A New Era in Manufacturing AI
In today’s rapidly evolving industrial landscape, manufacturing companies are leveraging artificial intelligence to drive efficiency and empower decision-making. Traditional production methods are giving way to digital transformation—MES systems now synchronize with cloud-based analytics platforms to integrate real-time data and monitor KPIs across decentralized tech stacks. This evolution challenges long-held misconceptions about AI while reinforcing a commitment to transparency and reliability.
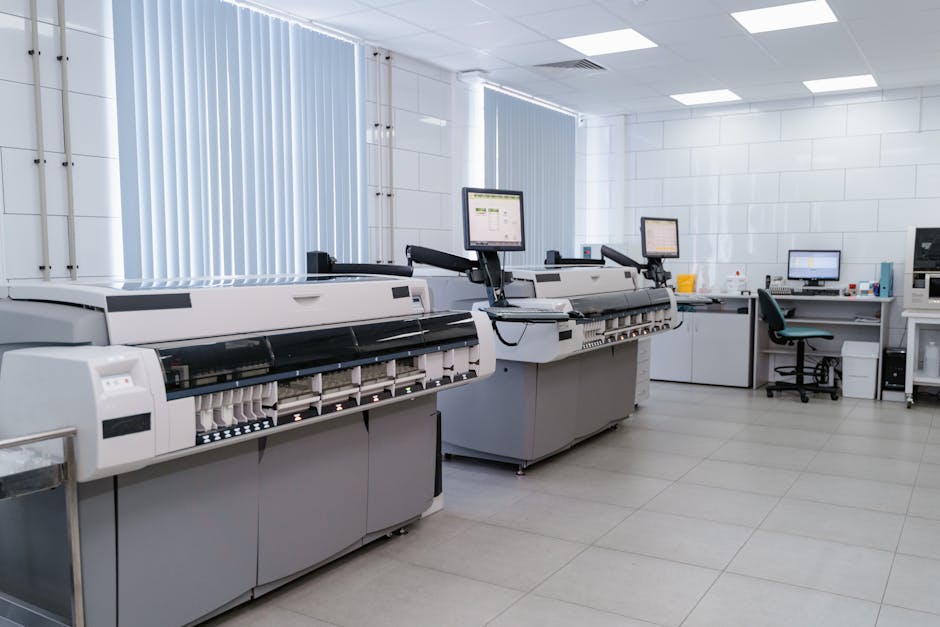
Policy and Production: A Guiding Framework
New regulatory frameworks, such as the European approach to AI, are laying the foundation for a trust-driven ecosystem. These policies are not mere formalities; they establish ethical standards and safe practices for AI deployment that support operational integrity and uphold public trust. Manufacturers worldwide now follow these guidelines to ensure that every step in the production process aligns with both strategic goals and societal responsibilities.
Data Integration in Action
Real-world examples of AI application are already transforming production spending. For instance, Wrapbook has harnessed AI to gain unprecedented control over production expenses—cutting costs by 15%. Through precise, AI-driven analytics, production accounting teams integrate data from diverse sources into actionable insights. This achievement serves as a beacon for manufacturing units navigating multiple, decentralized tech stacks.
Explore the Details of Data Integration Success
The integration of diverse data sources into a single, coherent platform not only streamlines production accounting but also promotes iterative feedback loops, ensuring that AI tools evolve with changing production patterns. Such integration has driven improvements in monitoring KPIs and refining production benchmarks.
Synthesizing Innovation at Scale
Visionaries in the field, like Kyle Coleman of copy.ai, are redefining AI-generated content as an integration of synthesized intelligence into existing operations. Coleman's approach, which built a $50M pipeline on modest investment, shows that AI tools must be deployed thoughtfully in order to enhance current processes and prevent the pitfalls of a black box system. As a testament to innovative problem solving, I’ve rebuilt flaky LangChain chains by replacing dynamic agents with tool-specific routing trees.
Sustainability and Mitigating Risks
AI is not only about increasing production efficiency—it is also key to fostering a sustainable future. Platforms like Clarity AI play an important role in carbon tracking and ESG reporting, while solutions from Blackbird.AI help organizations safeguard their reputations against narrative attacks. This dual approach addresses both environmental impact and the pressing need for transparent management of industry narratives.
Overcoming Misconceptions and Building Trust
Clear evidence from successful case studies, strategic partnerships, and ongoing innovation are vital to dispelling the myths surrounding AI's opaque nature and the build-versus-buy dilemma. Manufacturers build trust through regular KPI updates, transparent algorithms, and cross-channel communication—practices that ensure AI solutions continuously evolve with the market and operational data.
A Collaborative Roadmap for the Future
The future of manufacturing hinges on collaboration among industry giants, regulatory bodies, and technology experts. A structured ecosystem, built on robust AI trust-building strategies, empowers manufacturers to not only increase productivity but also create a legacy of responsible, sustainable innovation.
This collaborative approach secures an enduring competitive edge—ensuring every operational step is taken with both clarity and integrity.
Industry Essentials
- Model Drift
- The gradual decline in the predictive accuracy of a model due to changing environments or data patterns.
- Explainability
- The ability to interpret and describe the decision-making process of an AI model to ensure transparency and trust.
- Digital Twin
- A virtual replica of a physical system used to simulate, analyze, and enhance real-world performance.
Legacy vs AI-Integrated Workflows
Aspect | Traditional Workflow | AI-Integrated Workflow |
---|---|---|
Data Integration | Disconnected systems, manual consolidation | Real-time, automated data flows |
Decision Making | Experience-based, slower response | Data-driven, agile adjustments |
Cost Management | Higher overhead with manual reviews | Optimized spend with predictive analytics |
Sustainability | Limited ESG oversight | Integrated carbon tracking and ESG reporting |
Note: This comparison underscores key areas of transformation—data integration, decision-making speed, cost oversight, and sustainability. Keywords: KPI tracking, real-time data, predictive analytics, ESG reporting. |