Transforming Patient Journeys with Traceable AI
“When every clinical decision is backed by transparent AI, you gain trust, precision, and care that truly matters.” – An Enablement Lead at a renowned hospital.
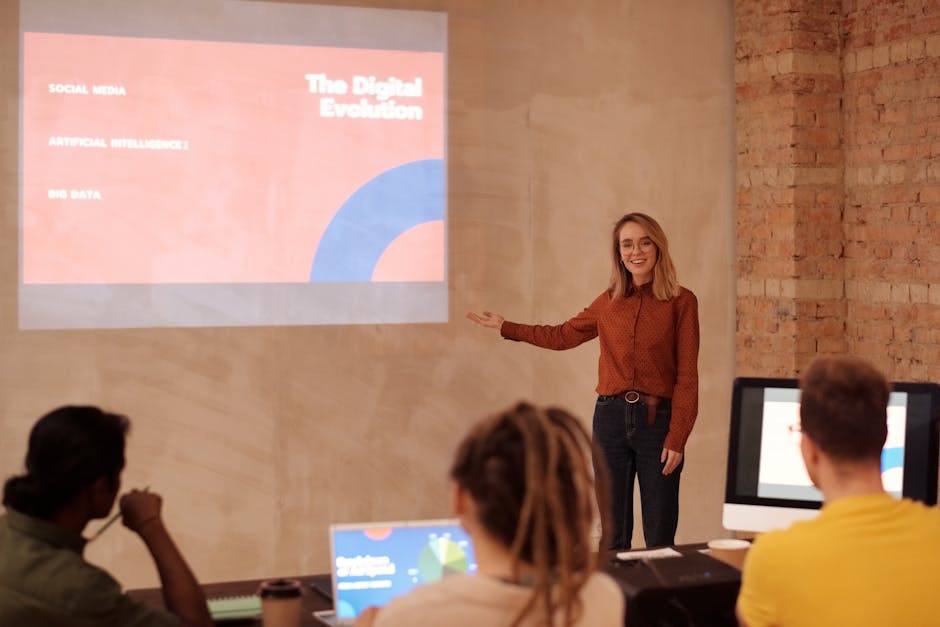
This guide explores a structured method to enhance AI transparency in healthcare. By transforming complex AI logic into traceable, audit-friendly models, leaders can safeguard patient outcomes and ensure operational efficiency. Let’s embark on a journey through each key checkpoint in this transformative process.
A Vertical Timeline of Key Checkpoints
- Concept Introduction
- This first checkpoint highlights the evolution of transparent, traceable AI logic—from the industry's early fascination with AI to its vital role in patient care today. It sets the stage for understanding how technology and healthcare can intersect to enhance decision-making.
- Technical Frameworks Unpacked
- Here, we delve into the statistical backbone behind AI models. With clear cause-and-effect trails, these models are designed for auditability. They are similar to those implemented by platforms like Salesforce Einstein and echo the precision required for modern healthcare analytics.
- Real-World Applications
- An exploration of radiology illustrates the application of traceable AI. Detailed examples from leading radiology centers demonstrate how linking every data point to verifiable logic can revolutionize diagnostics and enhance patient outcomes.
- Identifying Pitfalls & Mitigating Risks
- This checkpoint outlines common challenges such as mismanaged data and ambiguous logic. It presents robust solutions—grounded in risk management practices and supported by studies like those from Chartis Research—to ensure reliable and compliant AI integration.
- Interactive Learning
- Engagement is key. Interactive quizzes and checklists are introduced to reinforce learning at every module, empowering users to validate each step of the AI integration process and ensure they’re on track.
- Step-by-Step Integration Process
- We wrap up the timeline with a clear, methodical process for integrating traceable AI into existing healthcare systems. This includes rigorous data intake, alignment of internal tools, continual model validation, and periodic audits.
Deep Dive into Technical Frameworks
Modern AI models rely on statistical backbones that ensure every decision is traceable. In industries where patient data and operational efficiency are critical, these frameworks ensure that every output is rooted in a clear, auditable process. This level of transparency is not only essential for internal reviews but also pivotal when aligning with compliance standards such as HIPAA.
Real-World Transformation in Radiology
Radiology serves as an excellent case study of AI integration. By systematically linking every diagnosis to its underlying logic, leading radiology centers have enhanced accuracy and accountability. The process captures every data point, ensuring that diagnostic outcomes can always be traced back to verifiable clinical evidence.
“Imagine a scenario where every data point in a radiology report clearly shows its journey—from acquisition to final diagnosis—enabling a seamless, cross-functional review that builds trust across all stakeholders.”
Addressing Pitfalls with Robust Solutions
However, the path is not without challenges. Erroneous data changes, ambiguous internal logic, and unstructured model audits can compromise trust. A structured risk management framework can preempt these issues. By adopting well-documented processes along with traceable AI models, organizations can defend against data mishandling and ensure that every output meets rigorous quality and compliance standards.
Leveraging insights from research studies, such as those conducted by Chartis Research, this section details robust methods to mitigate potential risks while bolstering transparency and operational integrity.
Comparative Analysis of Traceability Frameworks
Understanding the nuances between different traceability frameworks can further empower teams in selecting the right tools for their needs.
Framework | Transparency | Speed | Clinical Trust |
---|---|---|---|
SHAP | High | Moderate | High |
LIME | Moderate | High | Moderate |
Custom Traceable Models | Very High | Variable | Very High |
Hybrid Approaches | High | High | High |
Note: Consider transparency and auditability as critical metrics when aligning AI outputs with healthcare compliance and internal operations. Keywords: traceable results, statistical backbone needed, workflow prioritization. |
Interactive Learning Tools for Effective Integration
Learning becomes more effective when it’s interactive. Real-time quizzes and checklists help ensure that every phase—from initial data collection to final model validation—is executed with precision. These tools empower teams to apply theoretical knowledge practically and verify compliance at each step.
View Examples of Interactive Checklists and Quizzes
The quizzes range from simple true/false questions to scenario-based queries that embed insights from daily cross-functional reviews. Meanwhile, checklists help ensure that all operational touchpoints are examined, reducing the risk of misalignment during model audits.
Step-by-Step Process for A Seamless Integration
The integration process begins with establishing a rigorous data intake procedure. Aligning internal tools with transparent business logic, teams can validate models and secure audit trails. Each step is reinforced by industry best practices and supported by dashboards that serve as plug-and-play overlays for existing systems.
This systematic approach ensures that every stakeholder—technical experts and operational leads alike—is operating from the same baseline, promoting consistency and accountability.
Strategic Recommendations and Final Thoughts
Embracing traceable AI logic is a continuous journey. Leaders are encouraged to adopt frameworks that not only support transparency but also build long-term trust and accountability within the system. With actionable strategies, historical evidence, and clear checkpoints, this guide serves as a dynamic blueprint for driving innovation in healthcare systems.
Integrating traceable AI into daily operations ensures that every clinical decision is backed by a robust and auditable process—a key step towards transforming healthcare for the better.