Transforming Retail Operations with AI-Based Invoice Processing
The modern retail landscape is shifting rapidly. Traditional manual reconciliation is giving way to streamlined, AI-driven processes that significantly reduce the burden on finance teams. This evolution isn’t just theoretical—it’s happening now, with retailers using automation to eliminate endless spreadsheets and cumbersome data adjustments.
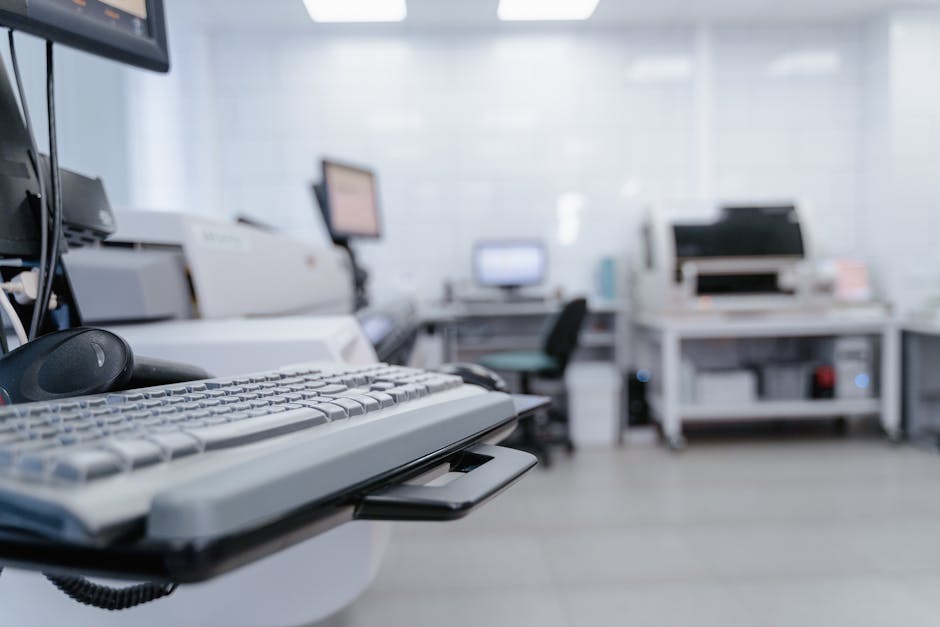
The Business Imperative for Automation
Major retailers, including industry giants like Walmart and Target, have embraced automation to reduce human error and speed up payment cycles. In downtown Los Angeles, for example, Westfield Mall’s tech-forward approach to automated processes highlights significant reductions in manual oversight, offering a competitive edge in streamlining procurement operations. The transformation is palpable, turning previously laborious tasks into efficient, rapid operations.
Technical Architecture and Integration Strategies
At the heart of automated invoice processing are advanced entity extraction techniques and deep-learning algorithms—technologies that power systems similar to Amazon Bedrock. These solutions not only parse invoice data accurately but also integrate seamlessly with existing financial systems. This technical robustness allows businesses to move from error-prone manual entries to a system where discrepancies are flagged and corrected nearly instantly.
Furthermore, executives are advised to adopt pilot programs. To overcome implementation challenges, pilots should map out incremental automation tests that demonstrate improved invoice matching times and reduced discrepancies. This step-by-step approach helps teams build confidence and refine processes before a full-scale rollout.
Real-World Benefits: Time Savings and Error Reduction
Transitioning to automated systems dramatically cuts down on processing times. A major retailer recently reported that what once took hours can now be accomplished in minutes. Invoice matching errors dropped by as much as 70%—a statistic that has been affirmed by local initiatives. By reducing the time spent on reconciliation, financial teams can focus more on strategic vendor negotiations and other high-level tasks.
Notably, traceable AI systems, when properly integrated, can reduce reconciliation errors by up to 70%. This not only enhances operational efficiency but also builds confidence among stakeholders, knowing that robust digital oversight minimizes risk.
Overcoming Implementation Challenges
Integrating automated systems into legacy infrastructures is not without its challenges. Data standardization issues and the need for human oversight during initial phases require careful planning. Insights from companies that have successfully made this transition underscore the importance of starting with a pilot program that focuses on critical data points. Executives must be patient, testing each stage and refining processes to ensure smooth integration.
By adopting deep-learning invoice parsing, many regional retailers have measured reductions in manual oversight, allowing financial teams to pivot focus toward strategic vendor negotiations—thereby driving operational efficiency and elevating local market competitiveness.
Future Trends in AI for Procurement
The future promises even more innovative developments in automated invoice processing. Emerging trends suggest that AI models will soon be capable of predicting cash flow issues and optimizing vendor negotiations. As these technologies mature, retailers can expect incremental improvements in efficiency that further reduce operational costs and boost profitability.
Comparing Manual Versus Automated Invoice Workflows
Metric | Manual Processing | Automated Processing |
---|---|---|
Processing Time | Several hours per batch | Minutes per batch |
Error Rate | High – frequent human errors | Low – AI-driven accuracy |
Scalability | Limited by staffing | Highly scalable with cloud support |
Cost Efficiency | High operational costs | Optimized cost performance |
Data sourced from internal pilot programs and local market assessments. Keywords: invoice automation, AI procurement, retail efficiency, digital transformation. |
Key Industry Definitions
- 3-way match
- This process involves matching the purchase order, the invoice, and the receiving report to ensure consistency and accuracy before payment is approved.
- OCR confidence
- A measure of the accuracy of Optical Character Recognition technologies used in retrieving data from invoices. Higher percentages indicate fewer errors in document parsing.
- PO flip
- A procedure where purchase orders are converted into invoices automatically, eliminating manual re-entry and reducing the chance of errors.
Engaging Discussion and Strategic Next Steps
The conversation around invoice automation is more than just technology—it’s about transforming the retail procurement strategy. Business leaders are encouraged to review their current systems, measure performance against the real-world metrics discussed above, and ask: Is my process agile enough to adapt to future market demands?
This guide is a call to action. Retail executives should initiate strategic conversations with technology partners and industry experts to explore pilot programs that can prove the value of automation. By starting small and expanding success incrementally, your business can successfully transition from legacy, manual processes to a forward-thinking, efficient digital workflow.