—ensuring the actionable data drives strategic outcomes. The guidelines recommended by SAP and MetaSource serve as benchmarks in ensuring precision in invoice evaluation and process orchestration.
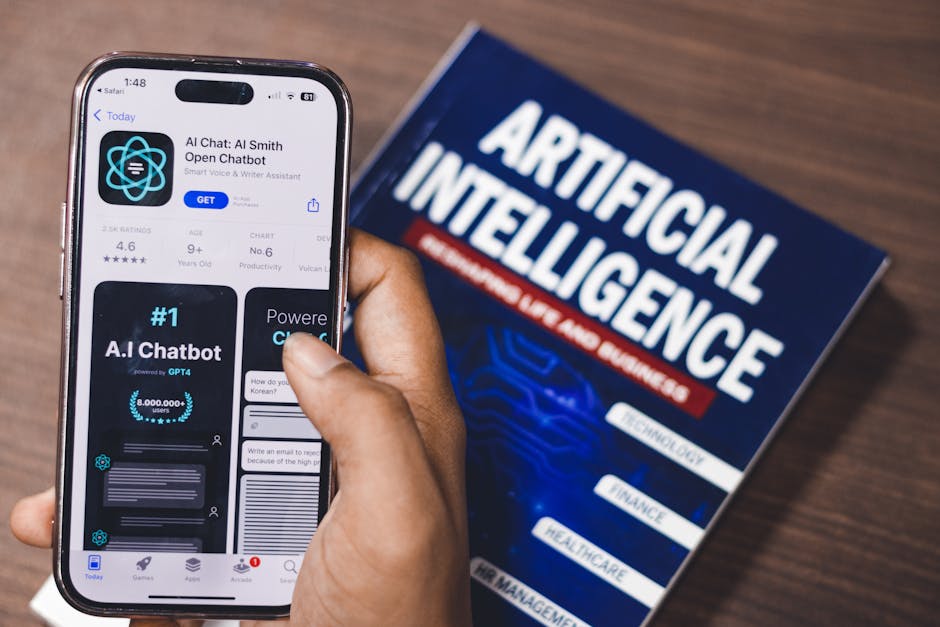
Comparing Manual vs. AI-Enhanced Invoice Precisions
Aspect | Traditional Process | AI-Enhanced Process |
---|---|---|
Overall Accuracy | Suffers from human error and inconsistencies | Higher accuracy due to continuous improvement |
Response Time | Delayed, impacting financial decisions | Timely responses and real-time adjustments |
Visibility | Low; requires manual dashboard reviews | Enhanced with automated alerts and predefined thresholds |
Efficiency | Time-consuming and resource-intensive | Optimized through combination of advanced algorithms and auditing loops |
Keywords: invoice parsing, premium leakage, reinforcement learning loops, continuous monitoring |
Ongoing Oversight and Continuous Improvement
Effective invoice processing isn’t static. The guide emphasizes that continuous oversight—through regular audits, dual-layer validation, and cross-functional review meetings—is necessary to maintain precision. Real-time data monitoring and periodic system tweaks are the cornerstones of sustaining a resilient AI-backed process.
Conclusion and Next Steps
In summary, mastering both data input and invoice processing is not just about leveraging advanced AI technology. It's a strategic initiative that requires careful implementation, constant oversight, and iterative learning. By challenging legacy methods, embracing real-time data insights, and employing AI solutions, organizations can drive measurable improvements and foster a long-term legacy of precision suitable for a remote and hybrid work era.
Remember: the journey towards precision in RevOps is an ongoing cycle of validation, calibration, and innovation. Adopt these strategies, and watch your operations transform.
Industry-Specific Definitions Continued
- premium leakage
- The inadvertent loss of revenue due to discrepancies in data processing, often mitigated by enhanced automation and continuous oversight.