Boosting Forecast Accuracy: Techniques & Insights
Enhancing Sales Calls by Detecting Early Attrition Signals
In today’s dynamic retail landscape, blending cutting‐edge technology with insightful data analysis has become crucial. Innovative companies such as Walmart and Target are leading the way in adapting forecast models that capture key attrition signals, ensuring their sales strategies remain both agile and accurate.
- attrition signals
- Early indicators that suggest a potential decline in customer engagement or loyalty, often identified through sales call data and feedback metrics.
- forecast decay
- The gradual divergence between projected outcomes and actual results due to external market or internal process changes.
Overcoming Data Inconsistencies
Many organizations face challenges due to disparate manual processes and decentralized key performance indicator (KPI) definitions. Legacy systems often lead to misalignment between forecast models and real-world outcomes. Consolidating these elements onto centralized KPI dashboards can improve consistency and data integrity—transforming scattered data points into actionable insights.
By streamlining data sources, companies are able to optimize their sales call strategies, ultimately reducing missed opportunities and improving overall forecasting performance.
Data-Driven Insights & Enhanced Techniques
Forecasting is not merely about prediction; it is a strategic process that leverages analytical models to detect subtle patterns in consumer behavior. Advanced techniques, including regression analysis and time-series forecasting, have identified early attrition signals that allow teams to pivot quickly and optimize forecast accuracy.
Real-time alerts—replacing outdated manual triggers—have proven to refine sales call strategies. For example, enhancements implemented by platforms like Cirrus Insight demonstrate significant uplift in sales outcomes when AI-driven insights are integrated.
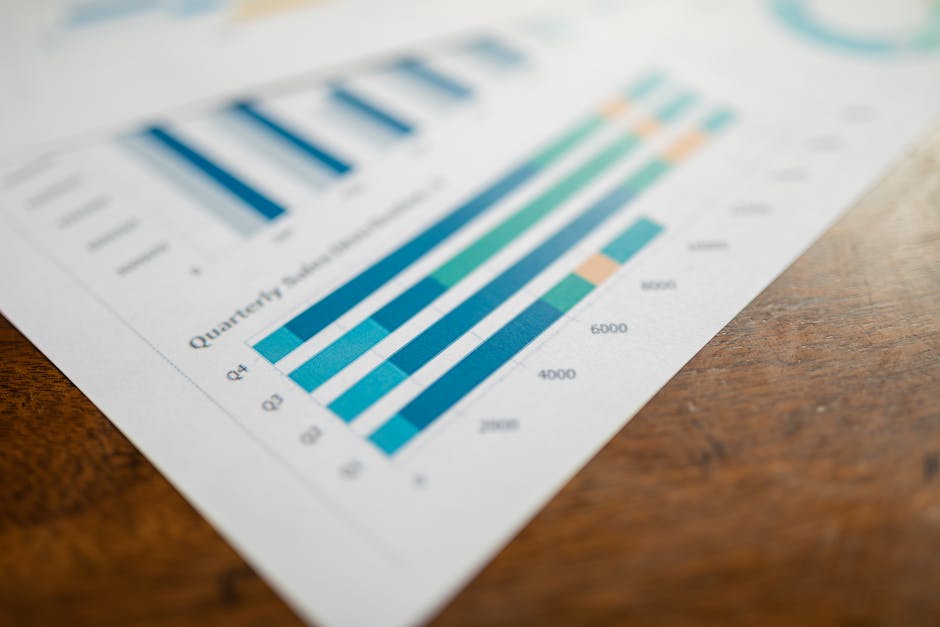
Metric | Pre-Implementation | Post-Implementation |
---|---|---|
Average Talk Time | 12 minutes | 15 minutes |
Objection Rate | 20% | 12% |
Conversion Rate | 30% | 38% |
Response Time | 5 hours | 2 hours |
Note: Figures are based on aggregated data across multiple retail scenarios. Keywords: sales call metrics, forecasting models, AI integration. |
Leveraging External Economic Insights
Incorporating external economic indicators can boost forecast accuracy by as much as 10–15%. With budget constraints and decentralized data practices, iterative model updates become crucial. Even simple feedback loops that prompt model recalibration can dramatically reduce forecast errors—saving both time and resources.
This approach allows analysts to navigate operational challenges while maintaining a competitive edge despite fluctuating market conditions.
In Summary
As market dynamics continue to evolve, it is clear that traditional forecasting methods require an upgrade. By adopting advanced analytical techniques and integrating real-time data signals, sales call strategies can be realigned to meet modern challenges. Embracing a data-driven approach not only reduces forecast decay, but also enhances overall sales performance—turning potential setbacks into strategic advantages.
This shift is essential for organizations determined to stay ahead in a competitive retail environment, where precision in forecasting translates directly into improved outcomes and sustained operational excellence.