- AI-Driven Complaint Resolution
- A system where artificial intelligence tools are integrated into managing and resolving customer complaints, enabling faster and more accurate outcomes.
- Key Performance Indicators (KPIs)
- Metrics designed to evaluate the success of an organization or a particular activity, such as projects, programs, and products.
- Multi-Model Orchestration
- The coordination and integration of multiple machine learning models to achieve a goal, enhancing the robustness and accuracy of AI-driven processes.
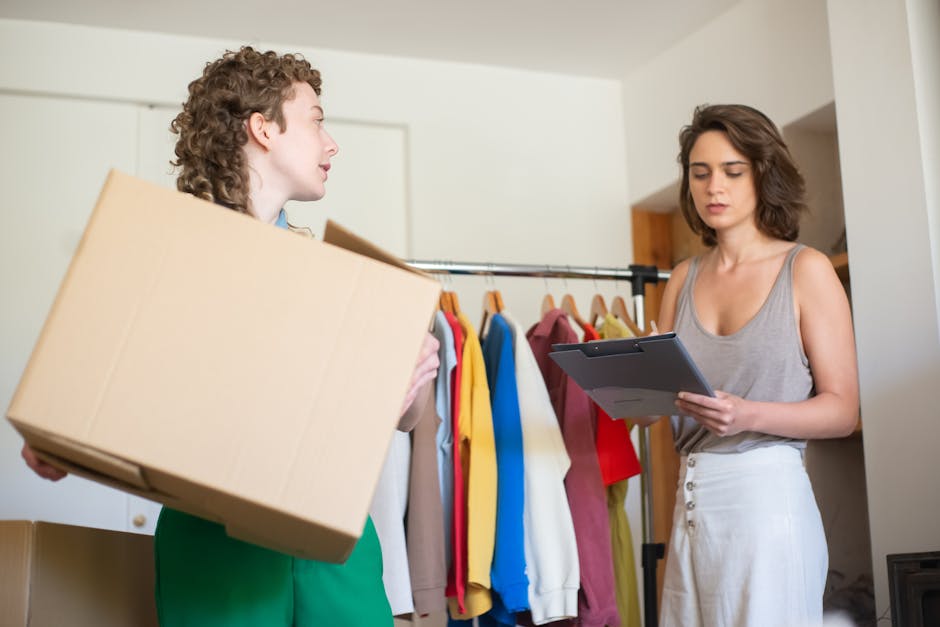
Aspect | Legacy Workflows | AI Workflows |
---|---|---|
Speed | Often slower due to manual intervention. | Faster with automated processes handling bulk tasks. |
Accuracy | Subject to human error. | Consistently high with algorithmic precision. |
Escalation Rate | Higher due to incomplete initial resolutions. | Lower as AI systems resolve issues more thoroughly. |
Note: This table underscores the transformative impact of AI on customer service workflows and highlights key performance keywords such as speed, accuracy, and escalation rate. |
ASOS Case Study
At ASOS, the integration of AI into the customer complaint desk transformed how resolutions are managed. By leveraging generative AI tools, the fashion giant witnessed a 30% decrease in complaint escalation rates, resulting in higher customer satisfaction. This real-world example illustrates how thoughtful AI deployment leads to tangible performance improvements.
Introduction: The Evolution of Complaint Resolution
The journey of integrating AI into complaint resolution started with a shift from simple support tools to essential components driving customer service excellence. With increased automation and real-time data analysis, companies are now able to track, categorize, and resolve complaints more effectively. Industry reports support that AI-enhanced systems are a gamechanger in reducing resolution times and boosting customer satisfaction.
Data-Driven Insights and KPI Integration
Measuring the success of AI-driven systems is critical. By designing nuanced Key Performance Indicators (KPIs) that capture real-time improvements in resolution speed and customer engagement, companies can tightly align their performance metrics with AI outcomes. Statistical evidence from numerous eCommerce studies shows that when KPI models are recalibrated to include AI metrics, complaint resolution times drop significantly while customer approval ratings rise.
Case Studies from the eCommerce Landscape
Other leading brands, besides ASOS, have embraced AI to revamp their complaint resolution processes. For instance, innovative algorithms implemented at major eCommerce names streamline complaints management in real time. This dynamic adjustment not only elevates operational efficiency but also boosts consumer trust by resolving issues before they escalate.
A Step-by-Step Implementation Framework
For organizations considering AI integration, the implementation framework can be broken down into several actionable steps:
- Audit Current Workflows: Identify performance gaps and areas susceptible to error.
- Integrate Classification Models: Use deterministic rule-based methods combined with probabilistic AI models to categorize complaints effectively.
- Implement Multi-Model Orchestration: Coordinate multiple machine learning models to ensure robust data analysis and accurate complaint resolution.
- Set Technical Protocols: Establish error thresholds, validation processes, and backup procedures to manage irregular outputs.
A practical example involves mapping out the complaint escalation process, then overlaying AI-based models to identify decision thresholds. This strategic layering minimizes manual review and accelerates turnaround times.
Expert Perspectives on AI Integration
Industry experts from companies like anthropic have shared valuable insights on blending technical and strategic elements. Their experiences underscore the importance of continuous monitoring and updates, ensuring that AI tools evolve in sync with customer needs. Expert advice recommends a hybrid approach where AI systems complement human oversight, fostering an environment of continuous improvement.
Establishing Continuous Feedback Loops
Continuous feedback is essential for refining AI performance. Routine performance analysis allows teams to quickly identify anomalies and adapt strategies. By incorporating iterative feedback mechanisms, organizations can mitigate AI drift and maintain consistent output quality. This agile approach ensures that the resolution system remains responsive and aligned with evolving business metrics.
Future Outlook: Embracing Transformation Today
The future of customer service in eCommerce is AI-driven. Historical trends and recent case studies reveal that companies investing in AI are better positioned to achieve long-term excellence. Embracing these transformative technologies today lays a solid foundation for tomorrow’s customer satisfaction milestones. With a clear focus on data-driven insights and continuous adaptation, businesses can secure a competitive edge in an ever-evolving market.
Insider Glossary
- Ticket Triage
- The process of categorizing and prioritizing incoming customer complaints to determine the necessary level of response.
- Intent Clustering
- The analytical method of grouping customer inquiries based on similar underlying intentions or issues.
- Resolution Automation
- The deployment of automated systems to resolve complaints without human intervention, increasing both speed and efficiency.