AI Model Breakdown
Decoding the Highs and Lows of AI Decision Processes
- Transparent AI Systems
- AI models that allow stakeholders to understand the decision-making logic, ensuring greater trust and reliability.
- Black-Box AI
- Models that produce outputs without revealing the underlying processes, often leading to trust issues.
- Explainable AI (XAI)
- A subset of AI that focuses on making AI decisions interpretable by humans.
- NLP
- Natural Language Processing, a branch of AI focused on interactions between computers and humans using natural language.
Clarity through Transparency
Empirical evidence from landmarks of scholarly might, like ScienceDirect and PubMed, decrypts the ethical pitfalls and uninvited biases of AI models. There's a consensus that unveiling hidden model processes is essential for building stakeholder trust. In critical industries—where even slight errors can have financial or safety repercussions—unfurling the opaque layers of AI methodology is not just advisable, it’s imperative.
When AI systems emphasize transparency, they lead to resounding successes. For instance, leaders in the healthcare sector have paved the way for models that decode risks and trends, aligning predictions neatly with regulatory standards. This transparency serves as a blueprint for other industries to follow.
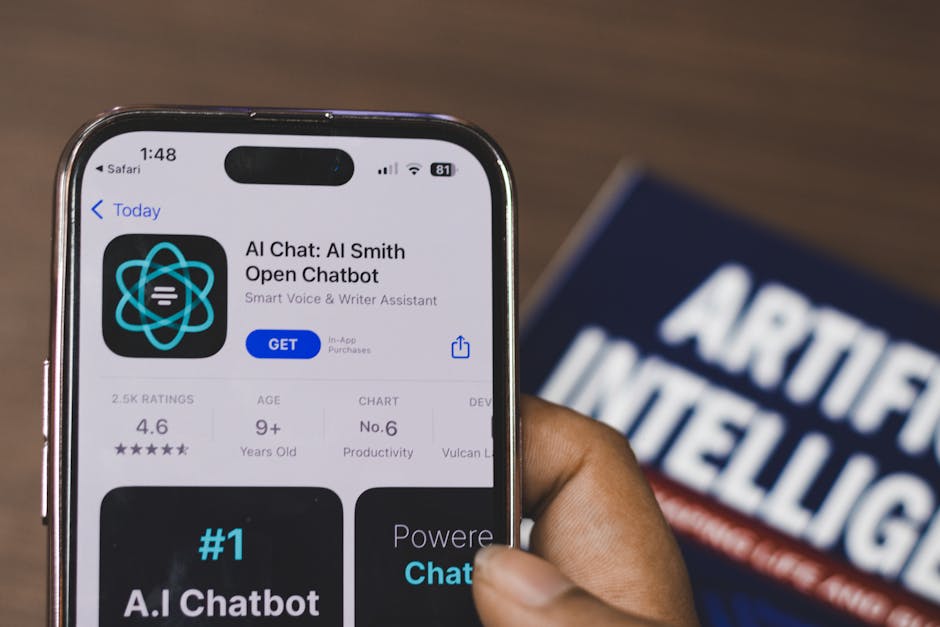
Failure: Boardrooms on Alert
Despite its promise, the dark side of AI remains a sobering lesson. Anecdotes from the sector recall AI systems that deviated drastically from projections, initiating board-level emergencies. These incidents underscore the perils of black-box approaches where unclear logic breeds distrust and operational vulnerabilities.
Analyses reveal that opaque methodologies in Natural Language Processing—consider, for example, the debate between LoRA techniques versus full fine-tuning—can lead to significant misalignments in expected outcomes. The experiences of industry giants like Anthropic highlight the urgent need for explainable AI to bolster confidence and adaptability.
Navigating Strategies: Excelling at the AI Game
Bridging the gap between technical precision and business strategy, modern AI deployments are increasingly integrating RegTech solutions and iterative recalibration practices. By coupling real-time error feedback loops with continuous model validation, organizations maintain operational agility and regulatory compliance.
This approach not only refines AI model outputs but also helps in eliciting an environment where decision outcomes are aligned with business goals. In practice, this means recalibrating your model pipelines regularly—a strategy reminiscent of real-time Slack messages being pipelined into OpenAI calls, validated as JSON outputs, and auto-routed directly to Salesforce.
A Simplified Comparison of Decision Outcomes
Model | Input Type | Outcome | Confidence | Error Type |
---|---|---|---|---|
Transparent AI | Structured & Real-Time Data | Predictable Outcomes | High | None |
Black-Box AI | Aggregated Data Streams | Unexpected Deviations | Low | Algorithmic Drift |
Explainable AI | Validated Inputs | Regulatory Alignment | High | Transient Errors |
NLP Systems | Natural Language Data | Contextual Relevance | Medium | Bias-Induced Errors |
Consider continuous recalibration, iterative model validation, and transparent logic as vital components to prevent model failures. Keywords: transparent AI, black-box limitations, explainable AI, NLP challenges. |
Conclusion and the Path Ahead
Our exploration into AI decision-making highlights the dual nature of these systems: the brilliance of transparency versus the pitfalls of obscurity. Lessons learned from both successes and failures drive home the essence of aligning technical operations with strategic business imperatives.
It is crucial that leaders continue to build bridges between model logic and real-world applications. By striving for iterative improvement and maintaining open channels of decision transparency, organizations not only safeguard themselves against board-level fire drills but also empower themselves to harness AI's full potential.