EMBEDDING OPERATIONALIZATION: UNLOCKING INSIGHTS
Embedding operationalization offers an advanced method for churn detection by transforming complex datasets into actionable insights. This technology, similar in spirit to SnapLogic's handling of vector embeddings, translates high-dimensional manufacturing data into a seamless vector space. The result is a clear pattern recognition process, making it easier to interpret key data trends.
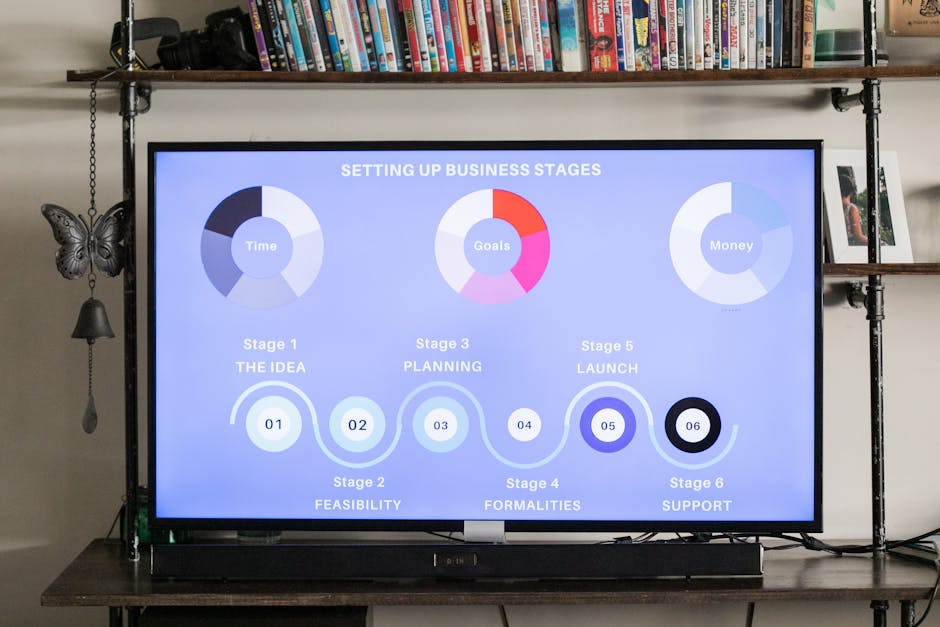
FROM DATA TRANSFORMATION TO STRATEGIC VALUE
Top industry leaders like Nvidia have demonstrated the benefits of converting diverse datasets into embeddings. This approach uncovers hidden relationships in data while refining churn detection models. By converting raw data into structured embeddings, organizations overcome recurrent data reconciliation hurdles—transforming complexity into strategic, actionable insights.
I helped a growth team use Named Entity Recognition with spaCy to extract marketing insights from unstructured call notes, proving that even the most chaotic data can be refined into a powerful strategic asset.
INTEGRATING EMBEDDINGS INTO CHURN MODELS
Embracing current AI trends, embedding operationalization shifts churn detection from conventional analytics to proactive strategic anticipation. Inspired by Explo’s embedded analytics and insights from experts like Matt Dancho, businesses are now fine-tuning machine learning models to precisely flag both compliance and churn risks.
This integration enables organizations to implement segmentation workflows, ensuring that each data point enhances predictive capabilities in real-time.
AVOIDING COMMON PITFALLS
Many teams encounter challenges when adopting AI, sometimes misunderstanding the true potential of embedding applications. A misalignment in data sources or operational configurations can lead to inefficiencies. However, by embracing focused segmentation workflows and strategic methodologies, teams can anticipate and mitigate risks like operational misconfiguration.
It is crucial to maintain consistent data formats throughout the analysis and visualization process to avoid pitfalls and maintain the integrity of the predicted outcomes.
TURNING INSIGHT INTO ACTION
A strong foundation in data transformation and embedding integration sets organizations on a clear course towards actionable insights. This method not only optimizes quarterly operations reviews but also sharpens customer retention strategies and sets new benchmarks for operational AI efficiency.
Implementing validation steps—such as change point detection thresholds—ensures the robustness of the embeddings and minimizes the risk of operational disruptions across diverse environments.
PRE-EMBEDDING VS POST-EMBEDDING DETECTION TIMELINES
Phase | Detection Approach | Time to Action | Operational Impact |
---|---|---|---|
Initial Analysis | Pre-Embedding Analytics | Longer | Limited insight; delayed response |
Feature Integration | Transition Phase | Moderate | Improved insight; evolving impact |
Operational Deployment | Post-Embedding Detection | Shortened | Rapid action; enhanced efficiency |
Optimization & Review | Continuous Embedding Updates | Minimal | Dynamic adjustments; sustained impact |
Considerations: Ensure data consistency, utilize segmentation workflows, and monitor change point detection thresholds for a robust operational model. Keywords for further exploration: churn detection, operational latency, embedding drift. |
KEY CONCEPT DEFINITIONS
- embedding drift
- A phenomenon where the statistical properties of embeddings change over time, potentially impacting model accuracy.
- churn proxy signals
- Indicators derived from embedding data that predict customer discontinuation or operational inefficiencies.
- operational latency
- The delay in operational processes that can be minimized via rapid data embedding, leading to faster insights and responses.
By anchoring strategic decisions in both advanced AI methodologies and proven industry practices, organizations can maintain a competitive edge—transforming raw data into a catalyst for change in the manufacturing landscape.