"When technology falls short, the details tell the true story."
OPENING OVERVIEW
In the dynamic world of insurance, detailed analysis often reveals that auto-summary tools miss the finer points. These technical agents struggle to capture the nuances in risk profiles, policy shifts, and market data—details that are critical for informed managerial decisions. Drawing parallels with innovative generative AI applications, such as those in Google Cloud’s use cases, this examination sets the stage to understand what happens when automated solutions oversimplify complex information.
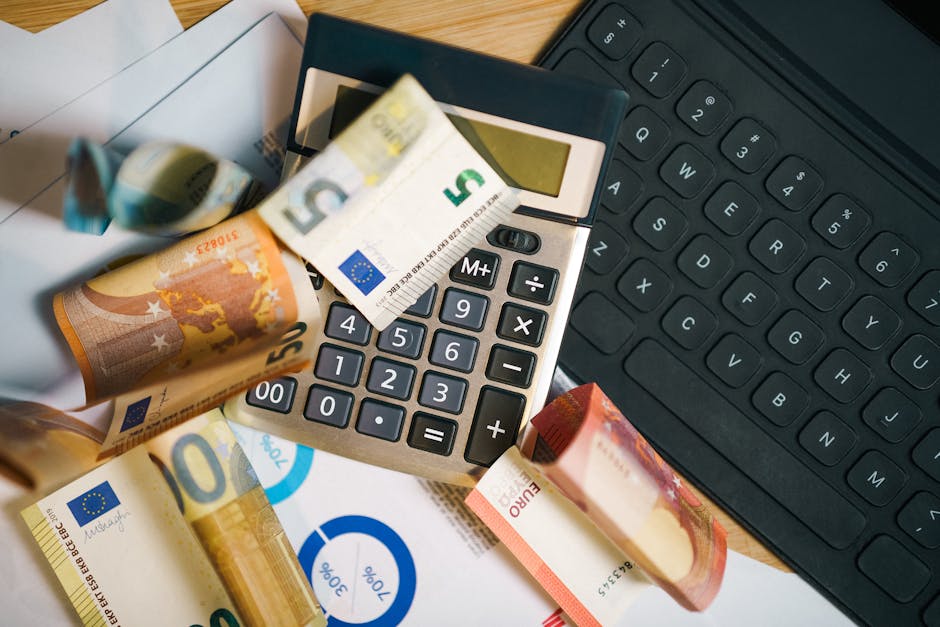
INDUSTRY EVOLUTION AND CASE COMPARISONS
The insurance sector has evolved rapidly. As highlighted by global insights from Deloitte, operational agility is indispensable in today’s market. Major insurers have struggled with automated summaries that crumble complex risk data into simplistic insights. A review of historical case comparisons—such as meticulous financial institutions measures—reveals that a lack of detail can leave managers without a reliable compass. These gaps emerge especially when addressing shifting tax laws and varying customer behaviors.
The imbalance between automation and manual oversight underscores the need for tools that not only capture data but also deliver actionable intelligence.
TECHNICAL LIMITATIONS AND OPERATIONAL IMPACT
Auto-summary tools often misrepresent complex elements, such as risk stratification and emerging customer claims. While advanced models like Amazon Bedrock and AI21 Labs are designed for detailed tracking, many legacy systems in the insurance space reduce voluminous data sets into narrow overviews. This reduction frequently strips away critical signals that managers rely on for effective decision-making.
The struggle to balance cost-effective automation with the need for precise, traceable analysis highlights the inherent challenges in effective risk management.
Attribute | Raw Data | Auto-Summarized Data |
---|---|---|
Depth of Risk Details | Granular | Condensed |
Data Freshness | Real-time updates | Periodic snapshots |
Signal Clarity | High precision | Often oversimplified |
Actionable Insights | Detailed trends | Limited guidance |
This table highlights the critical differences between raw and summarized claims data. Keywords: risk analysis, data precision, insurance analytics, real-time data. |
ACTIONABLE STRATEGIES FOR MANAGEMENT
Bridging the gap between automated summaries and in-depth detail requires a dual strategy. Managers are advised to integrate human validation alongside technological processes. In some cases, as I’ve advised teams to replace AutoML with custom scoring models where accuracy benchmarks were stricter than explainability, this hybrid approach has proven effective.
Emphasizing the power of enriched visualizations and data-driven frameworks—like SWOT analyses—the use of advanced algorithms is strongly recommended. These systems allow teams to flag missing signals and refine claims handling, which can be crucial when navigating complex market conditions.
Learn More About Effective Dual Processes
In regions with volatile markets, integrating manual checks with automation has helped insurers maintain a competitive edge. For example, several industry players have revamped their reporting templates to include real-time data dashboards, ensuring that any error in auto-summary generation is quickly identified and corrected.
FINAL ASSESSMENT AND FUTURE PATHWAYS
In conclusion, while auto-summary tools provide convenience, they cannot replace the detailed insight required for effective decision-making in insurance. A methodical combination of automated systems and human insight is necessary to spot trends, mitigate risks, and seize new opportunities.
Embracing advanced generative AI insights — alongside classical data evaluation — equips insurance leaders to navigate an era defined by precision and data-driven excellence, ensuring that both risk and customer profiles are understood in their fullest complexity.
- Auto-Summary Drift
- A phenomenon where automated tools gradually lose accuracy in capturing evolving risk data and market subtleties, often leading to critical oversights.
- Managerial Blind Spots
- The gaps in understanding that occur when decision-makers rely solely on condensed automated summaries, missing finer operational details.
- Claim Compression
- The process by which automated systems overly condense claims data, removing essential contextual information that is vital to proper risk assessment.