Laying the Groundwork for Precision in Data Migrations
Data migrations form the backbone of agile and robust customer complaint processes. Industry giants like eBay and Alibaba have shown that even minor data discrepancies can snowball into significant delays. By carefully aligning technical data extraction with customer communication flows, teams can develop a migration strategy that quickly and accurately resolves complaint backlogs.
The initial stage focuses on creating a precision-driven foundation, setting clear expectations for how data should be handled before it becomes part of the complaint resolution engine.
- Step 1: Identify Data Sources
- Map out all essential data repositories to ensure comprehensive coverage of customer information.
- Step 2: Implement Automated PDF-to-SQL Conversion
- Utilize automated tools to convert PDFs into SQL databases to maintain data integrity and speed up processing.
- Step 3: Validate Through Real-Time Synchronization
- Set up mechanisms for continuous data validation to quickly catch and correct any discrepancies.
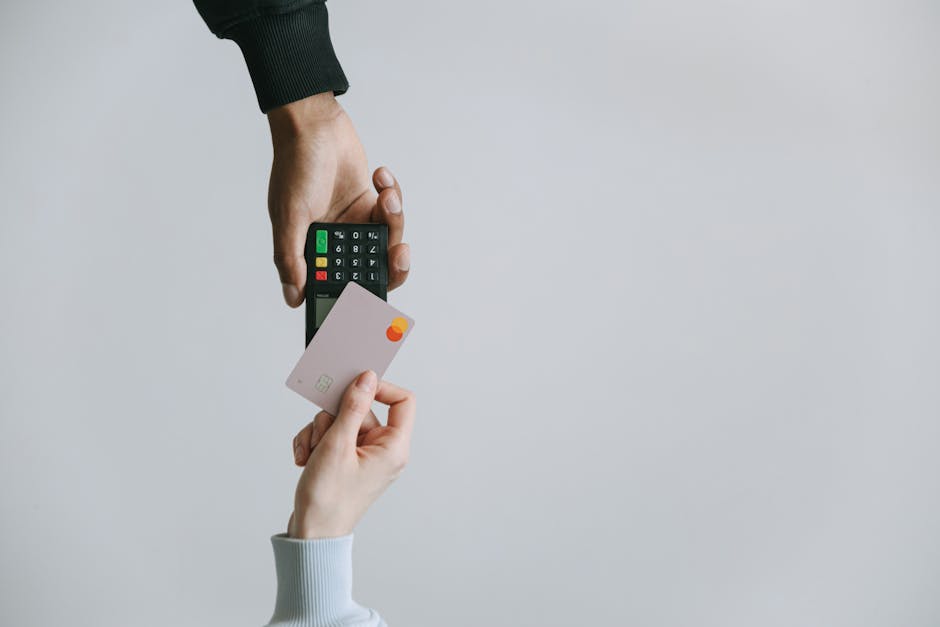
Crafting a Blueprint for Speed and Accuracy
A two-pronged approach is essential to merge the technical rigor of PDF-to-SQL migrations with automated insights from industry leaders such as Docsumo. This blueprint emphasizes precise data extraction, rigorous validation, and real-time synchronization with customer complaint systems.
Advanced techniques, including empirical reinforcement learning loops, are now available to catch data anomalies early, ensuring that the migration process is not only fast but also accurate.
- Step 1: Execute Precise Data Extraction
- Focus on extracting data with pinpoint accuracy to prevent errors downstream in the process.
- Step 2: Conduct Rigorous Validation Checks
- Incorporate multiple layers of validation to safely verify the data before it is used in decision-making.
- Step 3: Synchronize in Real-Time with Customer Systems
- Ensure that customer complaint data is immediately updated across platforms, maintaining consistency and reliability.
Expert Strategies and Technical Insights from Industry Pioneers
Leaders in the artificial intelligence and cloud industries, including Dataiku and Anthropic, advocate for the use of advanced tactics such as cloud-based integrity checks. Leveraging platforms like Google Cloud provides the necessary scalability and precision to ensure high data quality through generative AI solutions.
These expert strategies empower teams to integrate PDF-to-SQL migrations with sophisticated data visualizations, addressing the data quality issues that analysts encounter on a regular basis.
- Step 1: Utilize Cloud-Based AI Solutions
- Adopt cloud systems that enable advanced data integrity checks, ensuring that even the smallest anomalies are detected.
- Step 2: Integrate PDF-to-SQL with Data Visualization
- Create seamless data flows that merge transactional data with visual dashboards for real-time monitoring.
- Step 3: Anticipate Data Quality Issues
- Set up predictive analytics to foresee data inconsistencies and address them proactively.
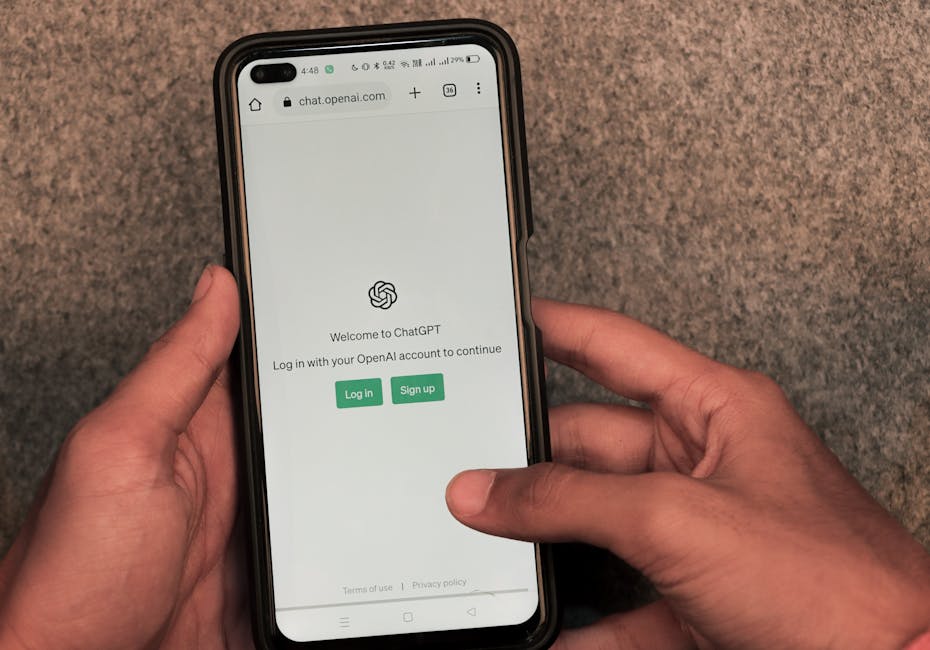
Seizing the Moment – A Call to Transform Data Migrations
It is time to take immediate, decisive action for transforming data migrations. By revisiting and re-evaluating current methods, teams can adopt hybrid technologies that combine rapid execution with robust reliability.
This transformation will not only enhance complaint handling efficiency but also lower response times and significantly improve customer satisfaction metrics. In the fast-paced world of eCommerce, staying ahead of the curve means embracing every opportunity to lead in operational excellence.
- Step 1: Re-Evaluate Current Methods
- Audit the existing data migration processes to identify areas that could benefit from technological upgrades.
- Step 2: Adopt Hybrid Technologies
- Combine traditional data processing techniques with modern automation and AI-driven verification processes.
- Step 3: Continuously Refine Protocols
- Commit to a regular review of procedures and implement improvements that keep pace with technological advancements.
Migration Phases and Key Terms Timeline
Below is a vertical timeline outlining critical phases in data migration journeys. Each phase is anchored by key terms that provide insight into the process, ensuring thorough documentation and quick reference for team members.
- Phase 1: Initiation – Data Integrity Lock
- Lock in data integrity at the onset by enforcing strict validation rules during initial extraction.
- Phase 2: Processing – Latency Bottleneck
- Identify and address latency issues to ensure that data flows efficiently between systems.
- Phase 3: Validation – Automated Speed Checks
- Introduce automated quality checks to sustain high accuracy in the migration pipeline.
- Phase 4: Synchronization – Real-Time Resolution
- Ensure that updated data is in perfect sync with customer systems to enable prompt complaint resolution.
Failure Modes: Pre and Post-Migration Complaint Metrics
Metric | Pre-Migration | Post-Migration | Improvement (%) |
---|---|---|---|
Response Time | 48 hrs | 24 hrs | 50% |
Resolution Accuracy | 80% | 95% | 18.75% |
Data Consistency | 70% | 90% | 28.6% |
Customer Satisfaction | 75% | 88% | 17.3% |
Considerations: Focus on continuous improvement, real-time validations, and AI-driven analytics to maintain operational excellence. Keywords: data integrity, latency, real-time synchronization, and complaint resolution. |
Final Thoughts
By integrating proven strategies and leveraging modern technologies, businesses in the eCommerce sector can transform data migrations into a potent tool for enhancing complaint handling efficiency. With insights drawn from industry pioneers, the path to operational excellence is clear for cross-functional teams ready to embrace change and excel in both speed and reliability.
This approach answers the common question: How can organizations ensure their data migrations not only avoid pitfalls but actively enhance customer satisfaction?