- Real-Time Data Analytics
- The process of collecting and processing data as it is generated, allowing for immediate input into decision-making processes.
- Heuristic Logic
- Rule-based methods used to make decisions in systems lacking complete information, often drawing on past experiences and "rules of thumb".
- KPI
- Key Performance Indicator: a measurable value used to evaluate the success of an organization in achieving its objectives.
Insider Terminology
- Cost Drift
- An observable trend where projected costs start diverging from actual expenses over time.
- Utilization Creep
- The gradual increase in resource usage that can lead to higher operating costs if not monitored closely.
- Predictive Burn Rate
- A metric that estimates the pace at which available budget is being consumed based on current trends.
Introduction
In an era marked by escalating healthcare costs, the necessity for precise financial forecasting has never been greater. Recent advancements in real-time data analytics are transforming the forecasting landscape, offering enhanced accuracy and adaptability. This article explores how sophisticated forecasting models, underpinned by dynamic data streams, are essential in managing healthcare operational costs effectively.
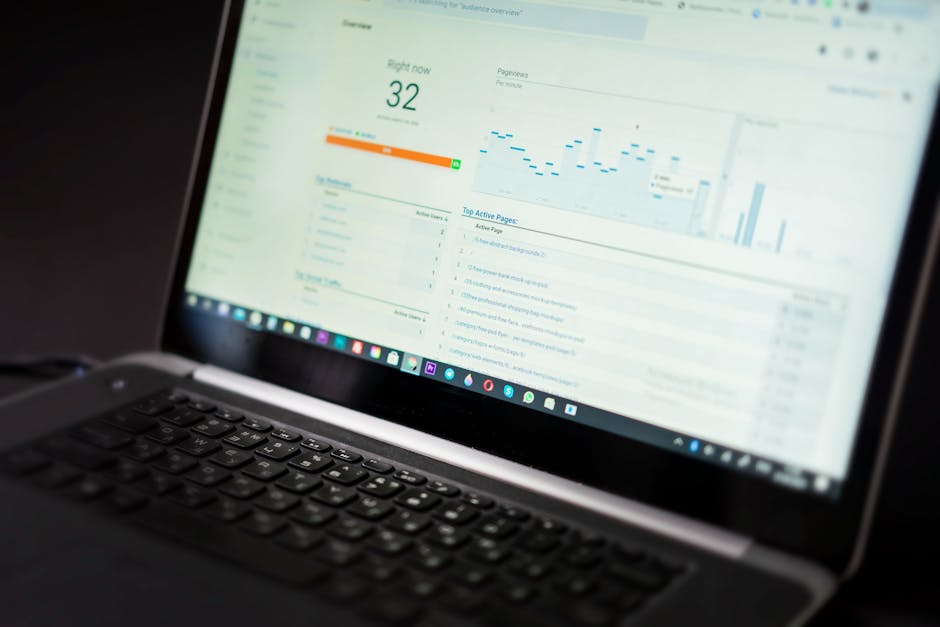
Evolution of Cost Forecasting in Healthcare
Historical analysis reveals that healthcare cost estimation has transformed from static budget models to integrated, analytics-driven approaches. Early methods struggled to adapt to rapidly shifting financial landscapes, but economic shifts and disruptive technological events have ushered in robust statistical models that harness continuous, real-time data. Furthermore, reconciling discrepancies between electronic health records and billing systems emphasizes the crucial role of data quality.
Real-Time Data Analytics in Cost Modeling
The integration of real-time data analytics is pivotal for achieving precise healthcare cost forecasting. By leveraging live metrics from extensive clinical and financial databases, organizations update predictions continuously. Advanced data pipelines process myriad information streams from multiple sources, ensuring that financial forecasts remain relevent and timely. This constant feedback loop allows immediate adjustments based on emerging trends and operational shifts.
Adaptable Models for Changing Dynamics
Innovative forecasting models are embracing flexible structures that combine regression analyses with heuristic logic layers for increased reliability. Empirical studies highlight that adaptive regression models can continuously refine cost estimates in dynamic environments. Such models are designed to adjust predictions based on new data inputs, ensuring resilience in the face of macroeconomic trends and internal changes.
In practice, these models allow organizations to pivot quickly, recalibrating their strategies to align with both short-term deviations and long-term trends.
Collaborative Forecasting through Cross-Functional Teams
A collaborative approach to forecasting capitalizes on the diverse expertise within cross-functional teams. Regular data review sessions and weekly updates have been shown to improve data ownership and forecast accuracy significantly. By sharing insights and aligning on key performance indicators, teams ensure that the forecasting process remains both rigorous and adaptable.
This collaborative environment, common in innovative sectors, bridges the gap between analytical rigor and operational realities.
Technical Methodologies and Case Study Insights
Technical advancements in regression modeling and KPI projection methodologies have provided tangible improvements in cost forecasting. Detailed case studies reveal that scenarios involving complex regulatory challenges benefit from the integration of real-time analytics, adaptable forecasting frameworks, and heuristic logic. The following table compares two widely used forecast models:
Model | Accuracy | Data Volume | Maintenance |
---|---|---|---|
ARIMA | High | Moderate | Frequent |
Prophet | Moderate | High | Occasional |
Considerations: Model selection depends on data volume, required prediction accuracy, and operational maintenance capabilities. Additional keywords: regression models, forecasting analytics, heuristic logic. |
Technical documentation often features formulas in monospace
font to underline the analytical depth involved in these calculations.
Conclusion
The evolving landscape of healthcare cost forecasting demands a blend of real-time analytics, adaptive modeling techniques, and robust cross-functional collaboration. By synthesizing advanced data analysis with flexible forecasting models, healthcare organizations are better positioned to address operational volatility and optimize financial performance. This integrated approach not only enhances predictive accuracy but also supports sustainable, strategic decision-making in an ever-changing healthcare environment.