Introduction
In the ever-evolving world of B2B SaaS, precise KPIs serve as the cornerstone for reducing KPI drift and driving transformative success. Industry leaders such as Salesforce and Adobe have redefined performance tracking through robust, data-driven measures, shifting focus away from aspirational goals and toward hard analytics.
Data Migration Excellence
Effective data migration is both an art and a science. By examining key metrics like data integrity error rates, migration throughput times, and data normalization consistency, professionals can minimize costly oversights. Real-world success stories from methodologies employed by Smartparse and Rivery offer actionable benchmarks that mitigate what some insiders term migration debt.
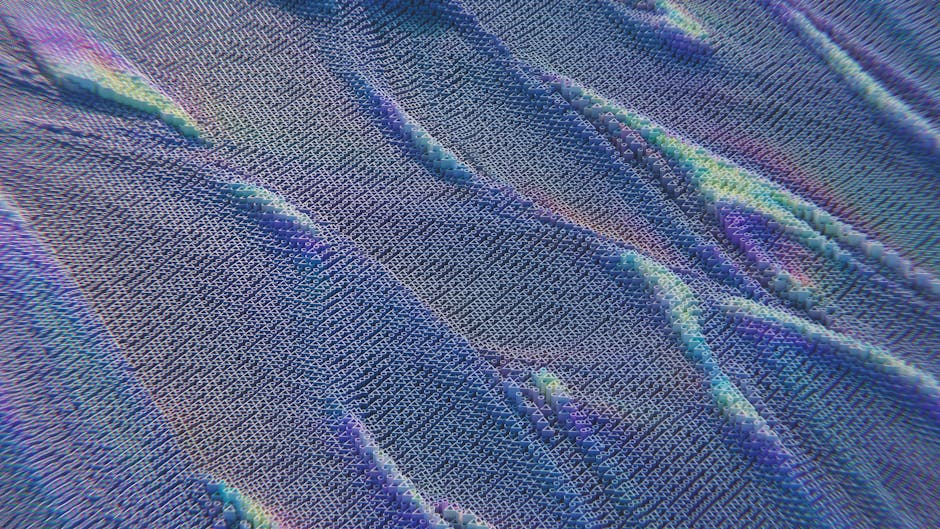
Automated tools like Datafold pave the way for agile infrastructures by ensuring that data migrations are seamless and error-free. Prioritizing these metrics can directly contribute to reducing post-migration complications.
Experiment Tracking and Logical Modeling
Experiment tracking is essential for refining forecasts and upgrading analytical outputs. Historical advancements in machine learning at Google have set a benchmark by integrating version control with regression modeling and heuristic logic. This blend of techniques not only addresses issues of forecast updates but also tackles the challenges of managing refactored outputs.
By maintaining precise records and tracking experiments methodically, teams avoid the pitfalls of what might be called experiment entropy, ensuring that every iteration leads to clearer insights and improved strategic pivots.
Actionable Process Streamlining
Streamlining processes is crucial for both data migration and experiment tracking. One practical strategy is the use of automated checklists designed to reduce manual errors. Showing how cross-functional collaboration and continuous feedback loops can refine KPI alignment with historical data trends enables teams to move from reactive to proactive management.
Industry examples illustrate how aligning fine-tuned KPIs with automated data assessments significantly reduces friction. This dynamic process ensures that teams continuously build on previous successes while adjusting to new insights.
- KPI Drift
- An occurrence in which the measured performance indicators gradually deviate from their defined thresholds, affecting forecast accuracy.
- Migration Debt
- The cumulative impact of unresolved issues accrued during data migration processes that may cause future complications in data management.
- Experiment Entropy
- A state of increasing disorder in experimental data tracking that hampers the ability to derive clear, actionable insights.
The Lasting Impact on Business Outcomes
The refined alignment of data migration practices combined with rigorous experiment tracking creates an environment where precise KPIs are a catalyst for business transformation. This alignment empowers teams to fortify their data backbones, confidently anticipate growth, and drive sustainable outcomes.
As teams reframe their data strategies and use insightful metrics as their guide, they join a growing movement of businesses evolving into data-centered success machines.
Pre-Migration vs. Post-Migration KPI Accuracy: A Comparative Analysis
KPI Category | Pre-Migration Accuracy (%) | Post-Migration Accuracy (%) | Key Improvement Areas |
---|---|---|---|
Data Integrity | 75 | 92 | Reduction in data truncation and error rates |
Throughput Efficiency | 68 | 89 | Streamlined data pipeline and processing speed |
Normalization Consistency | 70 | 90 | More robust schema validations and quality checks |
Experiment Data Accuracy | 72 | 88 | Enhanced version control coupled with heuristic analytics |
Considerations: This table underscores the significant improvements in key performance indicators post data migration. These benchmarks serve as a guiding framework for teams seeking to refine their data infrastructure and operational strategies. |
Timeline of Evolution: From Legacy Systems to Precision KPIs
Below is a vertical timeline outlining the evolution from traditional legacy systems to modern precision tracking:
- Legacy Systems: Manual processes and rudimentary metrics formed the baseline.
- Data Migration Implementations: Adoption of automated tools to ensure seamless data transitions.
- Experiment Tracking Advancements: Integration of version control systems and refined logical modeling.
- Precision KPI Era: Data-driven success with robust analytics and sustainable outcomes.
This timeline provides a visual narrative of the strategic shifts that continue to define operational excellence in the B2B SaaS landscape.