- Attribution Drift
- The challenge of tracking marketing efforts throughout the customer journey due to inconsistent data attribution.
- Signal Decay
- The gradual decline in data reliability and value over time if not constantly refreshed and validated.
Introduction
A leading analytics expert observed that the race for e-commerce excellence is now anchored in cross-functional analytics. Renowned firms like Amazon and Shopify are leveraging CRM algorithms powered by data science to uncover hidden trends within customer interactions. Academic insights from landmark studies on AI in CRM available on ScienceDirect, along with comprehensive definitions from TechTarget, lend credibility to these innovations. Improved conversion rates and enhanced customer lifetime values offer solid evidence of the CRM algorithm's efficacy.
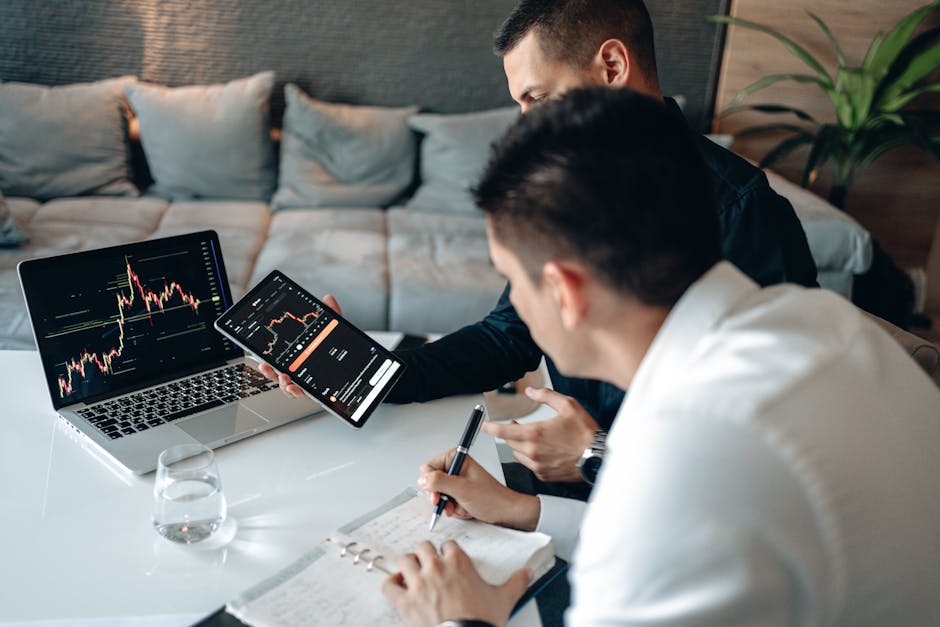
Recognizing the Challenges
Complexities arise when integrating sophisticated CRM algorithms across various teams. In practice, many corporations have faced disruptions from misaligned data inputs and the lack of a robust statistical backbone. For example, a multinational retailer encountered a sudden shift in customer behavior that forced both technology and analytics teams to quickly realign their strategies. The need to coordinate meeting notes with CRM inputs alongside evolving market signals remains a critical challenge, underscoring the importance of regular dashboard audits and prompt-based tool refinements.
A Turnaround Story
One prominent e-commerce platform struggled with ineffective segmentation even though it used state-of-the-art dashboards for cross-department collaboration. A deep dive into their data revealed delays in capturing attrition signals and erratic algorithm outputs that undermined their efforts. By merging insights from prompt-based tools with real customer data captured by established CRM systems, the team re-engineered its approach. Advanced prompt engineering transformed unstructured meeting notes into actionable insights, stabilizing predictive models and setting a new industry benchmark for responsiveness in real-time customer lifecycle management.
Data-Driven Solutions
The narrative shifted when the platform introduced a revamped analytics framework that combined algorithmic efficiency with clear visualizations. Detailed meeting notes were directly integrated into CRM insights, fostering real-time adjustments. The integration of statistical models with visual dashboards established a robust analytic pathway, ensuring that every element—from attrition signals to behavior trends—was captured accurately.
Metric | Pre-Optimization | Post-Optimization |
---|---|---|
Conversion Rate | 2.5% | 4.1% |
Customer Lifetime Value | $150 | $230 |
Session Duration | 2 minutes | 3.5 minutes |
Attrition Detection Speed | Delayed | Real-time |
Considerations: Robust statistical models, iterative data refresh, and cross-team collaboration are key elements for sustaining these enhanced metrics. Keywords: attrition signal detection, meeting note to CRM, statistical backbone needed. |
Tech giants such as Netflix and eBay have adopted similar approaches, tracking session duration shifts and user behavior patterns to seize retention opportunities—bridging human insight with algorithmic precision.
Conclusion and Actionable Takeaways
This exploration of cross-functional analytics demonstrates the transformative impact of aligning meeting insights with robust CRM algorithms to address unforeseen challenges. How can your organization iteratively adapt to evolving data inputs with actionable transparency? Readers are encouraged to evaluate whether their systems can agilely incorporate evolving data inputs. Adopting iterative and transparent analytic practices can inspire proactive change and drive long-term industry leadership.