Setting the Stage for AI-Driven SOP Innovations
Industries ranging from manufacturing to healthcare are reinventing their standard operating procedures with AI integration. Trendsetting enterprises such as Google Cloud are leveraging generative AI to boost operational efficiency, while innovators at IBM are re-engineering manufacturing strategies. Organizations are now blending human expertise with cutting-edge technology to dismantle old silos and drive new levels of operational harmony.
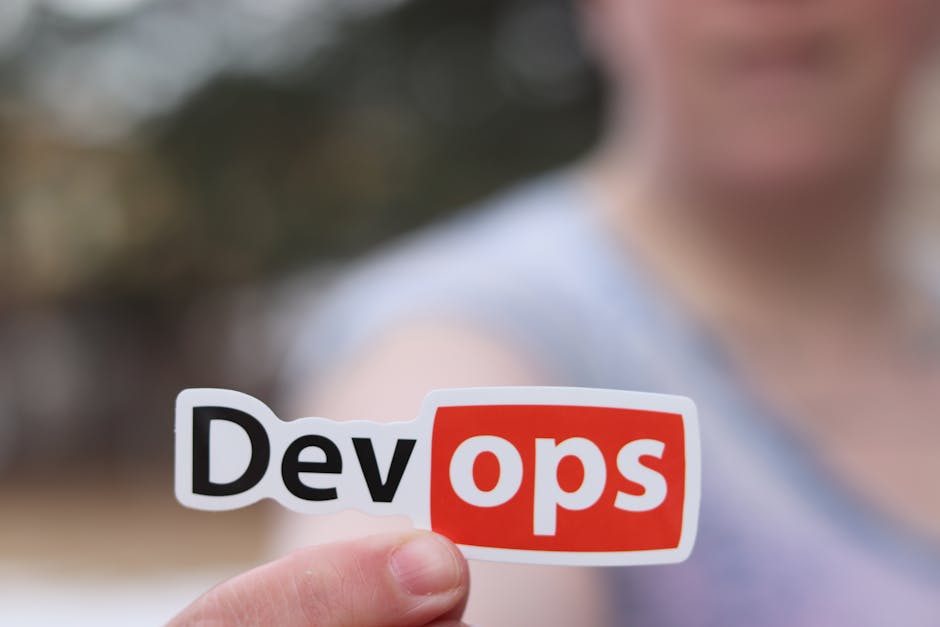
Learning from the Frontlines of SOP and AI Applications
Case studies from trailblazers like IBM and progressive healthcare organizations reveal how AI is seamlessly embedded into SOP architectures. Hospital teams, for example, have successfully used AI tools to enhance patient flow predictions and optimize staff allocations. These examples underscore the importance of restructuring workflows—similar to IBM's manufacturing revamps—to achieve consistent forecasting and reporting.
Navigating Forecast Accuracy Challenges with Tactical Precision
Forecast accuracy remains a pivotal challenge, often complicated by overlapping roles and inconsistent platform logics. Advanced forecasting systems built by major technology players demonstrate that algorithmic fine-tuning can significantly enhance accuracy. This discussion is anchored by key metrics such as Mean Absolute Percentage Error (MAPE) and Continuous Ranked Probability Score (CRPS), which provide quantifiable benchmarks for verifying AI outcomes.
- Forecasting Metrics:
- These metrics bridge AI insights with solid reporting results, helping teams pinpoint areas for improvement and refine their predictive capabilities.
By embracing dynamic feedback loops and methodical clarity, organizations can peel away the "black box" mystique and restore transparency to data-driven forecasts.
Identifying and Overcoming AI Production Pitfalls
The AI production journey is not without obstacles. Challenges such as managing data silos and mitigating model drift are commonplace. A notable example is Meta's Llama initiative, which illustrates the consequences of deploying a model without thorough operational verification. This cautionary tale reinforces the need for rigorous testing and evolving protocols.
- Model Drift Solutions:
- Employing ensemble techniques and transfer learning has proven effective in countering model drift, thereby ensuring sustained forecast precision in complex operations.
By drawing on proven strategies and a culture of continuous improvement, organizations can adeptly navigate the pitfalls of AI deployment.
Actionable Strategies to Empower Change
The following table outlines pragmatic steps to infuse your operations with AI-driven innovations, inspired by best practices from industry leaders:
Strategy | Action |
---|---|
Audit and Revamp SOPs | Emulate transformative projects at Google and IBM to drive SOP innovation. |
Facilitate Cross-Functional Reviews | Evaluate current forecasting methodologies and institute continuous feedback loops. |
Pilot AI Solutions | Implement controlled trials to ensure robust AI deployments without rushed integration. |
Adapt Forecasting Metrics Regularly | Continuously adjust metrics to align with measurable business outcomes. |
Consider regular reviews, feedback incorporation, and agile adjustments for a resilient and data-driven SOP evolution. Keywords: forecast adjustments, SOP generation, why genai fails in production, in house vs vendor ai. |
Additionally, a case snippet influenced by GitOps principles highlights the potential of under-the-radar tools to refine SOP workflows and enhance cross-disciplinary cooperation. This snippet stands as a reminder that realistic AI deployments, supported by stringent trials and feedback loops, can catalyze significant operational improvements.