I. Introduction
In today’s fast-changing fintech arena, outdated, manual forecasting is making way for a revolution powered by artificial intelligence. The era of relying on cumbersome spreadsheets and guesswork is ending. Instead of laboriously reconciling data across multiple sources, modern systems equipped with regression models are streamlining financial forecasting. This transformation not only enhances precision but also accelerates decision-making.
Sticky Title: Forecast Like a Pro: Tapping into AI-Driven Automation and Regression Models in Fintech
II. AI and the Fintech Revolution
At the core of this transformation is a new breed of predictive analytics that leverages advanced regression models. Leading firms such as IBM Watson showcase the tangible benefits of automating complex financial modeling. Regression models are continuously validated and retrained against key performance indicators, ensuring they adapt as data evolves. With AI learning from ever-growing pools of data in real time, businesses can unlock insights and prevent costly errors long before traditional methods even detect discrepancies.
Modern techniques—even those using Bayesian regression—offer confidence intervals that provide clearer risk insights. This evolution is setting the stage for efficiency levels that were once thought unattainable, reshaping how financial institutions approach forecasting.
III. Case Studies of Transformation
Several prominent financial institutions have adopted AI-powered forecasting with impressive results. For example, one major investment bank replaced its manual forecasting routine with an AI-driven model that analyzed historical trends alongside current market dynamics. By using regression models, the bank significantly improved accuracy and reduced operational risks by identifying potential areas of drift in advance.
Similarly, a leading venture firm harnessed AI to streamline portfolio analysis, cutting down the time spent on traditional data reconciliation and enhancing overall precision. Hybrid modeling approaches—combining deep learning with classical statistical methods like ARIMA or OLS regression—further elevate the sophistication of financial forecasts in volatile markets.
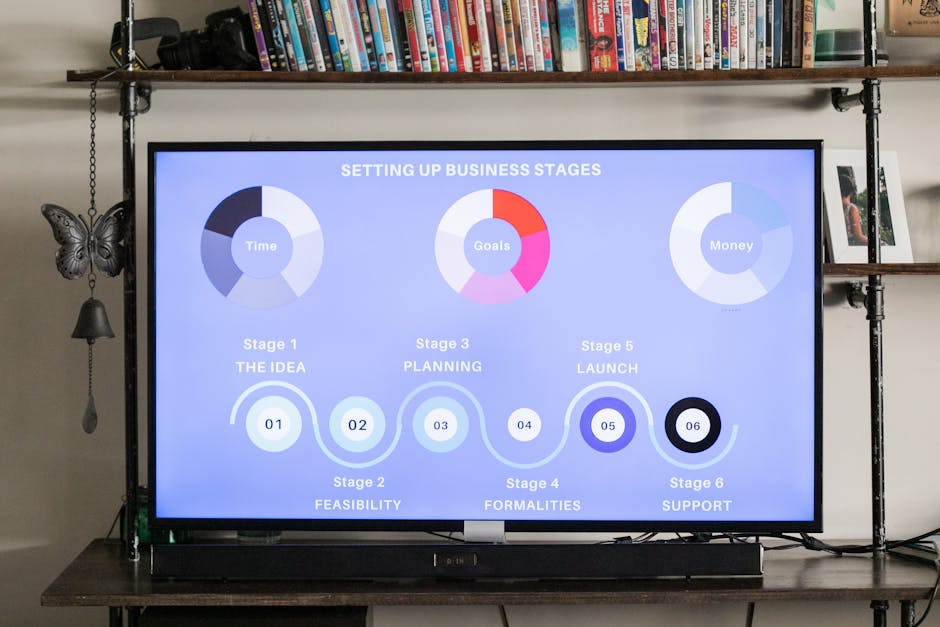
IV. Actionable Insights for the Now
For professionals who manage manual reporting processes, integrating automated regression models into existing frameworks can transform cumbersome tasks into efficient, error-resistant ones. Companies should consider replacing legacy systems with advanced AI solutions capable of near-instantaneous error detection and corrective measures.
However, while automated forecasting reduces manual errors, expert review remains essential. Algorithmic outputs must be cross-verified against regulatory benchmarks and historical performance to ensure full compliance and accuracy. This combined approach helps future-proof decision-making processes by seamlessly integrating human expertise with technological precision.
Comparative Analysis: Manual vs AI-Driven Forecasting
Metric | Manual Forecasting | AI-Driven Forecasting |
---|---|---|
Speed | Slower due to data reconciliation processes | Near-instantaneous analysis through automation |
Accuracy | Susceptible to human error | High accuracy with continuous validation |
Cost | Higher operational overhead | Reduced costs via optimized processes |
Risk Management | Delayed detection of operational risks | Proactive identification of potential drift |
Note: This table highlights key differences in forecasting approaches. For further keyword searches, consider terms like "operational efficiency", "predictive analytics", and "regression models." |
V. Future Trends and Evolving Landscapes
Looking ahead, AI’s role in financial forecasting is primed to deepen. The transition from static regression models to adaptive, learning algorithms is set to further enhance both accuracy and operational resilience. Continuous experimentation, coupled with rigorous tracking of model performance, is fast becoming a best practice.
In the coming years, the most competitive firms will not only adopt these innovations but will also drive further advancements through sustained investment and research. In this evolving narrative, AI-driven transformation is scripting a future where efficiency, precision, and proactive strategy become the cornerstone of success in the financial industry.
The integration of advanced regression techniques with AI-driven automation serves as a critical asset for organizations seeking to overcome traditional reporting challenges and embrace a more dynamic, accurate forecasting paradigm.
- Regression Models
- Statistical techniques that predict a numerical value based on historical data patterns.
- Predictive Analytics
- Technology that uses data, statistical algorithms, and machine learning techniques to identify the likelihood of future outcomes.
- AI-Driven Automation
- Processes that leverage artificial intelligence to streamline and optimize repetitive tasks.
- Probabilistic Forecasting
- Forecasting that provides a range of potential outcomes and the likelihood of each, as opposed to a single-point prediction.
- Hybrid Modeling
- Combining traditional statistical methods with modern machine learning techniques to improve the accuracy and interpretation of forecasts.