Introduction
Remember the days when your local neighborhood shopkeeper instinctively knew what to stock and when just based on intuition? Back then, forecasting was all about gut feeling mixed with a bit of guesswork—a memory as charming as a leisurely stroll down St. Marks in New York City. Today’s landscape, however, is more like high-speed traffic on the Autobahn. With artificial intelligence now redefining precision forecasting, industries such as healthcare and finance are experiencing a strategic shift from reactive measures to proactive, real-time adjustments.
AI-driven models provide a level of clarity and rapid adaptability that basic methods simply cannot match. This transformation demonstrates that relying solely on intuition is a thing of the past.
- Drift Detection
- A method used to identify when a model’s performance starts diverging from expected outcomes in real-time.
- Confidence Interval Tuning
- The process of adjusting the range in which a KPI is expected to fall, ensuring forecasts remain reliable.
- Signal-to-Noise Ratio
- Measures the clarity of the informational input against the background data fluctuations.
The Rise of AI-Enhanced Forecasting
Think back to when predicting future trends felt as uncertain as predicting the weather during Wimbledon without historical data. Today, leading tech innovators like Nvidia power sophisticated algorithms that make advanced forecasting accessible to every industry. Through neural network-based similarity scoring and the integration of real-time KPI dashboards, old challenges such as fluctuating consumer behavior and seasonal demand are met head-on.
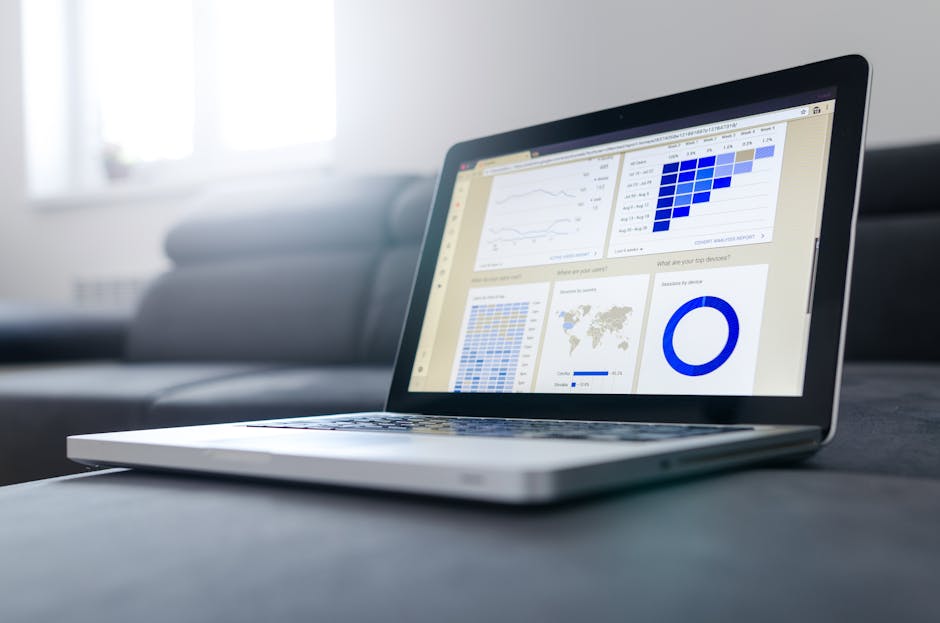
This dynamic blend of rigorous analytics and breakthrough machine learning ensures that precision is no longer optional but an essential part of the forecasting toolkit.
Aspect | Traditional Forecasting | AI-Driven Forecasting |
---|---|---|
Data Processing | Batch-based, delayed updates | Real-time, continuous updates |
Accuracy | Limited by static historical data | High precision through iterative learning |
Adaptability | Inflexible, slow to react | Dynamic adjustments and constant refinement |
User Interaction | Heavy reliance on manual tweaks | Automated insights enabling faster decision-making |
Key considerations: Continuous integration, iterative testing, and robust data cleaning help maintain forecasting efficacy. Look up more tables on AI-driven analytics and predictive models. |
Real-World Success Narratives
Consider a scenario in the healthcare sector—a story that resonates with transformation. Picture an operations director at a bustling metropolitan hospital in Chicago’s busy healthcare corridor. By retooling existing dashboards, she flagged deviations in patient flow and resource allocation almost instantly—much like air traffic controllers oversee planes at O’Hare International Airport.
This recalibration with AI-enabled dashboards boosted forecast reliability to 95%. Similarly, a global consumer goods corporation navigated turbulent supply chains by implementing advanced KPI models, converting potential inventory nightmares into streamlined operations.
"AI is not just a tool but a revolutionary force in operational efficiency. It transforms guesswork into measurable action." – A seasoned industry expert.
I've helped design GenAI flows that fallback to hand-tuned regex rules when structured inputs fail—because robustness matters.
Navigating and Overcoming Forecasting Pitfalls
Even with such advancements, precision forecasting faces its share of hurdles. Traditional methods often stumble due to an overdependence on static models and insufficient data refinement. Through dynamic adjustments bolstered by robust similarity scoring algorithms, these pitfalls are being steadily tackled.
In healthcare, iterative testing with key medical metrics has demonstrated considerable reductions in error margins and improved responsiveness to fluctuating patient volumes. Traceable AI-driven audits also ensure compliance with regulatory standards, functioning much like a seasoned New York City subway conductor who anticipates shifts in passenger demand.
- Similarity Scoring Algorithms
- Systems that compare current data trends against historical patterns to identify anomalies.
Actionable Insights and Future Directions
To sustain precision forecasting, continuous integration and flexible adaptation are key. Think of this as your hands-on guide to futuristic operations:
- Establish real-time KPI dashboards that provide instant feedback.
- Incorporate AI models that blend clear logic with similarity scoring to predict trends.
- Create robust frameworks for continuous forecast fine-tuning, ensuring data is always current and accurate.
- Implement a step-by-step troubleshooting guide to enhance internal data cleaning and synchronization.
These strategies empower leaders to navigate complex market dynamics, ensuring that AI forecasting digs deep into creating lasting and transformative operational impacts.
More Detailed Insights
When constructing internal data cleaning practices, consider automating routine checks for anomalies and integrating manual review workflows as backups. This dual approach not only boosts efficiency but also safeguards against unexpected system failures. Furthermore, seamless integration between AI-driven dashboards and traditional systems can bridge any technological gaps, fostering an environment of continuous improvement.
Embracing these practices will equip organizations to better understand market patterns, transforming raw model outputs (displayed here in a monospace
style) into actionable strategies. These enhancements represent a future where AI not only augments decision-making but redefines it.
Ultimately, the promise of AI in precision forecasting is a future where insights are timely, operations are smoother, and strategic decisions are smarter.