Dirty Data Bleeds Revenue
In today's competitive landscape, clean data is more than a necessity—it is a powerful strategic asset. Numerous studies, including those referenced by revenue operation experts and featured in the Harvard Business Review, emphasize that every dollar invested in data verification can save exponentially more in future remedial costs. Ensuring robust data integrity is especially critical in environments where daily metrics and dashboard monitoring drive operational decisions.
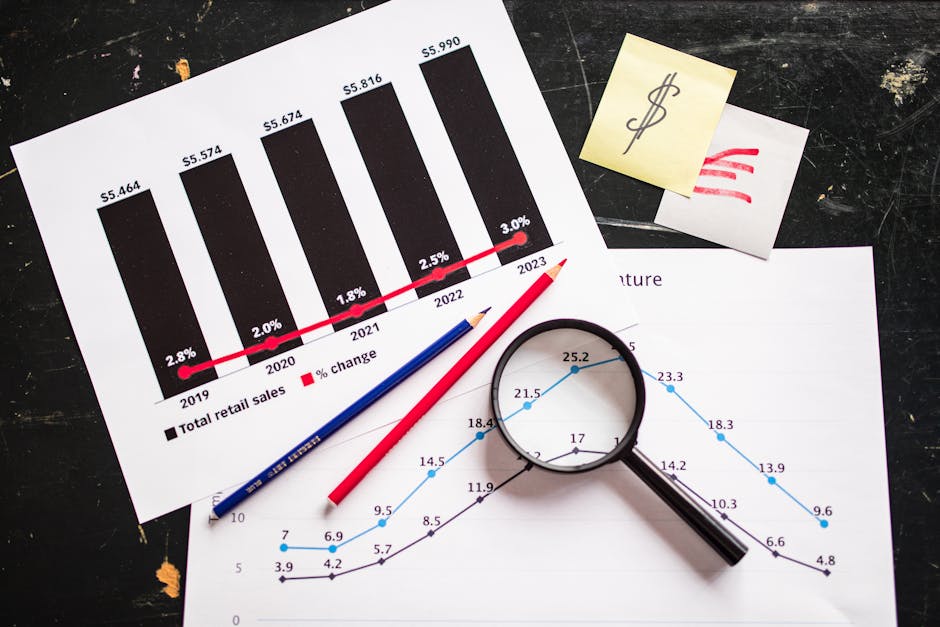
Setting the Stage for Data Integrity
Before diving into actionable strategies, it is crucial to understand the underlying economic rationale. Consider the widely acknowledged 1-10-100 rule: investing minimally in data verification yields returns that prevent significant annual losses. In industries where logistics and supply chain operations demand precise coordination, data anomalies directly hurt operations—an insight shared by industry veterans and financial fraud reviewers alike.
Uncovering Inconsistencies and Pitfalls
Imagine a scenario where product identifier mismatches cause logistics disruptions, or differences between warehouse scanners and ERP systems lead to delays. Manual audits often miss these subtle errors, resulting in cascading issues that affect customer support and contract reviews. Automating these checks with reliable scripts can detect anomalies early, reducing costly errors and safeguarding operational integrity.
Actionable Techniques for Data Cleaning
Implementing a systematic, step-by-step approach is key to maintaining clean data. The table below breaks down practical techniques used to address data discrepancies, integrating tools such as Tableau, PowerBI, and advanced AI-driven text-to-SQL models. These methods are essential for reconciling non-standard vendor data within complex ERP systems—especially in high-stakes supply chain operations.
Technique | Tool | When to Use | Impact on Churn |
---|---|---|---|
1 | Automated Scripts | Initial anomaly detection | High |
2 | Tableau / PowerBI | Routine audits | Moderate to High |
3 | Text-to-SQL Models | Complex ERP reconciliations | Very High |
4 | NLP Tools | Vendor data integration | Moderate |
Note: Integrating automation with human oversight is essential. Look for keywords such as "data verification," "automated audits," "ERP reconciliation," and "customer churn reduction" to discover similar strategies. |
Leveraging Cross-Departmental Insights
Clean data transcends the boundaries of IT and analytics—it directly strengthens departments like customer support and contract review. In real-world scenarios, data mismatches have led to critical delays in escalation, underscoring the need for precise data feeds. Improved data reliability feeds advanced survival analysis models, which in turn fine-tune retention strategies by accurately predicting customer behavior and vulnerability.
Sustaining Data Quality for a Strategic Advantage
Maintaining data quality is an ongoing, strategic endeavor. Integrating AI and machine learning for continuous validation and monitoring is vital. Organizations must avoid complacency by combining automated systems with vigilant human oversight—a dual approach ensuring resilient, high-quality data. This synergy not only reduces churn but also bolsters overall operational efficiency.
Industry Insider Definitions
- Data Decay
- A decline in data accuracy over time due to outdated or unverified information.
- Schema Drift
- Gradual changes in database structures that can lead to mismatches and errors if not managed.
- Churn Triggers
- Specific errors or anomalies in data that directly contribute to customer loss.
Final Thoughts
Embedding robust data cleaning practices into your operations is not just about avoiding errors—it is about strategically shifting the competitive balance. By adopting scalable and automated techniques, integrating cross-departmental insights, and maintaining a vigilant oversight with AI-driven tools, organizations can significantly reduce churn and drive sustainable growth. Remember, investing in data quality today adds exponential value to your bottom line tomorrow.
This approach is particularly vital in environments where every data point can impact the success of complex supply chain operations, customer support efficiency, and overall business resilience.