An Unexpected Spark
Nestled within a lively federal office, seasoned analysts recall the early skepticism that surrounded artificial intelligence. Amid the hum of automated dashboards and the routine update of weekly reports, an anomaly in a reporting tool ignited a debate: Was this machine truly intelligent, or was it simply executing a complex algorithm? This moment evokes memories of computing's early days and underscores the long-standing challenge of understanding AI's intricate nuances.
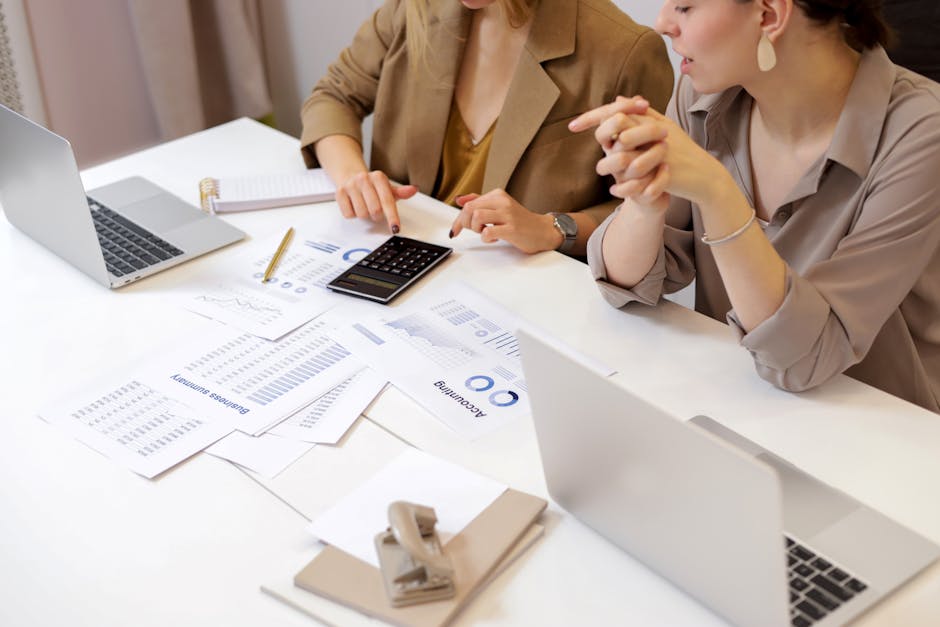
Decoding Skepticism in AI
History shows that skepticism is not merely a barrier but a vital dialogue partner. Just as recalibrated dashboards can reveal hidden metrics, examining AI's underlying logic layers—similar to transparency initiatives seen with major platforms like Amazon Bedrock—uncovers that many concerns arise from human bias and misinterpretations of complex algorithms. This deep dive into AI skepticism highlights the need for open, data-driven discussions in adapting to new technologies.
- AI Skepticism
- An ongoing scrutiny where emotional bias and misinterpretation intersect with genuine caution regarding emergent technologies.
- Model Transparency
- A commitment by AI providers to reveal inner mechanisms, much like well-calibrated dashboards provide clear insights into complex processes.
Transformations and Lessons from Information Systems
AI’s influence in sectors like healthcare and information systems has proven transformative. Radiology, for instance, has seen dramatic improvements in speed and precision with the integration of automated imaging, as highlighted by studies from the National Center for Biotechnology Information. Similarly, the integration of sophisticated algorithms into government dashboards optimizes report generation and detects attrition signals. These developments not only reshape operational landscapes but also turn skepticism into practical, data-driven utility.
- Alignment Drift
- This occurs when the outputs of an AI system slowly diverge from its intended behavior, necessitating regular recalibrations.
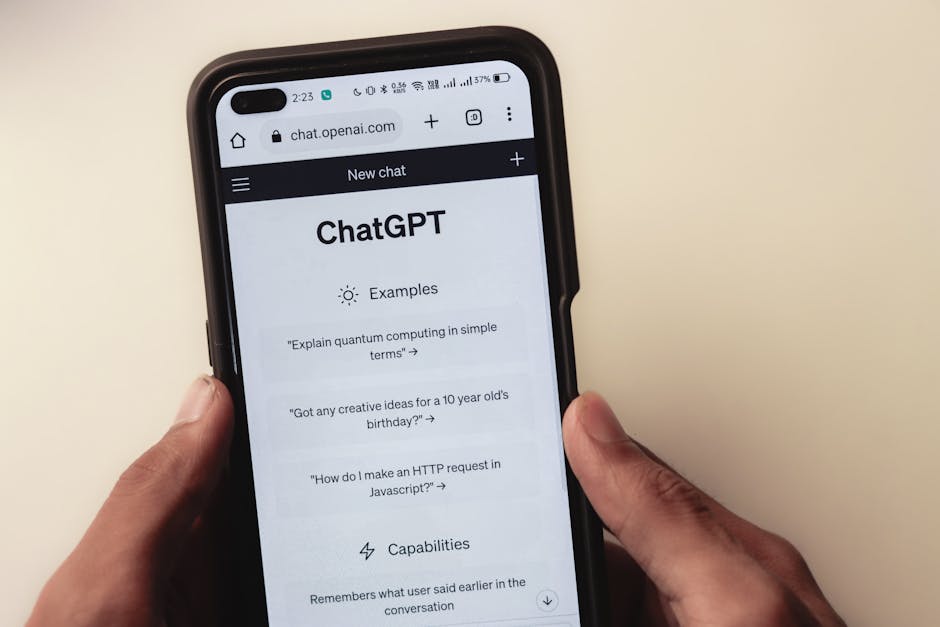
The ChatGPT Paradigm and Real-World Implications
Evolution in conversational models, epitomized by OpenAI's ChatGPT, demonstrates a clear journey from technological novelty to an indispensable tool in various sectors—ranging from customer support to policy formulation. This evolution is accompanied by a balanced approach addressing ethical concerns, inherent biases, and the call for transparency. In parallel, the real-world implications of AI in high-stakes settings, such as healthcare and digital governance, demand that every automated dashboard and decision support tool reflects both empowerment and cautious oversight.

Explore in-depth data comparisons
Year | Public Skepticism Level | Measured AI Performance | Dashboard Insights |
---|---|---|---|
2015 | High | Emerging | Basic Metrics |
2017 | Moderate | Improving | Enhanced Analytics |
2019 | Reduced | Robust | Predictive Dashboards |
2022 | Balanced | Advanced | Integrated Intelligence |
Note: Data reflects evolving sentiments and performance improvements. Search terms: "AI skepticism", "dashboard analytics", "public vs actual AI". |
A Call to Deeper Exploration
The narrative invites readers to embrace AI's potential while balancing celebrated advancements with a healthy dose of skepticism. Rather than unbridled optimism, the pace of progress calls for measured exploration, where every technological breakthrough guides us toward a more informed future. Each step in this journey—from early doubts to educated empowerment—offers an opportunity to reshape internal tools and operational insights.
Knowledge tidbits & historical insights: Consider the evolution of skepticism in government computing from early dashboard misinterpretations to today’s algorithmic transparency. Iterative audits and multi-layered checks, as endorsed by NIST's trustworthy AI guidelines, continue to ground AI discussions in practical reality.
This blog provides a window into navigating AI’s ever-evolving landscape, drawing connections between skepticism and understanding, and reinforcing the importance of data-backed decision-making and internal tool optimization.