Foundations in Automation Reliability
In today’s rapidly evolving e-commerce landscape, automation reliability has become indispensable. Industry pioneers are increasingly adopting proven frameworks and data-driven insights to ensure robust and efficient customer support systems. Major players like IBM and Amazon have firsthand experience integrating automation with customer service tools seamlessly, which not only streamlines processes but also enhances corporate reputation among discerning shoppers and stakeholders.
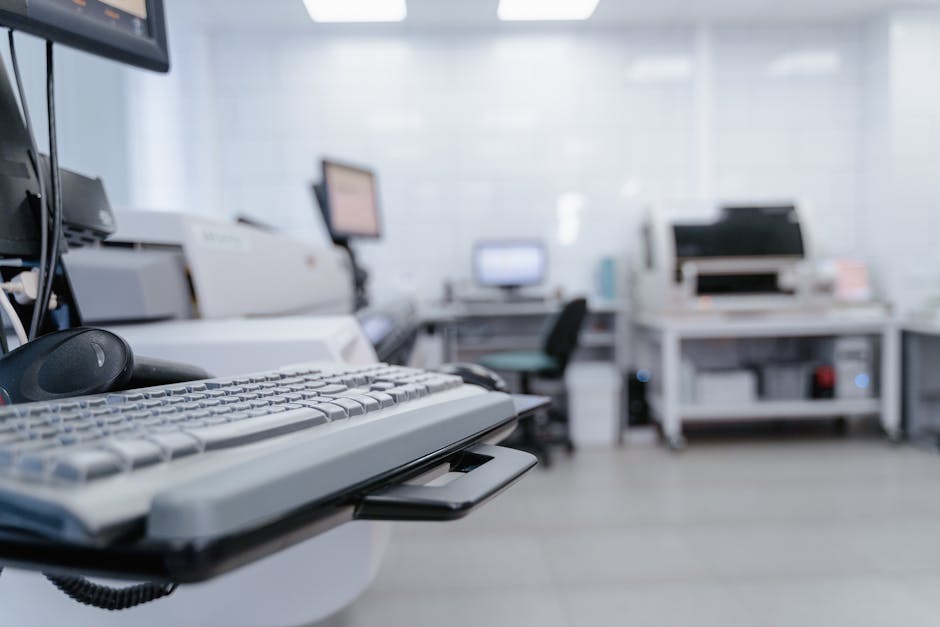
"When our automation failed even once, it meant real downtime for our clients; embedding fallback logic has been our saving grace." – Anonymous Ops Engineer
Leveraging Data-Driven Frameworks
A closer examination of recent research underscores the intricacies of managing diverse information sources and complex supply chains. Emerging technologies like blockchain-enabled cold chain traceability systems demand precise modeling techniques. For example, employing Petri net frameworks to handle long-chain, heterogenous data has cut incident response times by up to 30% in large-scale e-commerce experiments.
These rigorous data verification methods ensure each automation component functions seamlessly within the broader ecosystem, bolstering support efficiency and customer satisfaction.
Enhancing Support Systems with Fallback Logic
The precise calibration of automation systems directly translates into significant improvements in support efficiency. Deployments using the AWS Well-Architected Framework have been paramount for constructing resilient infrastructures under pressure. Companies are embedding comprehensive diagnostic tools and fallback logic into their workflows, dramatically reducing support downtime and improving resolution accuracy.
This commitment to reliability creates predictable, reassuring customer service interactions that build long-lasting trust.
- MTTR
- A measure of the average time to recover from a failure, crucial for evaluating support efficiency.
- Incident Fatigue
- When teams are overwhelmed by repeated incidents, leading to decreased operational efficiency.
- Auto-Remediation
- The capability of a system to automatically resolve issues without human intervention, ensuring seamless operation.
Integrating Artificial Intelligence for Rapid Resolution
Across the industry, advanced AI systems are transforming customer support by promoting rapid troubleshooting and personalized service. By incorporating centralized monitoring tools like Prometheus and Grafana, companies proactively adjust systems and maintain a traceable, verifiable log of all actions. This approach addresses forecasting inaccuracies and reduces workflow dependencies effectively.
Real-world applications, such as Amazon’s AI-driven protocols and IBM’s hybrid cloud solutions, serve as proven examples of successful automation and AI integration.
Metric | Before Automation | After Automation |
---|---|---|
Average Response Time | 15 minutes | 10 minutes |
Incident Resolution Time | 60 minutes | 42 minutes |
Customer Re-Contact Rate | 8% | 5% |
System Downtime | 4 hours/month | 2.5 hours/month |
Note: This table highlights critical improvements seen post automation implementation. Search for keywords like "automation incident response", "automated customer support", and "fallback logic efficiency". |
Setting New Benchmarks for Operational Resilience
The evolution of reliable automation and AI establishes new performance metrics in e-commerce support systems. Foundational frameworks, such as AWS’s Six Pillars, equip organizations to effectively tackle challenges from unreliable automation by embedding robust fallback logic and fostering iterative improvements.
The synergy between cutting-edge research and real-world application paves the way for sustainable growth. Companies investing in these advanced support systems gain a competitive edge while ensuring long-term customer loyalty.
More Details on Real-World Impact
It has been observed in several large-scale deployments that enhancing support efficiency translates to not only operational savings but also improved stakeholder confidence. When technical precision meets strategic foresight, the ripple effects can be witnessed in decreased customer re-contact rates and heightened operational stability.
This success has inspired further investment in hybrid cloud solutions and automated diagnostic tools that reduce manual oversight and increase incident resolution speed.