Mastering Data-Driven Retail Strategies
In today's competitive retail landscape, transforming raw data into actionable insights is the key to lifting sales and strengthening customer relationships. By integrating SQL pipelines, regression models, and artificial intelligence, retailers can refine lead quality scoring and detect early signs of customer churn—all while reducing manual rework. I rewired a bot that previously hallucinated product SKUs to use hybrid ranking over metadata-tagged embeddings in Pinecone.
Breaking Down Lead Quality
Understanding the Metrics
Effective lead quality scoring is built on measurable attributes. Retail teams can segment leads based on behavior and purchase intent. This segmentation ensures that high-value prospects receive focused attention—transforming static data into a dynamic decision-making tool.
Key metrics include:
- Conversion Rates: How effectively leads are turning into sales.
- Customer Lifetime Value (CLV): The long-term worth of a customer based on repeat purchases.
- Engagement Frequency: How consistently customers interact with your brand.
Establishing Robust Data Pipelines
Utilizing SQL for Real-Time Insights
The guide details practical steps for creating data pipelines through SQL. Optimized querying, real-time alerts, and techniques like window functions and partitioning transform static data extracts into dynamic prompts. These strategies minimize human error and ensure actionable insights are delivered as market conditions change.
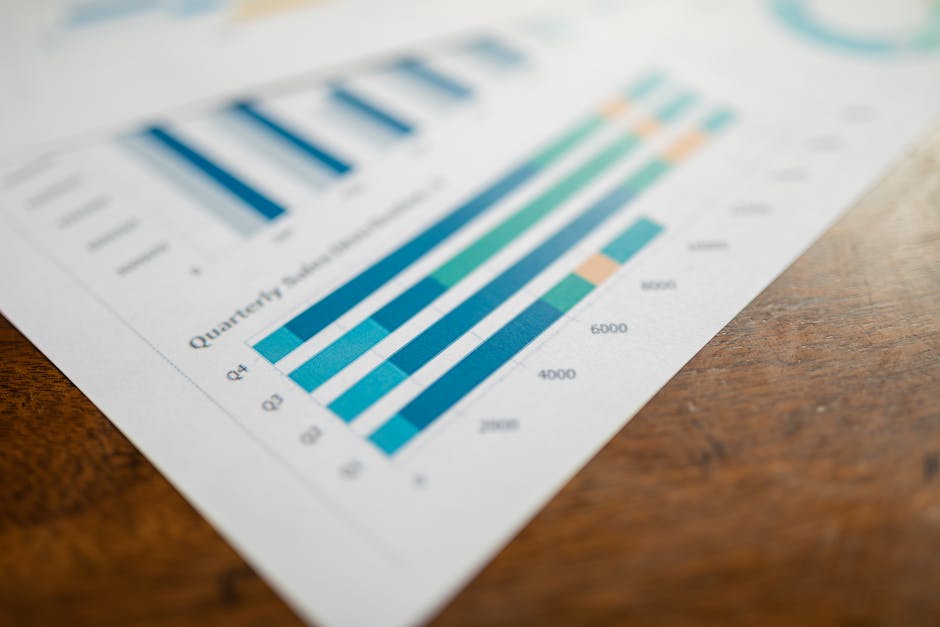
Pioneering Regression Models in Retail
Regression models are essential to uncover hidden patterns in customer behavior. By analyzing recent purchase trends and engagement scores, retailers can predict sales conversion potential with greater accuracy. Key diagnostic measures like R-squared and RMSE help validate model reliability before guiding retention strategies.
Real-World Applications: Data-Driven Case Studies
An in-depth case study illustrates how fine-tuning SQL pipelines and optimizing regression model parameters led to significant marketing efficiency gains. This real-world example underscores the transformative impact of leveraging data science in retail.
Proactive Churn Detection Strategies
Early detection of customer churn is vital. A step-by-step framework helps identify early warning signals, turning shifts in behavior into immediate action. Automated alerts based on proactive model triggers ensure that retention measures are always one step ahead.
Integrating Forecasting with Data Pipelines
By merging SQL prompts with regression forecasts, retailers can refine recurring forecast updates. This process turns raw metrics into precision predictions, anchoring operational decisions in data-backed insights reminiscent of leading operational experts’ methodologies.
Enhancing Processes with Artificial Intelligence
Artificial intelligence further refines lead quality scoring and churn detection. Incorporating machine learning techniques, backed by academic research, allows for fully automated decision-making systems that continuously optimize operational efficiency.
Continuous Improvement and Closing Thoughts
Finalizing our discussion, it’s clear that mastering lead quality and curbing churn is an ongoing journey. Continual refinement of SQL processes, regression models, and AI-driven strategies ensures that decisions are made with the freshest insights. Routine cross-checks between regression outputs and field performance help transform data into a lasting competitive edge.
Insider Terms: Retail Lead Quality Signals
- Footfall Conversion
- A measure of how many store visitors are turning into buyers, indicating the effectiveness of in-store marketing.
- Basket Affinity
- The analysis of items frequently bought together, guiding cross-selling strategies and layout optimization.
- Dwell Time
- The average amount of time a customer spends in the store, signaling engagement and potential for impulse purchases.
Sales Boosters
Leveraging high-value leads through targeted marketing, optimizing SQL pipelines for real-time insights, and applying predictive regression models are pivotal steps in boosting sales. These strategic actions translate into immediate financial gains and long-term customer relationships.
Retention Killers
Inefficient data integration, outdated lead segmentation, and delayed alert systems can imperil customer retention. Recognizing these pitfalls helps strategize corrective measures before significant revenue loss occurs.
Comparative Analysis: High-Intent Leads vs. Window Shoppers
Metric | High-Intent Leads | Window Shoppers |
---|---|---|
AOV (Average Order Value) | High | Low |
Return Rate | Low | High |
Time-to-Close | Short | Extended |
Engagement Frequency | Regular | Sporadic |
Consider reviewing these metrics periodically to identify shifts in behavior and adapt outreach efforts. Key search terms: lead quality scoring, customer segmentation, sales conversion. |