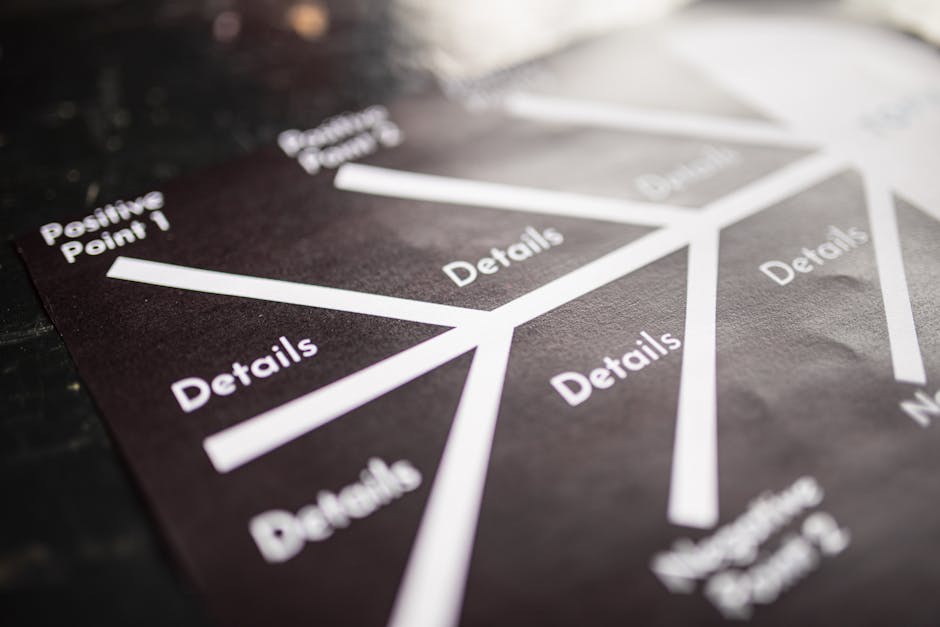
Overview and Context
In today’s fintech sector, internal tool owners manage sprawling data sources alongside persistent data quality challenges. Organizations are pressed to make highly technical decisions based on efficient workflow prioritization. With insights drawn from recent research in biomedical sequencing and healthcare workflow automation, this piece lays a foundation for understanding how embedding analysis can redefine decision-making and operational urgency.
Real-World Implications of Enterprise Patterns
Industry leaders such as IBM and Meta have set the pace in data-driven process improvements. In vibrant tech hubs like Austin, fintech teams integrate disparate data streams and leverage innovations like IBM’s Business Automation Workflow Assignments. These initiatives highlight how even mundane tasks—manual form cleaning and data reconciliation—can bolster operational efficiency. Crucially, many professionals review tool outputs for accuracy and detect hidden process cues in contexts where significant work happens outside formal systems.
Embedding Analysis and Enterprise RAG Patterns
Embedding space analysis combined with regression models is reshaping enterprise strategies. Specifically, how embedding analysis can predict operational bottlenecks by using RAG (Red, Amber, Green) signals to assess risk. By automating pipelines—a method once pioneered in biomedical sequencing research—fintech operations can better manage known data quality issues. For example, an Austin-based fintech operation detected an impending bottleneck using this analytical approach, turning abstract research insights into tangible workflow improvements.
Workflow Prioritization: From Research to Action
Moving from theory to practice, effective workflow prioritization leverages real-time data insights to trigger immediate actions. Lessons from health information technology research remind us that inefficiencies can lead to cascading failures. In fintech, where internal tools export data for team use and reconcile multi-source inputs, displaying real-time operational signals during cross-functional review meetings is a game changer.
Actionable strategies, such as implementing PowerAutomate, are emerging as effective means to replace broken processes. These systems replace legacy methods with responsive solutions, reducing the burden of manual data exports and reconciliation tasks.
Comparing Embedded and External Analysis Approaches
Criteria | Embedded Analysis | External Analysis |
---|---|---|
Speed | High; processes data in real-time | Slower; involves batch processing |
Accuracy | Enhanced via direct integration | Variable; dependent on manual reconciliation |
Cost | Efficient if leveraging automated pipelines | Higher due to manual efforts |
Adoption | Streamlined by ease-of-use in modern systems | Challenging amid legacy system constraints |
Consider future trends in real-time data processing and automation. Keywords: embedded systems, operational efficiency, fintech workflow. |
Strategic Takeaways and Future Directions
Fintech professionals must act swiftly to adapt to modern enterprise patterns. The integration of embedded analysis into everyday operational frameworks enables them to quickly resolve data quality issues and identify hidden correlations across multi-source financial data.
By embracing these data-driven strategies, organizations can transition from reactive measures to proactive, resilient operations. The adoption of systems like PowerAutomate is not just about upgrading technology—it’s about creating a sustainable process where early-warning systems guide real-time decisions.
Ultimately, the balance of strategic transformation and practical implementation offers a clear roadmap. With continuous advancements in technology and analytics, fintech operations can overcome outdated loops, drive innovation, and secure a competitive edge.