Introduction & Core Challenges
In today’s fast-paced production world, success hinges on seamless readiness and transparent operations. Many companies, from retail giants to logistics leaders, are increasingly relying on AI models that work like a black box—delivering remarkable efficiencies while concealing their decision processes. This creates both striking opportunities and daunting risks. Industry pioneers have noted that while these models optimize performance, their lack of explainability can lead to unanticipated setbacks.
The Challenge of Opaque Decision-Makers
At the heart of the issue is the concealed logic within these systems. In practice, models that drive efficiency can also hide critical mistakes. Experts have often cited frameworks like IBM's Explainable AI as a way to ensure that decision-making remains understandable and accountable. Without transparency, even well-intentioned deployments might result in volatility across production environments.
- Observability Debt
- The accumulated risk when insufficient monitoring leads to undetected issues in AI processes.
- Shadow Deploys
- Instances where unvetted versions of models are released in parallel to production systems, often increasing risk.
- Telemetry Drift
- The gradual deviation in system metrics that signals emerging inconsistencies in model performance.
Real-World Outcomes & Industry Insights
Case Studies of Production Setbacks and Successes
Across sectors, real-world deployments have yielded mixed results. One multinational e-commerce firm experienced a noticeable revenue dip after an AI pricing misfire, whereas a major supply chain operator saw improvements by integrating explainability tools. Metrics such as order fulfillment and inventory turnover reveal the tangible impact of embracing transparent model practices.
Expert Opinions and Analysis
Industry leaders from organizations like Gartner and IBM stress that a solid understanding of AI algorithms is indispensable for informed decision-making. Publications like Black Box Intelligence have driven home the message: clear and trustworthy models are not a luxury, but a necessity for responsible AI deployment.
Comparative Readiness Signals: Legacy vs. Modern Approaches
Signal Type | Visibility | Actionability |
---|---|---|
Static Logs | Low | Reactive |
Manual Reports | Moderate | Delayed |
Real-Time Metrics | High | Prompt |
Explainability Dashboards | Very High | Immediate |
This table highlights the evolution from legacy methods to advanced, transparent systems that empower rapid intervention and improvements. Keywords: production_ready_nlp, why_black_box_is_bad, just_gimme_the_logic. |
Practical Strategies for Enhanced Transparency
Implementing Explainable AI (XAI)
Practical strategies include adopting models that feature clear, visual explanations of their internal decision processes. A step-by-step implementation of Explainable AI (XAI) not only builds trust in the system, but also provides technical teams with the means to swiftly intervene when irregularities occur.
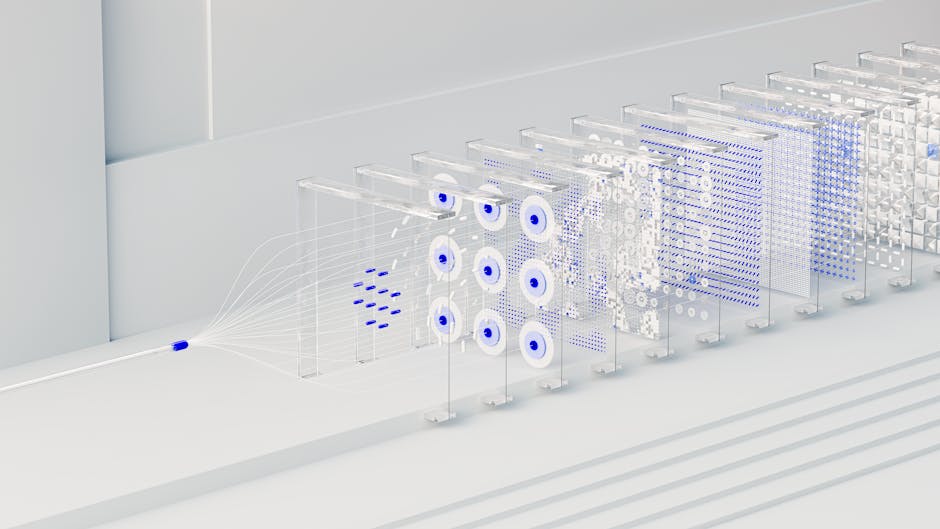
Rigorous Testing and Continuous Improvement
Comprehensive testing regimes, including unit tests, UI assessments, and end-user usability checks, are central to ensuring consistent model performance. Techniques drawn from industry leaders like BrowserStack and Applitools underscore the importance of both functional and stress-testing protocols. The evolution towards continuous feedback loops means that systems can harness real-time data to convert abstract metrics into actionable insights.
Conclusion & Future Engagement
Moving away from the pitfalls of black box systems calls for a strategic overhaul emphasizing transparency and accountability. By learning from past failures and building on expert guidelines, organizations can enhance not only their operational resilience but also foster ongoing innovation in AI. Consider: could improved interpretability be the key to unlocking more reliable production outcomes?
Share your insights and participate in the dialogue on sustainable AI innovations. Your experiences and expert observations could be the catalyst that drives the next wave of transparency in production systems.