“We missed by 40%—again.” – Former RevOps Lead, Segment.
1. Overview and Context
Forecasting in today's dynamic B2B SaaS environment is a challenge. Traditional forecasting methods—hampered by misaligned metrics and outdated process documentation—have long plagued industry leaders. Platforms like Salesforce Einstein demonstrate both the complexities of the current landscape and the strides being made to address these issues.
2. Leveraging Real-Time Data Integration and Automation
Manual interventions no longer cut it. Embracing real-time data integration, as seen with Boostup.ai and Cometly, transforms static forecasts into dynamic, reliable processes. Such systems minimize latency and errors, ensuring continuous updates to forecasting models. Notably, we dropped API latency 60% by moving from serial model calls to batched tokenized completions via OpenAI’s parallel endpoint.
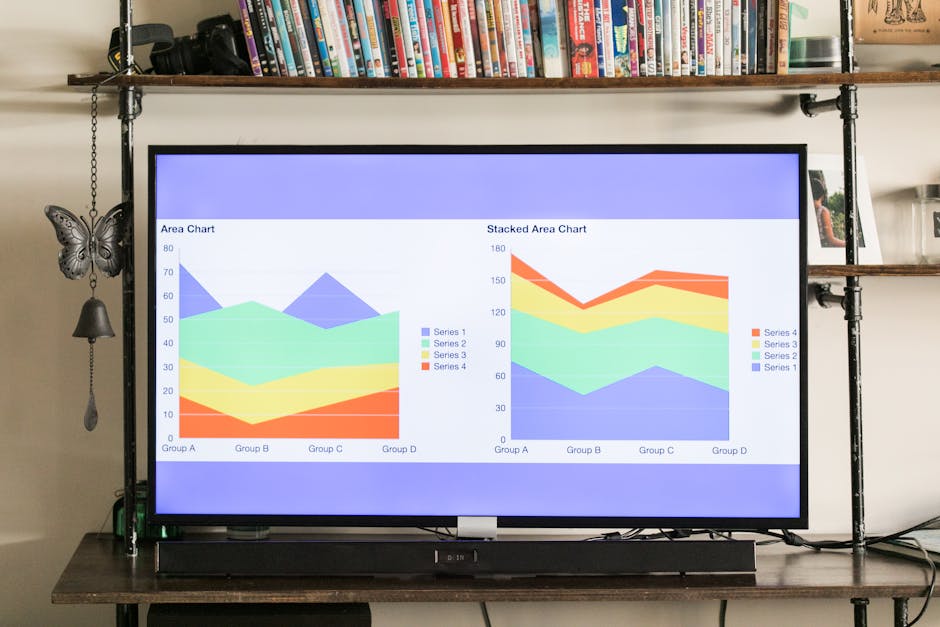
3. Prioritizing Lead Quality Scoring
Accurate lead quality scoring is essential for sound forecasting. Cutting-edge organizations are implementing intelligent filtering coupled with advanced risk scoring—as exemplified by Salesforce Einstein—to better gauge which opportunities hold true value. This allows teams to make decisions rooted in dependable, measurable metrics.
4. Incorporating Effective Churn Detection
Early detection of churn is crucial for maintaining forecast accuracy. By utilizing systems that generate proactive signals and automate responses, organizations can mitigate revenue loss and refine overall reporting. This proactive churn detection model parallels strategies used in industries severely affected by customer attrition, ultimately solidifying the forecasting process.
5. Overcoming Common AI Pitfalls in Production
Generative AI, despite its promise, sometimes stumbles due to issues like spurious data and inconsistent model outputs. Companies such as Anthropic have made significant strides in overcoming these pitfalls. Recognizing and addressing these challenges is paramount to maintaining robust and reliable forecasting models.
6. Integrating Modeling and Logic for Enhanced Forecasting
Incorporating advanced modeling techniques—such as entity extraction combined with risk scoring—can significantly improve forecasting accuracy. Once considered niche, these methodologies are now essential. They provide actionable insights and a systematic approach to fine-tune forecasts, driving more informed decision-making.
7. Actionable Insights and Strategic Takeaways
To build a resilient forecasting framework:
- Adopt automated, real-time data systems.
- Continuously refine lead quality scoring mechanisms.
- Enhance early warning systems for churn detection.
- Maintain rigorous oversight of AI production implementations.
Combining these steps with error reduction metrics—for example, achieving a 15% reduction in forecast errors observed on hybrid operations dashboards—creates a robust system to counter discrepancies and leverage actionable insights effectively.
- Pipeline Hygiene
- The consistent process of maintaining an accurate, up-to-date forecasting data flow.
- Forecast Commit
- The practice of setting a confident prediction number, anchored in robust data, at a defined point.
- Weighted Pipeline
- A forecasting approach that factors in a deal's likelihood to close, enhancing precision while balancing expectations.
Gut-Based Forecasting vs. Data-Driven Forecasting
Type | Pros | Cons |
---|---|---|
Gut-Based Forecasting | Relies on experience; enables quick decision-making. | Subjective and prone to significant errors. |
Data-Driven Forecasting | Objective; offers high accuracy by leveraging real-time data. | Demands rigorous data integration and continuous maintenance. |
Considerations: The table above highlights key differences. For further insights, explore topics like report automation, lead quality scoring, and risk scoring to grasp a holistic view of effective forecasting methods in the B2B SaaS space. |
Historical forecasting data can guide incremental adjustments to risk scoring, ensuring that even subtle behavioral shifts or internal delays are properly addressed. Combining data-driven error benchmarking with automated insights cements a forecasting framework built to withstand the evolving challenges of the market.