>> anomaly_detected
- SQL Gap Detection
- Utilizes SQL queries to identify discrepancies in data reporting, ensuring accuracy and operational alignment.
- Customer Support Tagging
- Structured labeling of support inquiries enhances resolution speed and service delivery accuracy.
- AI Buy vs. Build Dilemma
- Weighing the decision between developing in-house AI capabilities or partnering with third-party vendors to leverage established technologies.
Comparative Insights
Detected Issue | Resolution Path |
---|---|
SQL Gap Detection | Pinpoints data discrepancies to enhance operational precision. |
Customer Support Tagging | Reduces response time and improves overall service efficiency. |
AI Buy vs. Build Dilemma | Guides a strategic approach for leveraging either proprietary development or vendor partnerships. |
Operational Drift | Identifies misalignments in reporting flows that can be corrected in real-time. |
Insights: These techniques offer a blend of technical precision and strategic flexibility, which is critical in dynamic work environments like nonprofits and tech sectors. Keywords: SQL gap detection, customer support efficiency, AI decision-making. |
Operational Process Timeline
This vertical timeline outlines a series of practical steps demonstrating how operational discrepancies are detected and resolved:
- Initial Detection: A RevOps manager at a nonprofit runs a SQL query to identify a subtle formatting error in a reporting template—similar to troubleshooting at local community centers.
- Tagging Implementation: Customer support tagging is used to label and categorize support inquiries. The technique feeds into automated summary emails, paralleling how local sports teams efficiently manage scheduling updates.
- Strategic Decision-Making: The ensuing discussion on the AI Buy vs. Build dilemma draws from evidence such as benchmarks against platforms like IBM Watson, enhancing decision-making in a competitive landscape.
Real-World Application and Local Anecdotes
In community-driven projects and hybrid workplaces, similar methodologies have been successfully applied. For example, when a local nonprofit restructured its reporting flow, adopting SQL gap detection resolved a recurring data mishap before it escalated—a turnaround reminiscent of rapid problem-solving in bustling community hubs. Structured customer support tagging, on the other hand, has directly improved communication in teams, echoing the quick, precise coordination seen in neighborhood volunteer groups.
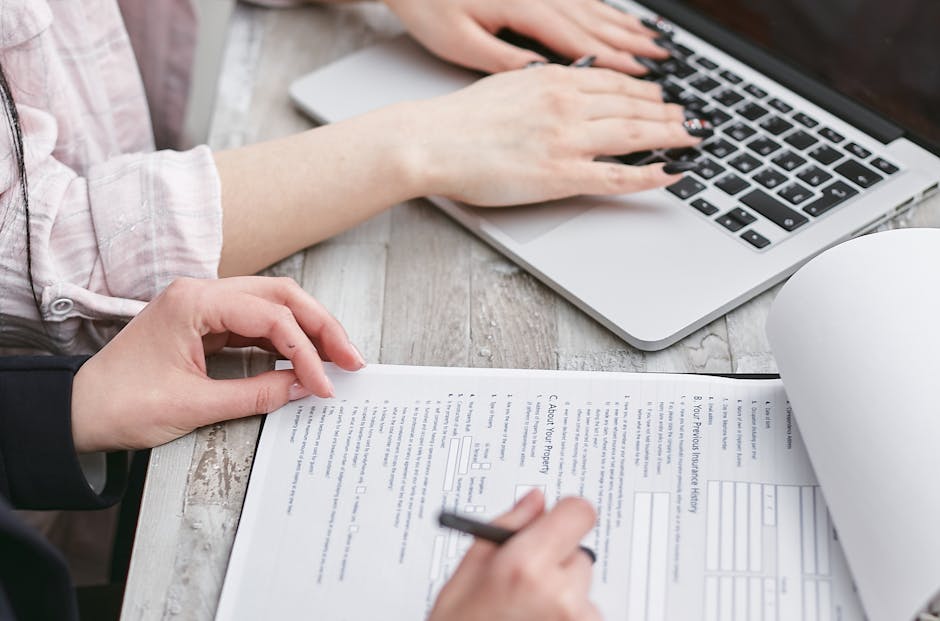
Strategic Recommendations
To optimize operations and customer support efficiency, organizations should consider integrating these practices into their daily workflows. Implementing SQL gap detection minimizes unnoticed discrepancies, while structured support tagging increases resolution speed. Moreover, addressing the AI Buy vs. Build dilemma empowers decision-makers to balance innovation with cost-efficiency. This guidance is backed by empirical data and local success stories, ensuring that the approach remains both practical and scalable.
Embracing these change mechanisms not only streamlines operations but also builds a resilient infrastructure ready to tackle unexpected challenges—long before they escalate into critical issues.