A Journey of Operational Evolution
The evolution of onboarding efficacy and model traceability in e-commerce reveals a narrative of progress and transformation. By tracing the path from early operational practices to today’s data-driven strategies, this discussion guides organizations in integrating advanced systems and overcoming obstacles with insights drawn from renowned global enterprises and tech innovators.
Streamlining Onboarding for Operational Efficiency
In the digital market’s fast-paced environment, inefficient onboarding directly impacts operational throughput and customer satisfaction. Leading e-commerce players have experienced delays that obstruct progress even in innovative firms. By adopting robust training, real-time data syncing, and backed accountability mechanisms, companies can reduce integration delays by upwards of 30%—answering the common question: How can we lower onboarding friction?
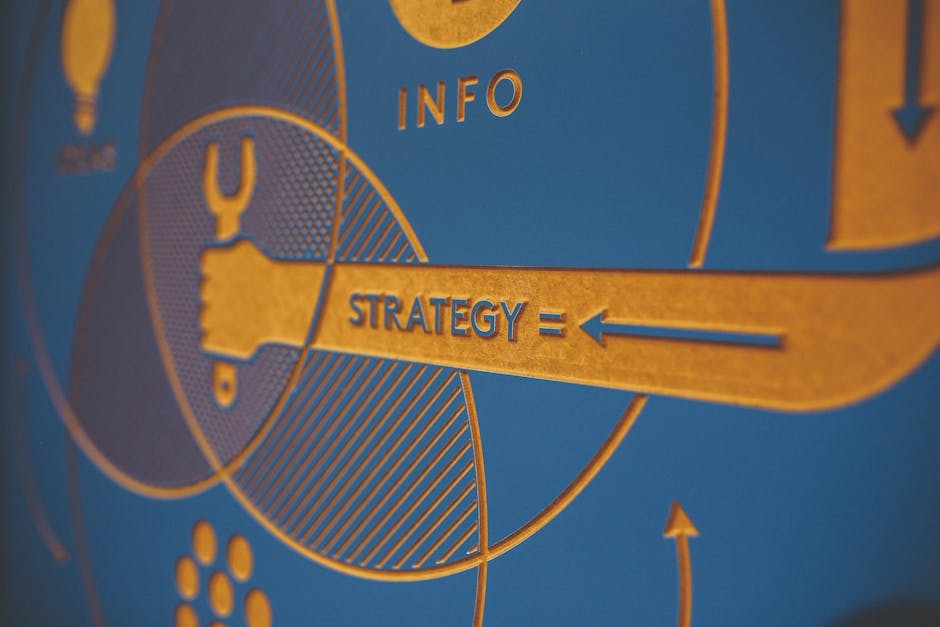
Learn more about onboarding challenges and solutions
Detailed case studies reveal that embedding error detection and continuous training significantly smooths the onboarding process. Companies employing these measures have observed not only faster integration times but also improved satisfaction metrics from both employees and clients.
The Impact of Model Traceability
For decades, model traceability has underpinned operational integrity; ensuring reproducibility and accountability in algorithm-driven decisions. Industries such as finance and retail have faced setbacks due to opaque models, but transparent audit trails and version management now empower organizations. This shift has led to a reduction of reconciliation errors by approximately 20%—addressing the frequent inquiry: What elevates decision accuracy?
"Clear model audit trails are no longer optional; they are essential for sustaining trust in automated processes."
Explore model traceability metrics
- Model lineage
- Tracks the evolution and history of data models through every stage of development.
- Version management
- Ensures that every alteration in the model is recorded and reproducible for audit and quality control.
Integrating Advanced Technologies
Pioneers like IBM and leading Master Data Management (MDM) vendors such as Stibo Systems have demonstrated how blockchain strengthens trust in interconnected systems and enhances data traceability. Implementing AI-driven MDM practices has increased operational efficiencies by up to 25%—concisely clarifying how enhanced security and transparency drive better business outcomes.
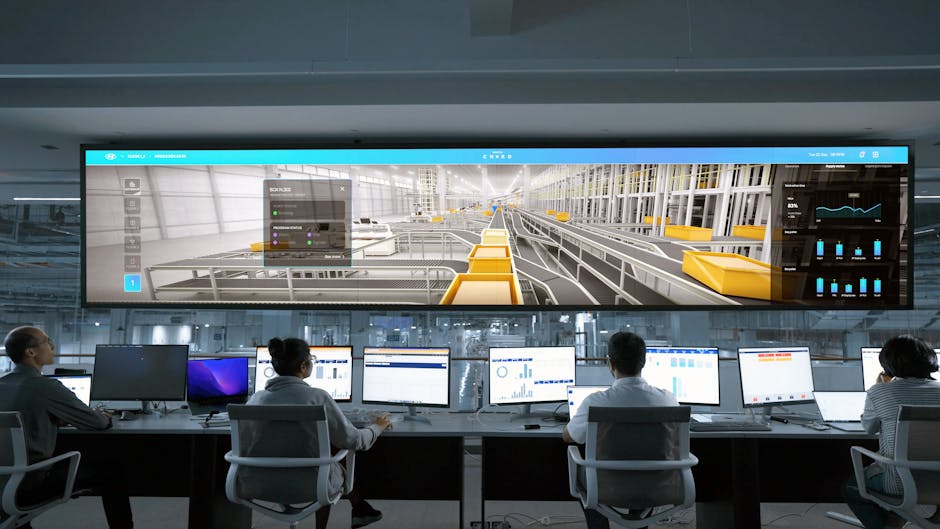
Understand blockchain's role in operational transparency
Blockchain isn’t just a buzzword. Its implementation in inventory and vendor management systems offers a robust solution to data tampering while providing clear, auditable trails that underpin trust and operational reliability.
Overcoming Embedding Challenges in Analytical Models
Integrating embedding systems in complex analytical models presents transparency hurdles. Historical experiences with model drift and opaque decision-making have caused disruptions. Modern solutions such as MLflow offer reproducibility and prompt error detection—resulting in a renewed focus on predictive analytics. These improvements help answer the question: How do we overcome the pitfalls of complex machine learning integrations?
Examine embedding system case details
Through improved tracking and iterative diagnostics, companies have successfully integrated embedding systems into their analytical frameworks, thereby enhancing model fidelity and overall operational stability.
Forecasting Models and Experiment Tracking in Action
Forecasting models are critical in optimizing inventory management, demand planning, and risk assessment. Forward-thinking companies now standardize experiment tracking to continually refine their models, which has led to a 15% boost in predictive accuracy. This practice is vital for reducing risks and ensuring market adaptability.
See detailed experiment tracking strategies
By utilizing real-time tracking and iterative model adjustments, businesses maintain precision in forecasting, promptly addressing discrepancies and further solidifying their market competitiveness.
Industry Case Studies: Learning from the Front Lines
Industry leaders and prominent retailers have confronted onboarding and traceability challenges head-on. Implementations combining blockchain with refined lifecycle traceability—from vendor to consumer delivery—demonstrate clear operational improvements. These case studies provide verifiable performance benchmarks and highlight how strategic foresight mitigates compliance risks while enhancing customer satisfaction.
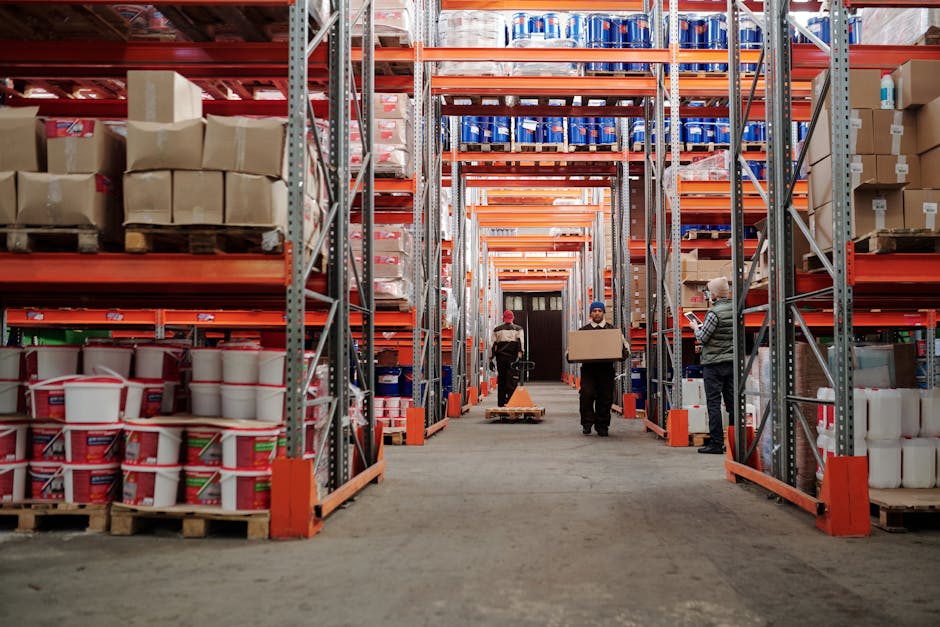
Actionable Strategies for Immediate Operational Improvements
Drawing on empirical data and best practices, organizations are encouraged to audit current onboarding protocols, integrate blockchain for improved traceability, and adopt cutting-edge MDM practices. Utilizing AI-driven anomaly detection and experiment tracking can help spot and fix model deficiencies before they escalate, thereby reinforcing market standing.
Team | Traditional Onboarding (days) | Enhanced Onboarding (days) | Efficiency Gain (%) |
---|---|---|---|
Retail Operations | 20 | 14 | 30 |
Logistics | 18 | 12 | 33 |
Customer Service | 15 | 10 | 33 |
Tech Integration | 22 | 16 | 27 |
Consider this overview when planning transformational onboarding implementations. Keywords: risk scoring, region priority recommender, operationalizing embeddings, need traceable models, experiment tracking, forecasting models. |
These strategic insights form a traceable roadmap for embedding operational excellence across e-commerce ecosystems, ensuring a sustainable path toward a data-centric and efficient future.