Pitfall #1: Introduction
In today’s AI-driven fintech world, maintaining robust compliance is non-negotiable. Industry pioneers have experienced how gaps in documented logic and unmanaged tool outputs can lead to significant regulatory fallout. This emphasizes that cutting-edge technology must run on solid foundations to prevent costly oversights.
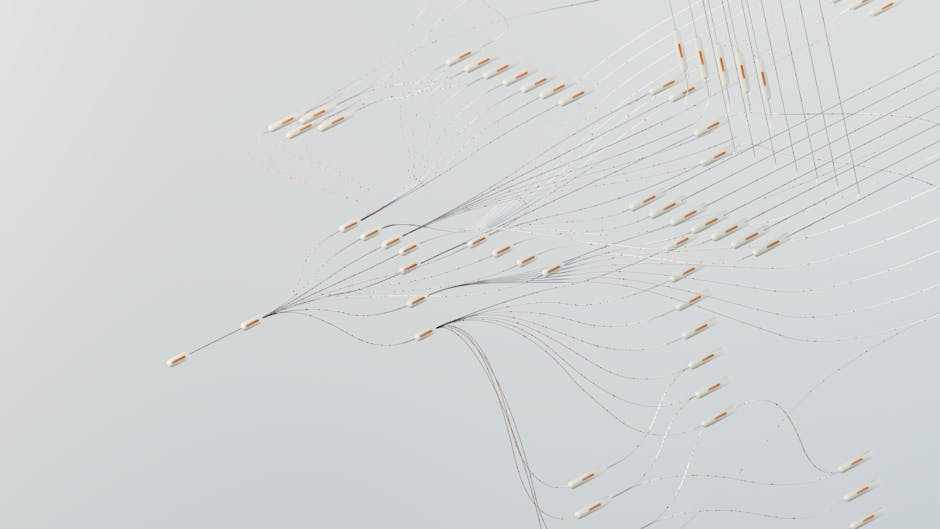
By integrating human judgment with advanced AI, teams can maintain oversight even as automated reporting tools and real-time dashboards create a complex data landscape.
Pitfall #2: Expert Insights
Influential voices from companies like LogicGate Risk Cloud and Unit21 emphasize that transparency paired with continuous monitoring creates the backbone for a reliable system. Platforms such as Flagright supply rigorous real-time observability, revolutionizing how fintech firms manage accountability and risk.
This collective expertise draws on empirical results and practical lessons. As an anecdote, I helped a growth team use Named Entity Recognition with spaCy to extract marketing insights from unstructured call notes, demonstrating how nuanced data can reshape oversight structures.
Pitfall #3: Compliance Failures
Historical lapses—including AI misjudgments that nearly cost firms dearly on Wall Street—warn of the dangers of insufficient oversight. Unchecked drift in key performance indicators has forced financial leaders to adopt comprehensive, automated guides that flag compliance issues before they escalate.
Emphasize real-time data drift detection at the pipeline level as an effective early warning method to avoid cascading compliance errors. This approach not only saves costs but also facilitates continuous learning and adjustment in dynamic environments.
Pitfall #4: Actionable Steps
Successful fintech operations integrate both technology and human oversight. Tools such as Unit21’s API dashboard detect anomalies early by merging automated alerts with manual oversight, providing a critical backup against potential blind spots.
Implement comprehensive third-party risk management methods, rigorous testing protocols, and real-time drift reviews. These measures ensure that both the automated process and the human decision-making aspects remain on track.
Furthermore, teams should maintain documented governance frameworks and version-controlled logs to solidify operational integrity.
- Model Drift
- Refers to the gradual change in model performance due to evolving data patterns that can impact decision-making if left unchecked.
- Overfitting to Regulation
- Occurs when a system is excessively tailored to meet current regulatory standards, potentially hindering its ability to adapt to future changes.
- Explainability Debt
- The cost incurred when AI models become opaque over time, making it challenging to understand or rectify decision logic during compliance audits.
Pitfall #5: Conclusion
Fintech entities can mitigate errors and build trust by triangulating powerful AI capabilities with disciplined compliance practices. Real-world lessons, expert insights, and clear processes create a blueprint for safe, reliable operations.
Such a blueprint relies heavily on documented governance frameworks and version-controlled logs to ensure alignment with industry best practices. This balanced approach addresses the rapid evolution typical in the fintech industry while safeguarding against both internal and external risks.
By merging automation with manual oversight, organizations not only enhance regulatory compliance but also secure competitive advantages through adaptive risk management strategies.
Additional Considerations and Deep Dive
Further exploration into the integration of AI in compliance reveals that continuous monitoring and audit trails are essential. These practices help in predicting potential issues even when data quality challenges occur, ensuring that proactive measures are always in place. This deeper understanding is critical for long-term stability in rapidly evolving fintech environments.