In the ever-evolving landscape of remote and hybrid workplaces, mastering forecast adjustment strategies goes beyond mere adaptation—it's about integrating insights and local expertise to meet global demands. Dive into this comprehensive guide designed for e-commerce operations directors, seasoned by real-world examples and strategic revelations.
Key Terminology
- Phantom Demand
- A situation where perceived demand is higher than actual customer interest, often due to erroneous data interpretation.
- DC Lag
- Delay in distribution center operations that causes discrepancies between current supply capabilities and inventory requirements.
Overview Of Strategic Areas
Section | Description |
---|---|
Recognizing the Need for Strategic Adjustments | Emphasizes the transition from historical data dependency to proactive demand management, illustrated by Walmart and Amazon's practices. |
Building a Real-Time Data Analysis Framework | Explores constructing a dynamic data infrastructure with decentralized sources, promoting the integration of OpenAI-driven analytics. |
Implementing Forecast Adjustment Strategies with Practical Scenarios | Highlights actionable techniques and case studies for refining inventory forecasts, drawing on sector-specific challenges. |
Interactive Elements: Worksheets, Checklists, and Process Blueprints | Introduces tools like checklists and worksheets to bridge theory and practice, alongside examples relevant to fragmented data scenarios. |
Evaluating Impact and Scaling Future Opportunities | Presents methods for assessing forecast adjustments' success and integrating emerging machine learning models for scalability and refinement. |
Consideration Keywords: cost_forecast_tweaks, attrition_signal_detection, forecast_adjustments, classification_models, rag_for_internal_docs, kpi_projection_modeling. |
Step 1: Recognizing the Need for Strategic Adjustments
Embark on understanding the necessity of evolving beyond a heavy reliance on historical data. Leading industries like Walmart and Amazon have demonstrated that operational success depends on identifying when existing models no longer capture consumer behaviors effectively. By monitoring key performance indicators and observing even the smallest drift in inventory levels, operational leaders can swiftly adapt to changes, ensuring that supply closely follows fluctuating consumer demand.
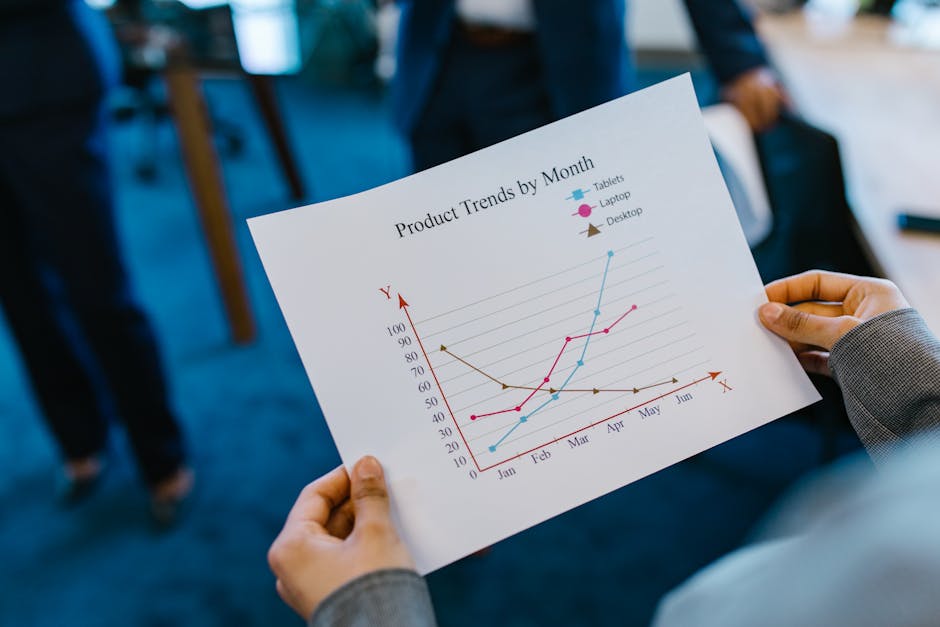
Step 2: Building a Real-Time Data Analysis Framework
A robust data analysis framework is essential to bridge decentralized sources with centralized analytics. By integrating team-specific dashboards and OpenAI-driven systems, leaders can monitor granular KPIs and swiftly detect emerging attrition signals. This modern approach allows for dynamically adjusting forecasts in accordance with real-time market developments. The flexibility of this layered approach is particularly valuable for organizations managing decentralized tech stacks and complex inventory systems.
More on Building a Framework
An efficient framework collects detailed inputs from regional operations and combines them with historical trends to forecast demand accurately. Techniques include setting up automated data pipelines, creating alert systems for crucial performance changes, and using machine learning models to refine predictions.
Step 3: Implementing Forecast Adjustment Strategies with Practical Scenarios
Put theory into practice by employing actionable techniques to fine-tune inventory forecasts. By simulating forecast scenarios—such as seasonal trends or localized demand surges—you gain the edge in anticipating adjustments before they impact overall operations. For example, a simulation model might incorporate granular weekly data cascaded from regional hubs, allowing immediate response to unexpected market fluctuations. This results in sharper alignment between supply and dynamic consumer demands.
How do you immediately know when to adjust your forecasts? Leaders use simulation models that integrate real-time market shifts with historical data, ensuring that even subtle KPI drifts are detected and addressed.
Step 4: Interactive Tools for Bridging Theory and Practice
Translate strategic insights into on-the-ground actions with interactive resources. Worksheets, checklists, and process blueprints enable teams to document forecast scenarios and operational responses, serving as practical tools during cross-functional meetings. These elements are especially useful in environments where reminders are set manually and internal logic systems may be decentralized.
View Sample Checklist
A sample checklist might include items like verifying data inputs, cross-checking regional trends, and confirming alerts from machine learning systems. Each step acts as a safeguard against potential misalignments in forecast accuracy.
Step 5: Evaluating Impact and Scaling Future Opportunities
The final step involves continuous monitoring and evaluation of forecast adjustments. Advanced techniques, including real-time impact assessments and iterative scaling using machine learning models, help refine operational strategies over time. This systematic evaluation paves the way for pioneering improvements, ensuring that operational efficiency evolves alongside market demands.
By consistently reviewing outcomes and integrating emergent technologies, operations directors can transform legacy processes into agile, data-driven models that are both resilient and scalable.