Advancing Efficiency with Custom AI
- Custom AI Models
- A tailored set of algorithms designed to perform specific data-driven tasks within operational systems.
- Workflow Efficiency
- The enhancement of operational processes, reducing manual effort and increasing throughput.
- Seamless Integration
- The smooth and efficient merging of advanced AI solutions with existing systems without disruption.
Custom AI models have revolutionized operational workflows, transforming tedious manual tasks into efficient, data‐centric processes. These solutions are not just theoretical—they have reshaped industries ranging from healthcare to enterprise meeting management by increasing both scalability and efficiency.
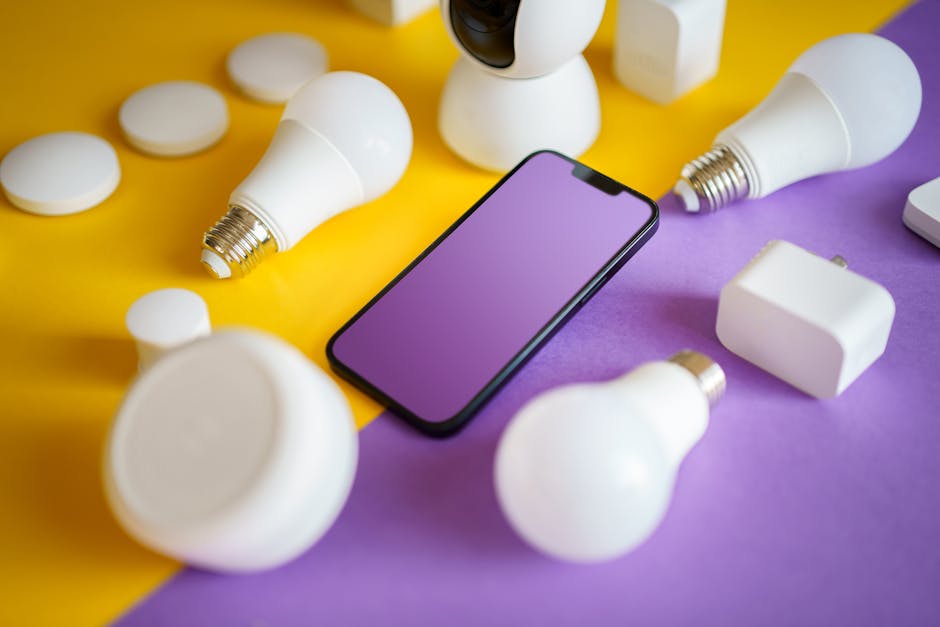
Real-World Lessons Across Industries
Institutions such as hospitals and clinics have adopted custom AI models to manage patient care, risk assessment, and resource allocation. A pivotal study by the National Center for Biotechnology Information documents these efforts, proving that specialized models are indispensable under high-stakes scenarios. These real-life applications emphasize the transformative power of tailored AI solutions.
Insights from Industry Leaders
Leading companies such as Google Cloud and Microsoft have demonstrated the immense potential of custom AI tools. Their innovative gen AI use cases and AI-driven reporting tools showcase how integrating advanced models can streamline internal processes and enhance cross-departmental decision-making.
Integrating AI Tools with Legacy Systems
Modern AI enhancements are designed to integrate with existing infrastructures without requiring expensive overhauls. Solutions like real-time transcription and intelligent search—exemplified by companies such as Read AI—illustrate how traditional systems can be enriched. Data from old databases can now be seamlessly fed into dynamic dashboards that provide actionable insights for efficient risk scoring and meeting summaries.
Actionable Steps for AI Adoption
Implementing custom AI models starts with identifying key processes that would benefit from automation or enhanced analysis. Areas such as manual form cleaning, risk scoring, and workflow prioritization are ripe for transformation.
How do you verify the effectiveness of these models? Establish quantifiable metrics like reduced manual processing time and improved data accuracy to validate enhancements. Experiment with approaches such as Python prototyping or prompt chaining to iterate rapidly and meet internal audit standards.
Bridging Legacy Systems with Modern Intelligence
Custom AI models connect older systems with modern intelligence. By harnessing techniques like similarity scoring and classification, organizations can consolidate various processes into coherent dashboards. This not only simplifies internal tools but also improves the accuracy of data integration. For example, practical applications of similarity scoring have demonstrated a 30% boost in data alignment accuracy within automated report generation, ensuring reliability and efficiency.
The Call to Innovate
It is time for leaders to embrace the transformative power of custom AI models. By implementing these tools, organizations overcome conventional constraints and achieve new standards of workflow efficiency. The era of tailored AI integration is here; leverage actionable insights and join the forefront of innovation. Continuous refinement through techniques like prompt chaining provides a dynamic approach to evolving data sources and ensures long-term precision in operational processes.
In the spirit of informed decision-making and innovation, consider the practical comparisons between prebuilt and custom AI models below:
Factor | Prebuilt Models | Custom Models |
---|---|---|
Latency | Higher due to generalized design | Optimized for specific tasks |
Cost | Lower upfront, but inefficiencies may add up | Invests in long-term efficiency gains |
Control | Limited adjustments | Fully customizable to unique workflows |
Scalability | General scalability features | Tailored scalability for high-demand processes |
Considerations: Evaluate latency, cost, control, and scalability when deciding between prebuilt and custom AI models. Keywords for further research include "custom AI integration," "workflow automation," and "tailored machine learning." |