- DATA LAKEHOUSE
- A modern data architecture that combines data lakes and data warehouses for scalable storage and analytics efficiency.
- ETL DRIFT
- A gradual, unmanaged shift in the ETL pipeline processes that can lead to inefficiencies and data inaccuracies over time.
Introduction
The supply chain landscape today is like a vast, intricate web where data pipelines work behind the scenes to keep operations efficient and costs low. Remember Maersk’s 2017 cyberattack? It serves as a real-world cautionary tale that underscores the need for operational resilience powered by agile data management. Major players in the industry, from retail behemoths to logistics leaders, are reaping the benefits of systems that swiftly ingest, transform, and analyze real-time data. This evolution—from mere report compilations to advanced, automated pipelines—is reshaping inventory management, logistical planning, and strategic decision-making.
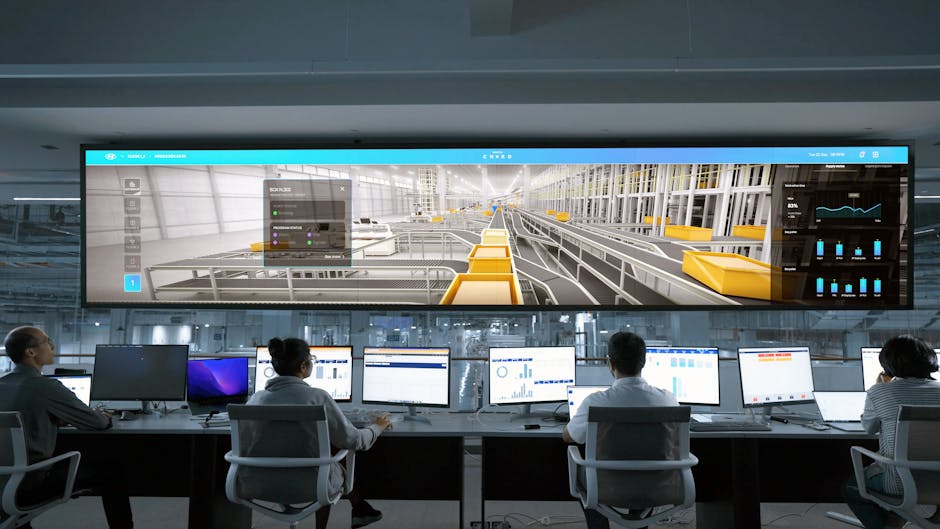
Expert Insights on Overcoming Automation Challenges
Industry experts discuss the hurdles of integrating legacy systems with modern cloud-based solutions—challenges that often lead to what some call data pipeline regret. By adopting strategic measures like API layer abstraction and microservices architecture, these issues become manageable. Thought leaders at conferences and analytics gurus alike emphasize the need for flexibility, scalability, and robust error-handling protocols along every stage of the pipeline. Designing a comprehensive blueprint from the ground up not only mitigates risks but also sets the stage for long-term success.
Case Studies: Real-World Success Stories
Real-world applications prove that the right data pipeline strategy boosts more than just efficiency—it transforms entire operational models. For example, Acceldata showcased a retail giant that achieved significant savings by implementing real-time monitoring of their data pipelines. Similarly, in transportation, integration with platforms like Azure Data Factory improved inventory forecasting and reduced turnaround times. These success stories highlight how embracing automated, data-driven approaches can lead to operational triumphs and even influence executive level strategies.
Innovative Technologies Driving Transformative Change
Today's technological landscape is revolutionizing data pipeline processes. Cloud computing advancements combined with event-driven architectures are easing the transition to automated workflows. Platforms such as Azure Data Factory handle complex workflows while emerging tools from providers like Hazelcast utilize real-time streaming for more efficient data ingestion. Implementing monitoring dashboards with automatic alerts can prevent data consumption failures, ensuring that operations—from warehouse management to transportation logistics—remain agile. Furthermore, the integration of predictive analytics—even driven by sensor data—enables near real-time corrective actions, minimizing downtime and adapting quickly to evolving market conditions.
Learn More About These Technologies
The transition to modern data pipelines is not just about updating technology—it involves rethinking the entire operational model. For instance, an API layer abstraction enables different software systems to communicate seamlessly. Likewise, a microservices architecture allows individual components of the data pipeline to be updated independently without causing major disruptions. Such innovations underscore the importance of adaptable system designs that are resilient even in the face of unexpected challenges.
Comparing Legacy and Automated Pipelines
Aspect | Legacy Pipeline | Automated Pipeline |
---|---|---|
Speed | Slow, manual interventions required | Fast, real-time processing |
Error Rate | High due to manual data handling | Low with automated error-handling |
Scalability | Limited and cumbersome | Easily scalable with cloud technology |
Maintenance | Time-consuming and reactive | Proactive monitoring and maintenance |
Considerations: Data integration, system agility, cost-effectiveness, and long-term operational efficiency are key when comparing pipelines. Keywords: speed, scalability, error rate, maintenance. |
Conclusion
In today’s fast-paced global supply chain environment, the stakes are too high to ignore the benefits of automated data pipelines. By learning from industry pioneers and incorporating innovative technologies, companies can avoid the pitfalls of data pipeline regret. Transitioning from outdated legacy systems to flexible, automated processes is not just an upgrade—it’s a strategic imperative that drives long-term success and fuels transformation within supply chain operations.
As operational demands continue to evolve, embracing comprehensive digital strategies means being prepared today for the challenges of tomorrow. The shift isn’t merely about technological investment; it’s about fostering a dynamic mindset that values proactive improvement and real-time responsiveness.